Online Missing Value Imputation for High-Dimensional Mixed-Type Data Via Generalized Factor Models.
Computational statistics & data analysis(2023)
摘要
The complete-observation requirement of most machine learning methods necessitates new statistical methods to handle datasets messy with missing values. This is especially urgent for streaming data that are generated at high speed and with a lack of quality control. Missing data imputation becomes an inevitable preprocessing step before subsequent analysis. A practical and meaningful online imputation algorithm should be not only scalable to large-scale datasets but also able to manage high-dimensional mixed-type data containing binary, count and continuous variables. To fill this gap, a novel online imputation algorithm, called OMIG, is proposed for streaming data under the framework of generalized factor models. To obtain deeper insight, OMIG is theoretically and empirically compared to its other two versions, the oracle version and the offline version. Theoretical and numerical findings show that (a) the imputed data obtained by OMIG are not equivalent to but instead at a slower rate than those obtained by its oracle version in terms of imputation accuracy; (b) OMIG outperforms its offline version in imputation accuracy; and (c) OMIG is equivalent to its oracle version in estimation accuracy for the factor loading, which largely facilitates interpretation and follow-up analysis. Extensive numerical experiments and two real datasets are used to demonstrate the performance of the proposed method.
更多查看译文
关键词
Generalized factor model,High-dimensional mixed-type data,Missing at random,Streaming data imputation
AI 理解论文
溯源树
样例
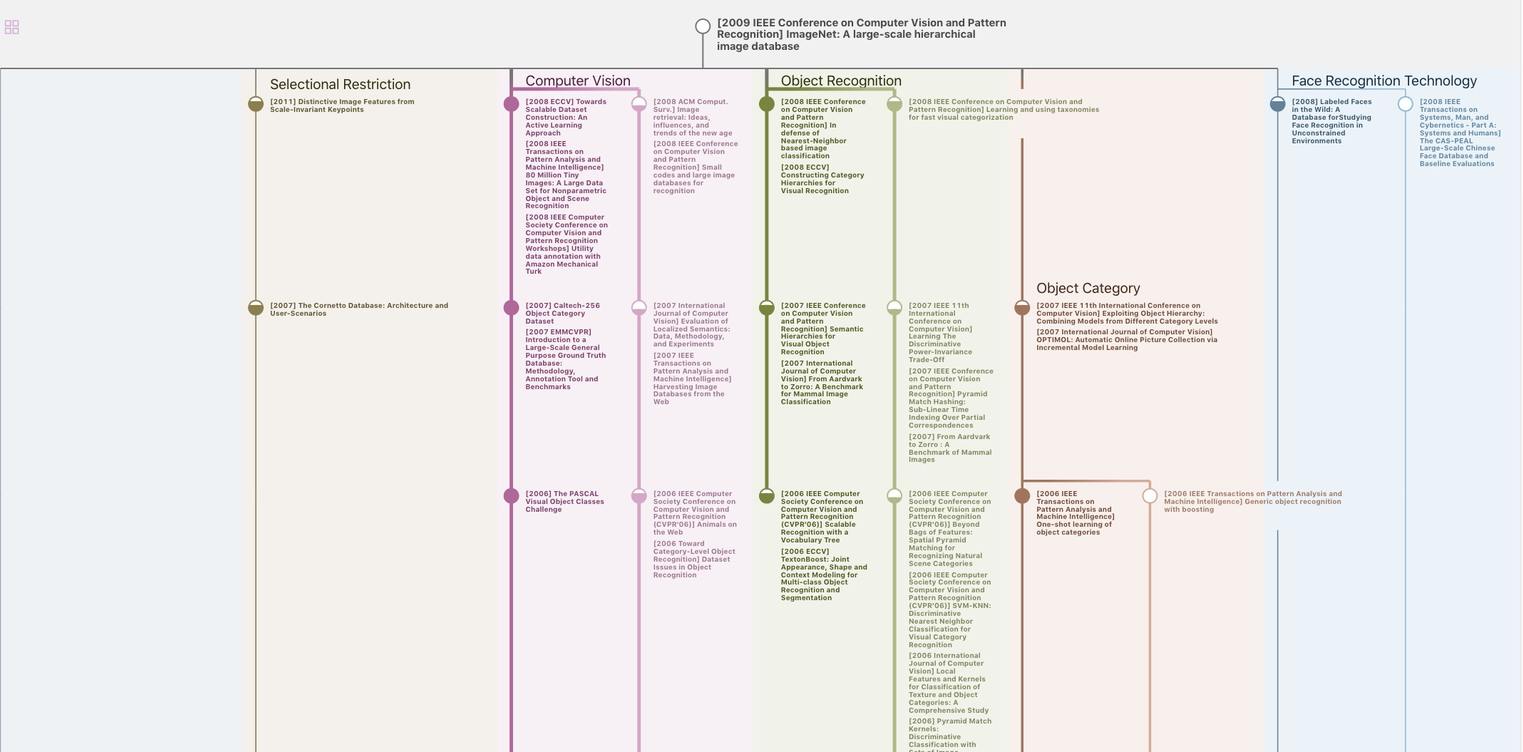
生成溯源树,研究论文发展脉络
Chat Paper
正在生成论文摘要