High-Precision Identification of Power Quality Disturbances Based on Discrete Orthogonal S-Transforms and Compressed Neural Network Methods
IEEE Access(2023)
摘要
Power quality disturbances (PQDs) occur as the use of non-linear load and renewable-based micro-grids increases. This paper presents a new algorithm that consists of the discrete orthogonal S-transform (DOST) in the feature extraction stage, compressive sensing (CS) in the feature reduction stage, and a deep stacking network (DSN) for the automatic classification of single and multiple PQDs. It compresses the extracted feature matrix (orthogonal S-matrix coefficients) to minimize the computational process and provide more diversified features. Firstly, PQDs data is generated from a modified IEEE 13 bus system with wind grid integration, both synthetically and in real time. Moreover, compressive measurements of 24 types of multiple PQDs events and nine types of single PQDs events of synthetic and real data, and 12 type of three-phase single and multiple PQDs from the modified IEEE wind grid integration are fed to a proposed DSN classifier for PQD recognition. The DOST-based CS feature extraction technique achieves good robustness and time-frequency localization while retaining useful information. The DSN classifier method utilizes a Batch-mode gradient as a fine-tune, which has less noise gradient and improved efficiency of PQD classification. A noise level of 20 dB to 50 dB is considered. Other models, such as k-Nearest Neighbor (KNN), Multiclass Support Vector Machine (MSVM), and ensemble learner methods, are also developed to compare the efficiency. The high classification results demonstrate that the DOST-CS feature extraction and the DSN classifier have high precision in identifying multiple power quality events, even in noisy conditions.
更多查看译文
关键词
Multiple power quality disturbances identification,compressed sensing,discrete orthogonal S-transform,deep neural network,wind-grid distribution
AI 理解论文
溯源树
样例
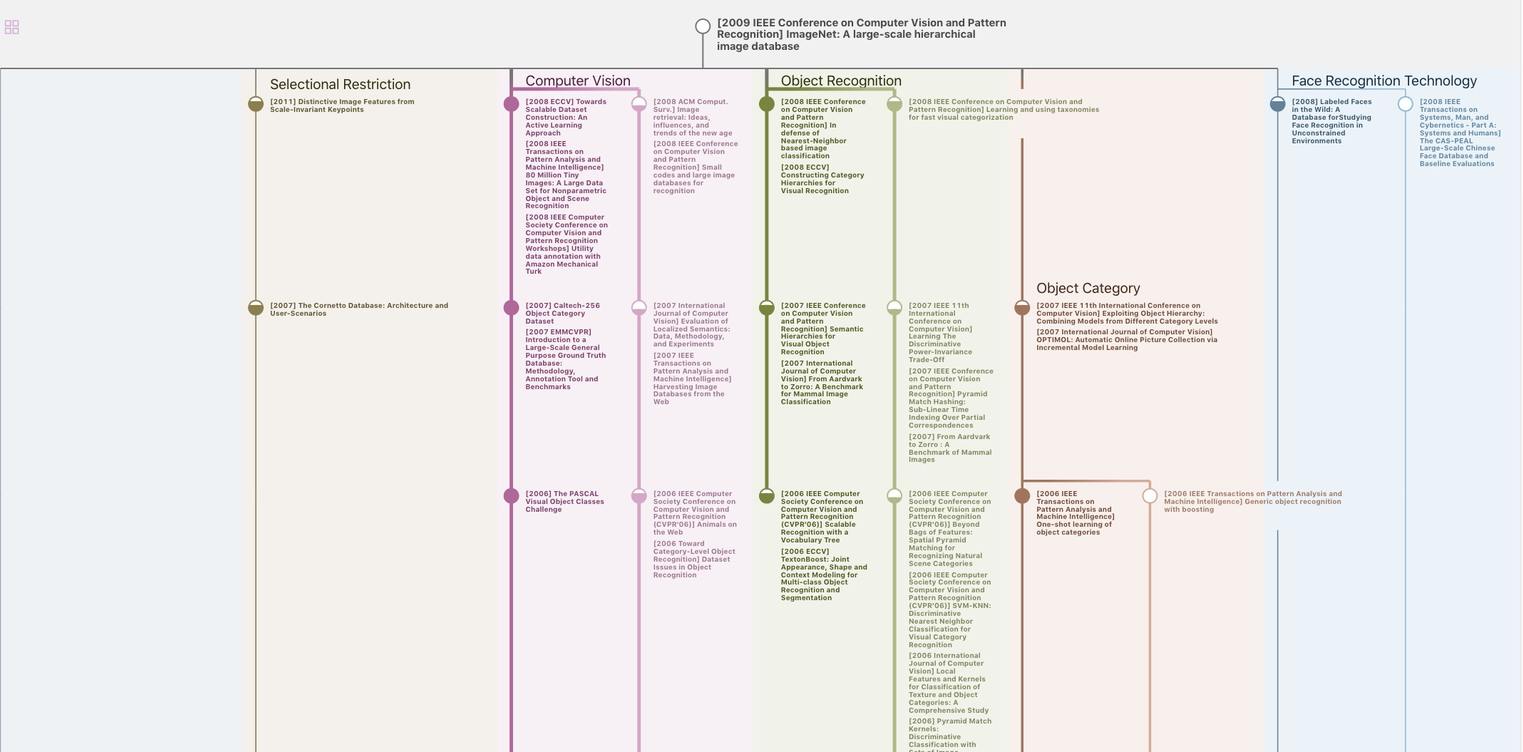
生成溯源树,研究论文发展脉络
Chat Paper
正在生成论文摘要