A Comparative Study for SDN Security Based on Machine Learning.
Int. J. Interact. Mob. Technol.(2023)
摘要
In the past decade, traditional networks have been utilized to transfer data between more than one node. The primary problem related to formal networks is their stable essence, which makes them incapable of meeting the requirements of nodes recently inserted into the network. Thus, formal networks are substituted by a Software Defined Network (SDN). The latter can be utilized to construct a structure for intensive data applications like big data. In this paper, a comparative investigation of Deep Neural Network (DNN) and Machine Learning (ML) techniques that uses various feature selection techniques is undertaken. The ML techniques employed in this approach are decision tree (DT), Naïve Bayes (NB), Support Vector Machine (SVM). The proposed approach is tested experimentally and evaluated using an available NSL–KDD dataset. This dataset includes 41 features and 148,517 samples. To evaluate the techniques, several estimation measurements are calculated. The results prove that DT is the most accurate and effective approach. Furthermore, the evaluation measurements indicate the efficacy of the presented approach compared to earlier studies.
更多查看译文
关键词
sdn security,machine learning
AI 理解论文
溯源树
样例
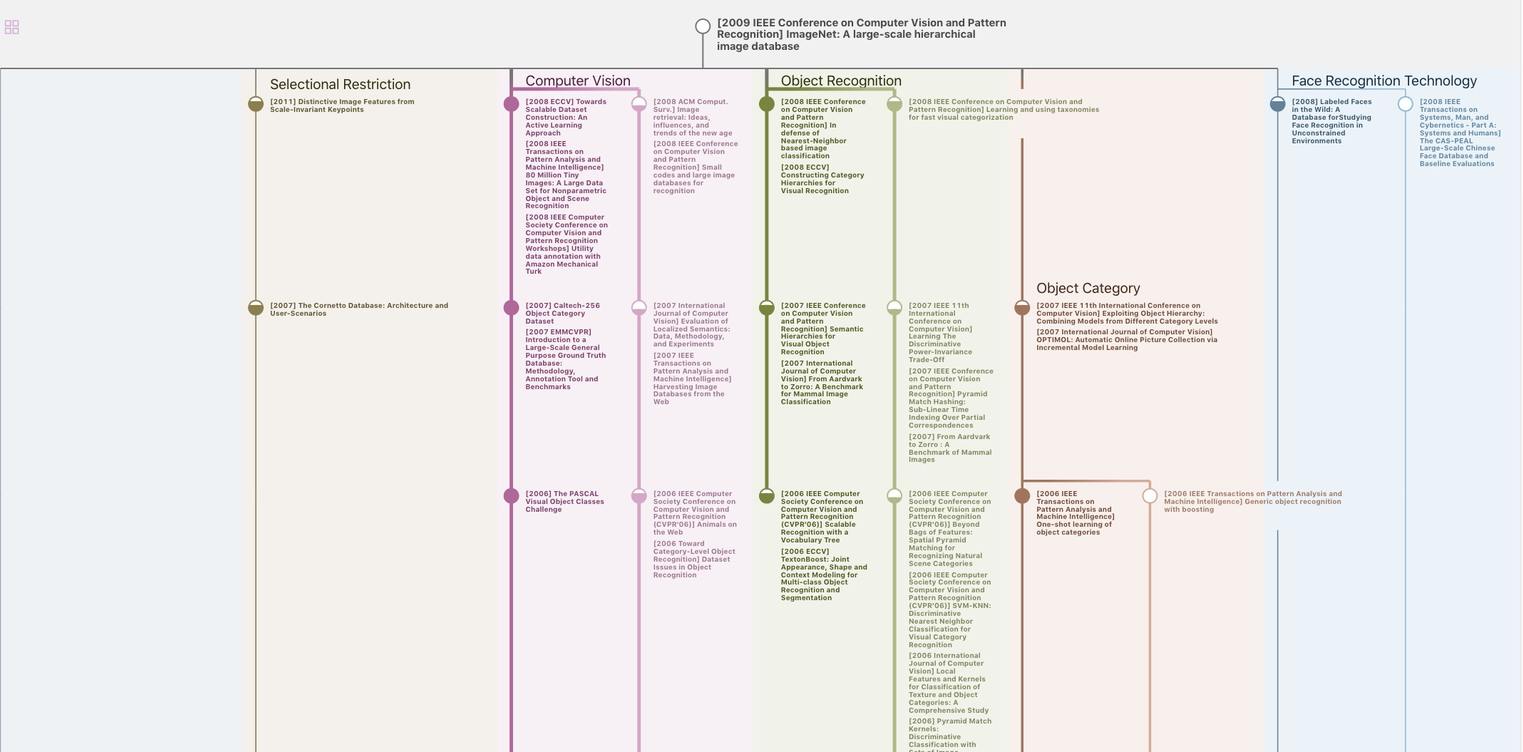
生成溯源树,研究论文发展脉络
Chat Paper
正在生成论文摘要