A Multi-Objective Optimization Approach Based on an Enhanced Particle Swarm Optimization Algorithm With Evolutionary Game Theory
IEEE Access(2023)
摘要
Due to conflicts among objectives of multi-objective optimization (MO) problems, it remains challenging to gain high-quality Pareto fronts for different MO issues. Attempt to handle this challenge and obtain high-performance Pareto fronts, this paper proposes a novel MO optimizer via leveraging particle swarm optimization (PSO) with evolutionary game theory (EGT). Firstly, a modified self-adaptive PSO (MSAPSO) adopting a novel self-adaptive parameter adaption rule determined by the evolutionary strategy of EGT to tune the three key parameters of each particle is proposed in order to well balance the exploration and exploitation abilities of MSAPSO. Then, a parameter selection principle is provided to sufficiently guarantee convergence of MSAPSO followed after the analytical convergence investigation of this optimizer so as to assure convergence of the searched Pareto front toward the true Pareto front as far as possible. Subsequently, a MSAPSO-based MO optimizer is developed, in which an external archive is applied to preserve the searched non-dominated solutions and a circular sorting method is amalgamated with the elitist-saving method to update the external archive. Lastly, the performance of the proposed method is examined by 16 benchmark test functions against 4 well-known MOO methods. The simulation results reveal that the proposed method dominates its peers regarding the quality of the Pareto fronts for most of the studied benchmarks. Furthermore, the results of the non-parametric analysis confirm that the proposed method significantly outperforms its contenders at the confidential level of 95% over the 16 benchmarks.
更多查看译文
关键词
Multi-objective optimization,particle swarm optimization,evolutionary game theory,convergence investigation,pareto front
AI 理解论文
溯源树
样例
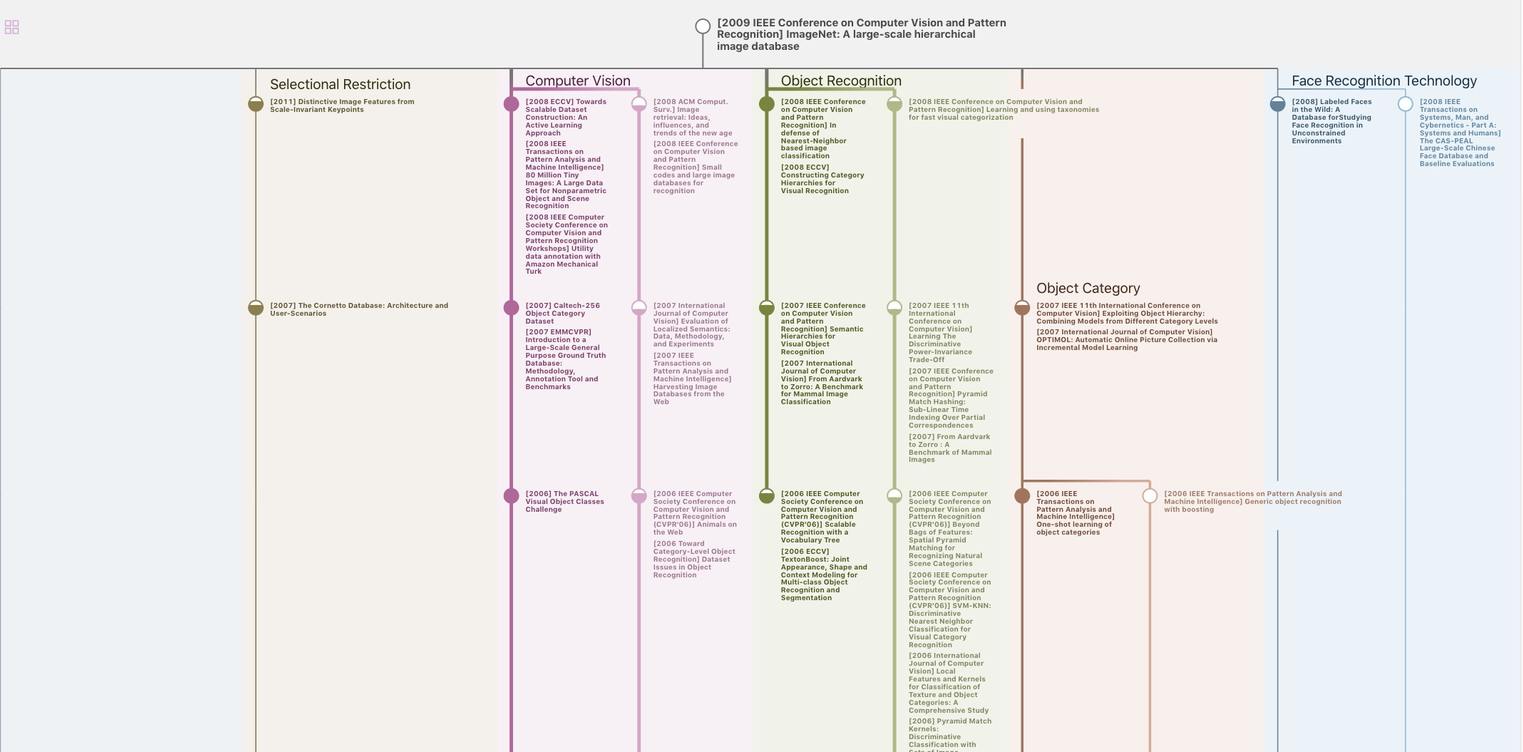
生成溯源树,研究论文发展脉络
Chat Paper
正在生成论文摘要