Regularized boosting with an increasing coefficient magnitude stop criterion as meta-learner in hyperparameter optimization stacking ensemble
Neurocomputing(2023)
摘要
Hyperparameter Optimization (HPO) aims to tune hyperparameters for a system in order to improve the predictive performance. Typically, only the hyperparameter configuration with the best performance is chosen after performing several trials. However, some works try to take advantage of the effort made when training all the models with every hyperparameter configuration trial and, instead of discarding all but one, they propose performing an ensemble of all the models. However, this ensemble consists of simply averaging the model predictions or weighting the models by a certain probability. Recently, some of the so-called Automated Machine Learning (AutoML) frameworks have included other more sophisticated ensemble strategies, such as the Caruana method or the stacking strategy. On the one hand, the Caruana method has been shown to perform well in HPO ensemble, since it is not affected by the issues caused by multicollinearity, which is prevalent in HPO. It just computes the average over a subset of predictions, previously chosen through a forward stepwise selection with replacement. But it does not benefit from the generalization power of a learning process. On the other hand, stacking approaches include a learning procedure since a meta-learner is required to perform the ensemble. Yet, one hardly finds advice about which meta-learner can be adequate. Besides, some possible meta-learners may suffer from problems caused by multicollinearity or need to be tuned in order to mitigate or reduce this obstacle. In an attempt to reduce this lack of advice, this paper exhaustively explores possible meta-learners for stacking ensemble in HPO, free of hyperparameter tuning and able to mitigate the problems derived from multicollinearity as well as taking advantage of the generalization power that a learning process may include in the ensemble. Particularly, the boosting strategy shows promise in this context as a stacking meta-learner, since it satisfies the required conditions. In addition, boosting is even able to completely remove the effects of multicollinearity. This paper provides advice on how to use boosting as a meta-learner in the stacking ensemble. In any case, its main contribution is to propose an implicit regularization in the classical boosting algorithm and a novel non-parametric stop criterion suitable only for boosting and specifically designed for the HPO context. The existing synergy between these two improvements performed over boosting exhibits competitive and promising predictive power performance as a stacking meta-learner in HPO compared to other existing meta-learners and ensemble approaches for HPO other than the stacking ensemble.
更多查看译文
关键词
Hyperparameter optimization,Stacking ensemble,Boosting
AI 理解论文
溯源树
样例
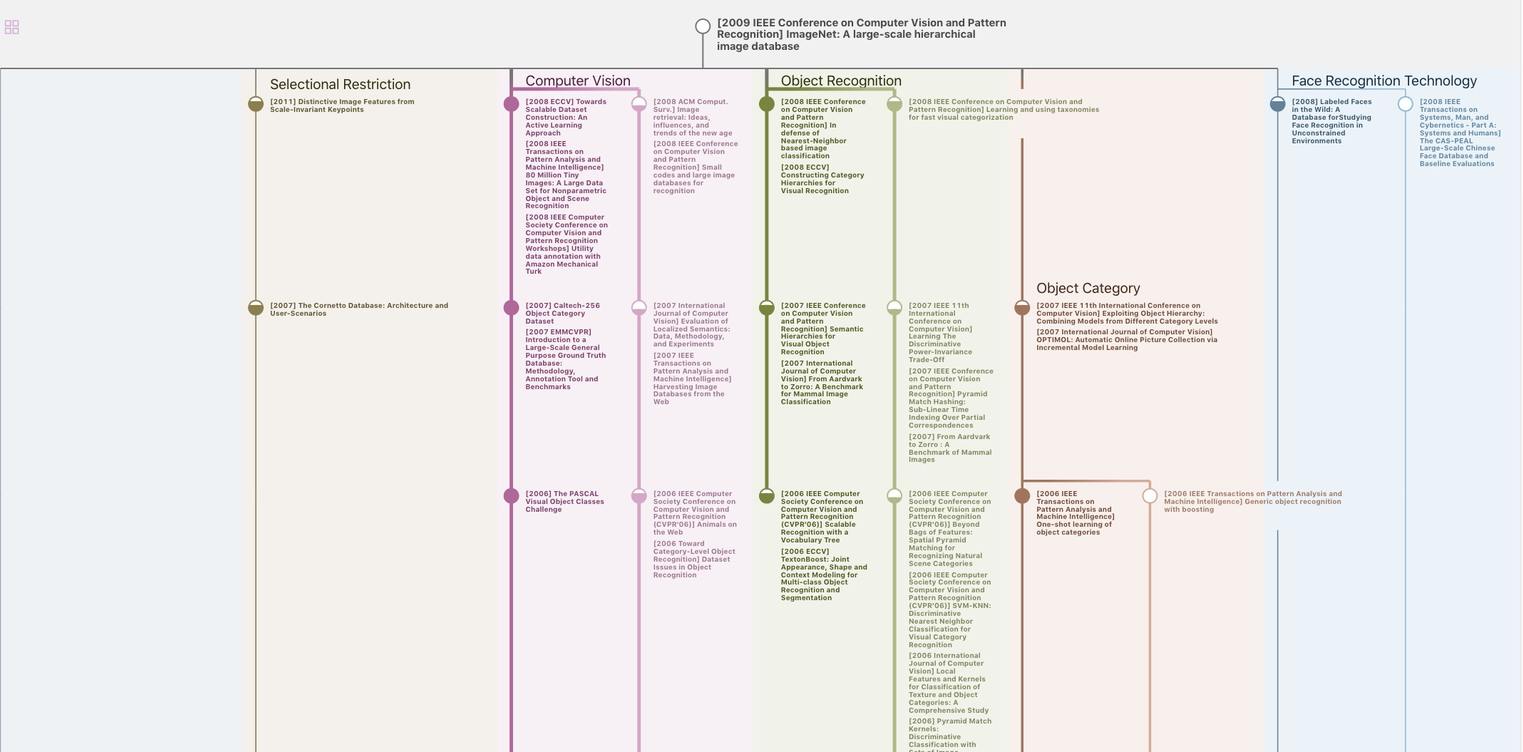
生成溯源树,研究论文发展脉络
Chat Paper
正在生成论文摘要