Exploring latent weight factors and global information for food-oriented cross-modal retrieval.
Connect. Sci.(2023)
摘要
Food-oriented cross-modal retrieval aims to retrieve relevant recipes given food images or vice versa. The modality semantic gap between recipes and food images (text and image modalities) is the main challenge. Though several studies are introduced to bridge this gap, they still suffer from two major limitations: 1) The simple embedding concatenation only can capture the simple interactions rather than complex interactions between different recipe components. 2) The image feature extraction based on convolutional neural networks only considers the local features and ignores the global features of an image, as well as the interactions between different extracted features. This paper proposes a novel method based on Latent Component Weight Factors and Global Information (LCWF-GI) to learn the robust recipe and image representations for food-oriented cross-modal retrieval. This proposed method integrates the textual embeddings of different recipe components into a compact embedding to represent the recipes with the latent component-specific weight factors. A transformer encoder is utilised to capture the intra-modality interactions and the importance of different extracted image features for enhanced image representations. Finally, the bi-directional triplet loss is further used to perform retrieval learning. Experimental results on the Recipe 1M dataset show that our LCWF-GI method achieves competent improvements.
更多查看译文
关键词
Food-oriented cross-modal retrieval, image-recipe retrieval, cross-modal food domain retrieval, recipe representation, image representation
AI 理解论文
溯源树
样例
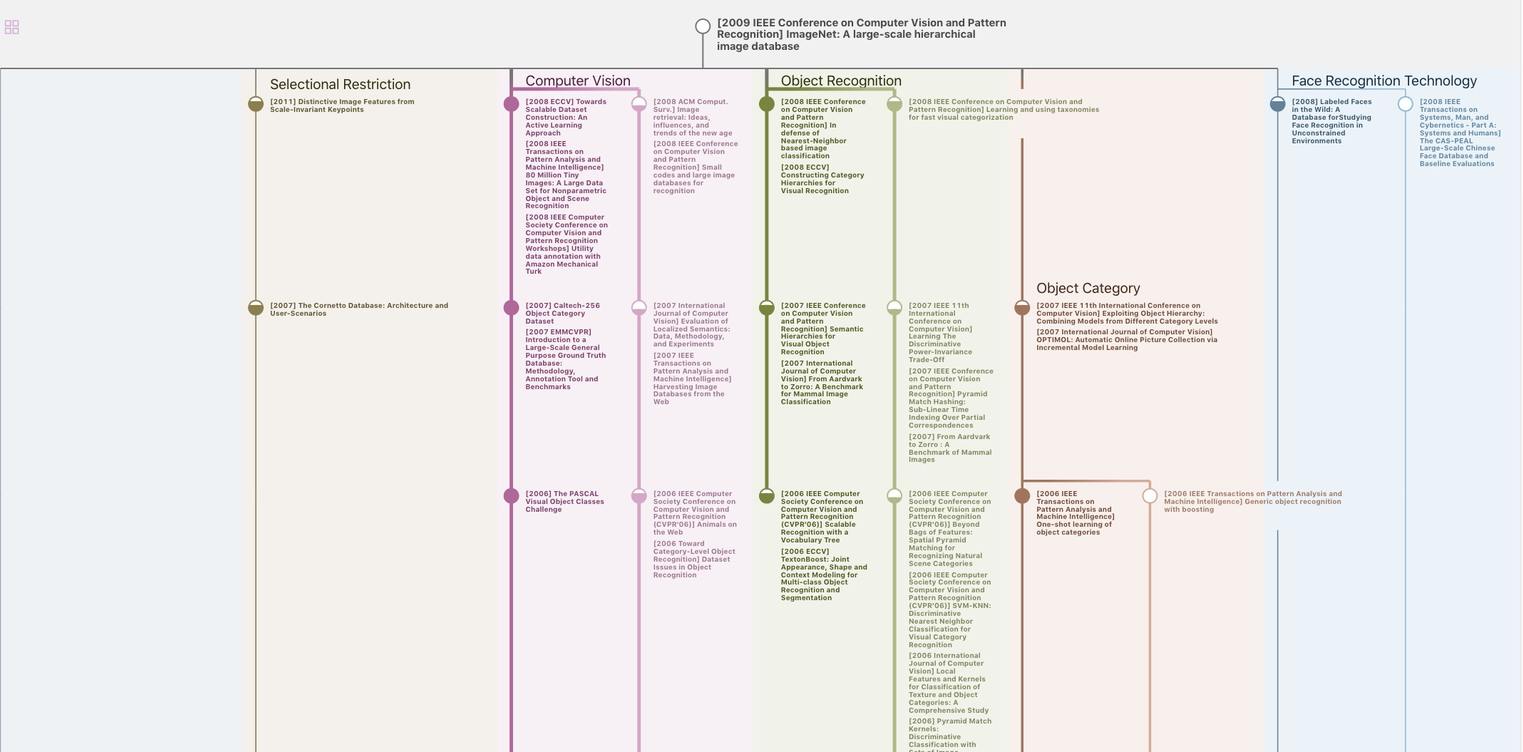
生成溯源树,研究论文发展脉络
Chat Paper
正在生成论文摘要