Defending AI-Based Automatic Modulation Recognition Models Against Adversarial Attacks
IEEE Access(2023)
摘要
Automatic Modulation Recognition (AMR) is one of the critical steps in the signal processing chain of wireless networks, which can significantly improve communication performance. AMR detects the modulation scheme of the received signal without any prior information. Recently, many Artificial Intelligence (AI) based AMR methods have been proposed, inspired by the considerable progress of AI methods in various fields. On the one hand, AI-based AMR methods can outperform traditional methods in terms of accuracy and efficiency. On the other hand, they are susceptible to new types of cyberattacks, such as model poisoning or adversarial attacks. This paper explores the vulnerabilities of an AI-based AMR model to adversarial attacks in both single-input-single-output and multiple-input-multiple-output scenarios. We show that these attacks can significantly reduce the classification performance of the AI-based AMR model, which highlights the security and robustness concerns. Therefore, we propose a widely used mitigation method (i.e., defensive distillation) to reduce the vulnerabilities of the model against adversarial attacks. The simulation results indicate that the AI-based AMR model can be highly vulnerable to adversarial attacks, but their vulnerabilities can be significantly reduced by using mitigation methods.
更多查看译文
关键词
& nbsp,Artificial intelligence,next-generation networks,automatic modulation recognition,adversarial attacks,model poisoning,defensive distillation
AI 理解论文
溯源树
样例
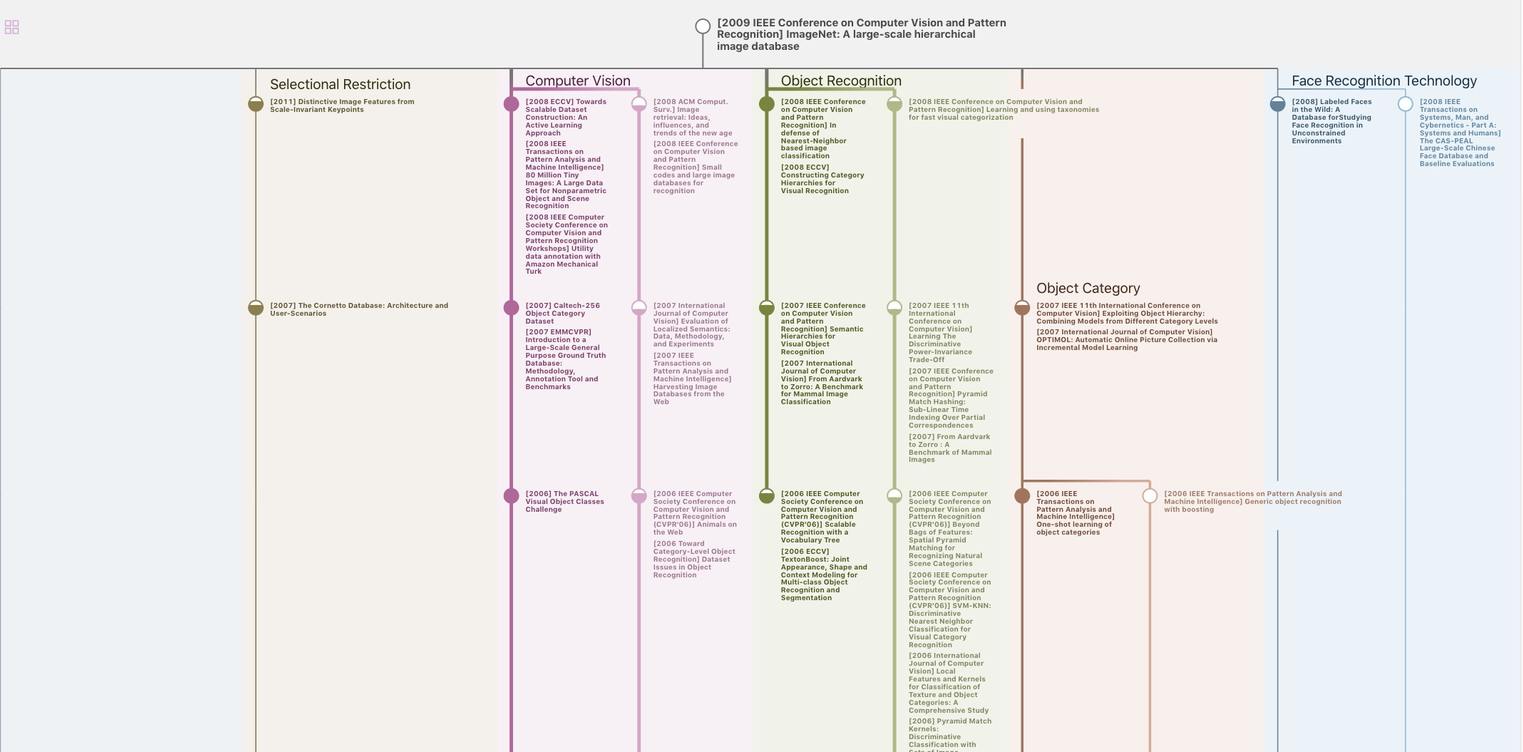
生成溯源树,研究论文发展脉络
Chat Paper
正在生成论文摘要