Simulation and Experimental Research of Thermal Power Plant Pipeline Displacement via Deep Learning Algorithms
IEEE TRANSACTIONS ON INSTRUMENTATION AND MEASUREMENT(2023)
摘要
The displacement of pipelines in thermal power plants is critical to ensure the safe operation of the thermal power unit. A 3-D online displacement monitoring network, the deep displacement network (DDN), has been designed to obtain real-time displacement data for the entire pipeline. The DDN utilizes a displacement network comprising a 3-D CNN-attention-gate recurrent unit (GRU)-3-D transposed CNN structure and a dataset established by CAESAR II to establish a displacement prediction model for four key piping systems. In addition, to address the limitations of traditional mechanical pointers, which can only measure 2-D displacement and cannot measure displacement online, a real-time stereo displacement measurement device (SDMD) has been developed based on the theory of stereoscopic vision and artificial neural networks (ANNs). The reliability of the DDN and SDMD has been validated through simulations, experiments, and field applications in power plants. Field application results have demonstrated that the absolute error of the SDMD is less than 2 mm compared with traditional mechanical pointers, and the relative error between the predicted value by DDN and the measured value of SDMD is less than 9%. Furthermore, the attention layer can improve efficiency in reducing the relative error, while the 3-D transposed CNN structure can minimize the maximum relative error. Finally, the DDN can solve the issue of requiring a large number of measurement points for the SDMD and significantly reduce the cost of measurement equipment.
更多查看译文
关键词
Attention structure,deep learning algorithms,displacement,stereo displacement measurement devices (SDMDs),thermal power plant pipeline
AI 理解论文
溯源树
样例
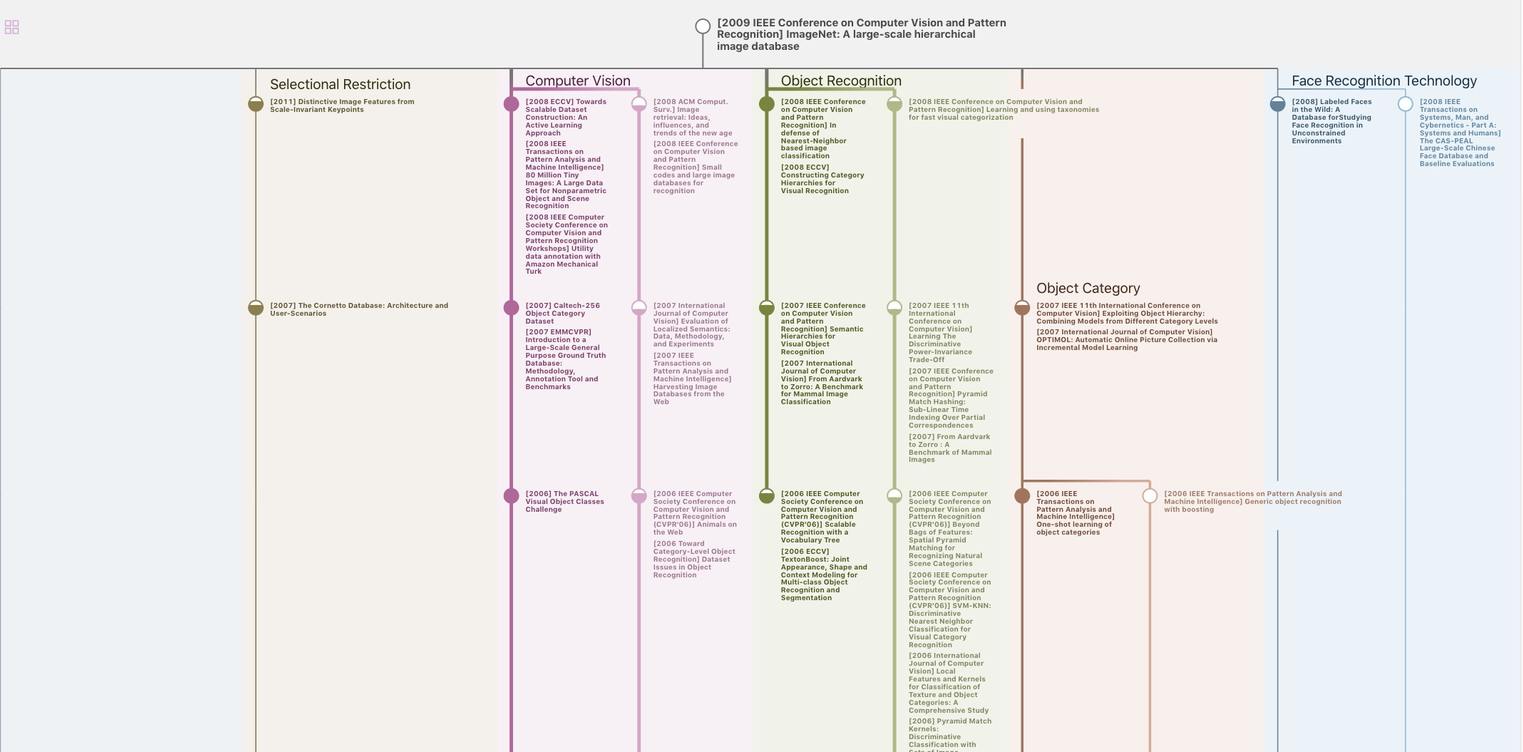
生成溯源树,研究论文发展脉络
Chat Paper
正在生成论文摘要