DBENet: Dual-Branch Ensemble Network for Sea-Land Segmentation of Remote-Sensing Images
IEEE Trans. Instrum. Meas.(2023)
摘要
Sea-land segmentation of optical remote-sensing images holds great importance for military and civilian applications, such as coastal monitoring, target detection, and resource management. Although convolutional neural networks (CNNs) have achieved significant improvements in semantic segmentation, the challenges of efficient feature extraction, representation, fusion, and information transmission remain unsolved, which especially impacts the segmentation effectiveness of existing CNN-based models in extracting irregular and refined sea-land boundaries. In this article, a novel dual-branch ensemble network (DBENet) is proposed for pixel-level sea-land segmentation. The salient properties of the DBENet are: 1) a novel dual-branch network architecture is developed to achieve sufficient feature extraction and representation and 2) an efficient ensemble attention learning strategy suitable for the DBENet is designed to strengthen the correlation between dual branches to further facilitate feature fusion and information transmission. The comparative study with state-of-the-art methods reveals the superior performance of our approach, and the ablation study demonstrates the effectiveness of each component in the proposed network. The source code is available at https://github.com/RobertTang0/DBENet.
更多查看译文
关键词
Convolutional neural networks (CNNs),deep learning,remote-sensing image,sea-land segmentation,semantic segmentation
AI 理解论文
溯源树
样例
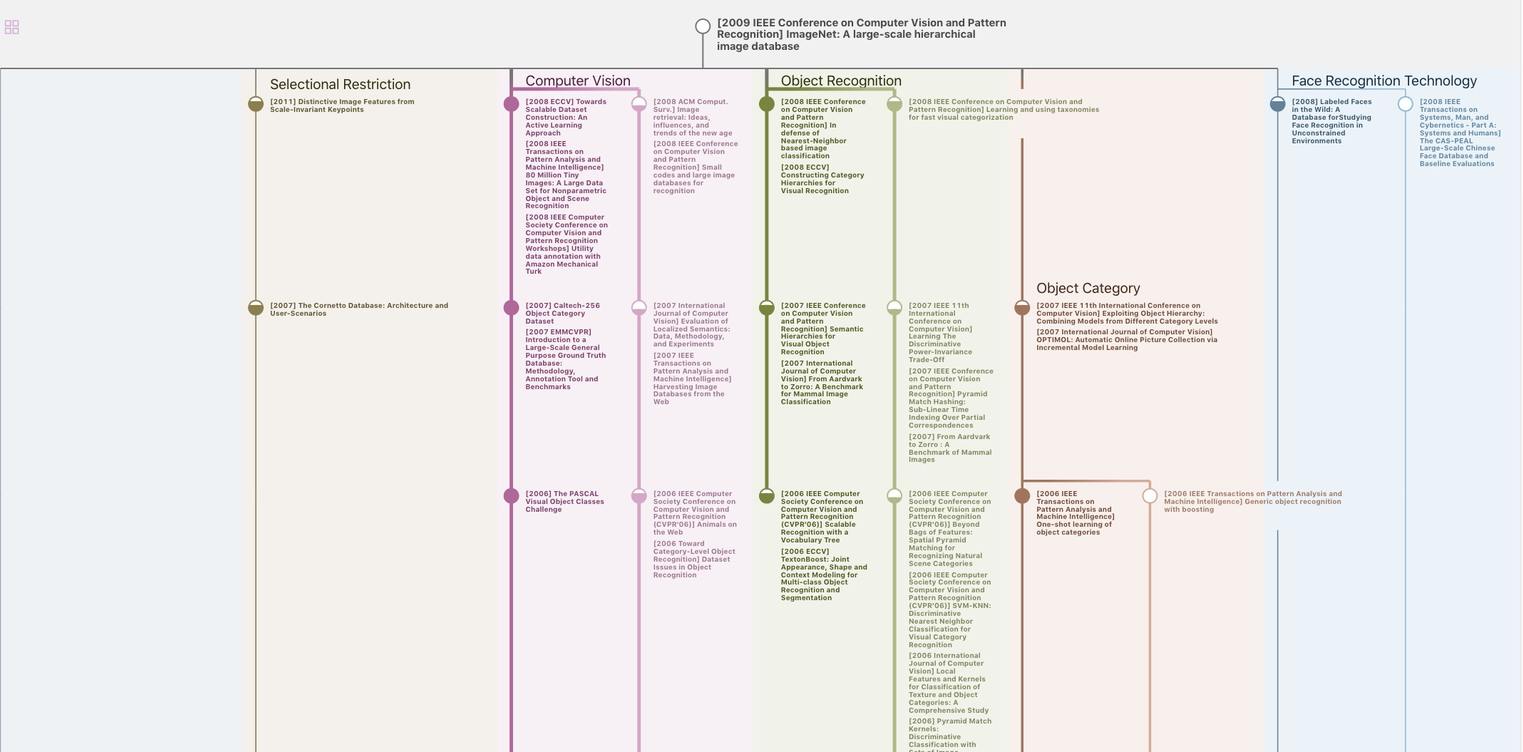
生成溯源树,研究论文发展脉络
Chat Paper
正在生成论文摘要