Multimodal Transfer Learning for Oral Presentation Assessment.
IEEE Access(2023)
摘要
Oral communication has consistently been ranked as a key skill, with 90 percent of hiring managers and 80 percent of business executives saying it is very important for college graduates to possess, according to a recent survey. Consequently, training and evaluating oral presentation skills remains a priority for educators worldwide, and there are increasing numbers of automated tools developed for providing feedback and assessment of such skills. However, modeling approaches typically require collecting large amounts of data and labels, which can be both expensive and laborious. In this paper, we explore the possibility of transfer learning between two different but related multimodal datasets to benefit the evaluation of oral presentation performance. We utilize knowledge from a job interview dataset as pretraining material and adapt the learned knowledge from the pre-trained model to a small amount of presentation data to improve the learning of the presentation assessment task. We demonstrate the efficacy of our approach, especially in improving performance for inference on small datasets (< 100 data points), and we report our findings. Moreover, we give a comparison between the proposed TL approach and a standard TL method based on a large-scale pre-trained model. Despite the simplicity of our proposed TL approach, the results show that our approach has promise in application to smaller datasets such as ours.
更多查看译文
关键词
transfer learning,presentation,assessment
AI 理解论文
溯源树
样例
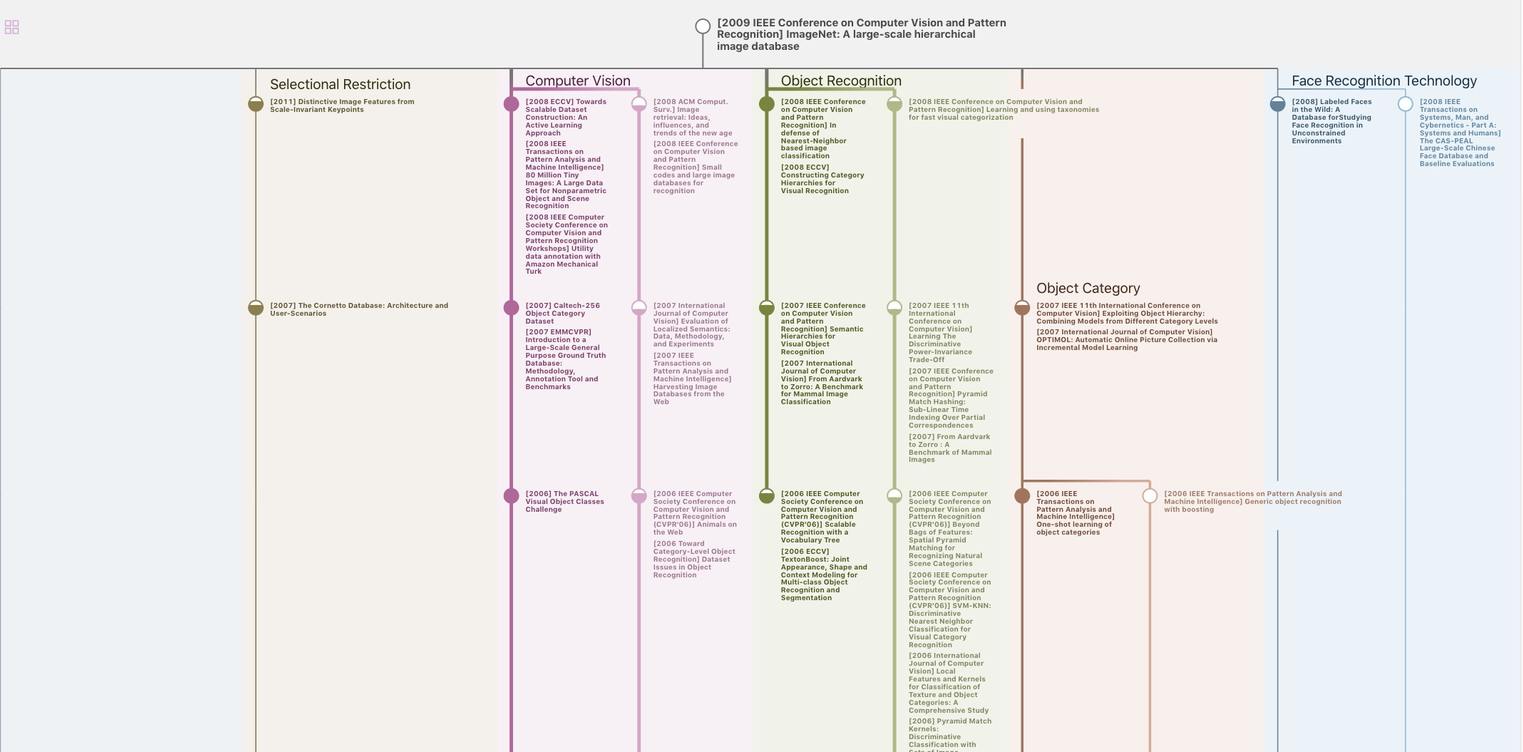
生成溯源树,研究论文发展脉络
Chat Paper
正在生成论文摘要