MDGAD: Meta domain generalization for distribution drift in anomaly detection
Neurocomputing(2023)
摘要
Anomalies often experience distribution drift over time in anomaly detection. Traditional anomaly detec-tion methods detect anomalies with the same distribution effectively, but it is difficult to detect anoma-lies with changing distributions. In this paper, we propose a meta domain generalization based anomaly detection (MDGAD) framework to detect anomalies when distribution drifts. The framework first divides the data into a series of subsets. We measure the domain shift between sets with a class-sensitive dis-tance metric, and merge similar sets, thereby generating different source domains to enhance domain diversity. We utilize the distribution shifts that existed on these source domains to simulate the distri-bution shifts that would be encountered when the model was applied. Meta learning is employed during training. First, the network is updated once on the meta-training set, and then another update is per-formed on the meta-verification set. These two gradients together determine the update direction of the network. At the same time, our framework will complete the domain alignment task on multiple source domains to extract domain invariant features and enhance the generalization of feature learning. We use Devnet as the base model and test our framework on 5 simulated data sets. The results confirm that our MDGAD outperforms current popular algorithms in detecting unknown anomalies and has better robustness. In addition, we also test our framework on real financial datasets to demonstrate the effectiveness.& COPY; 2023 Elsevier B.V. All rights reserved.
更多查看译文
关键词
Anomaly detection,Distribution drift,Domain generalization,Meta learning
AI 理解论文
溯源树
样例
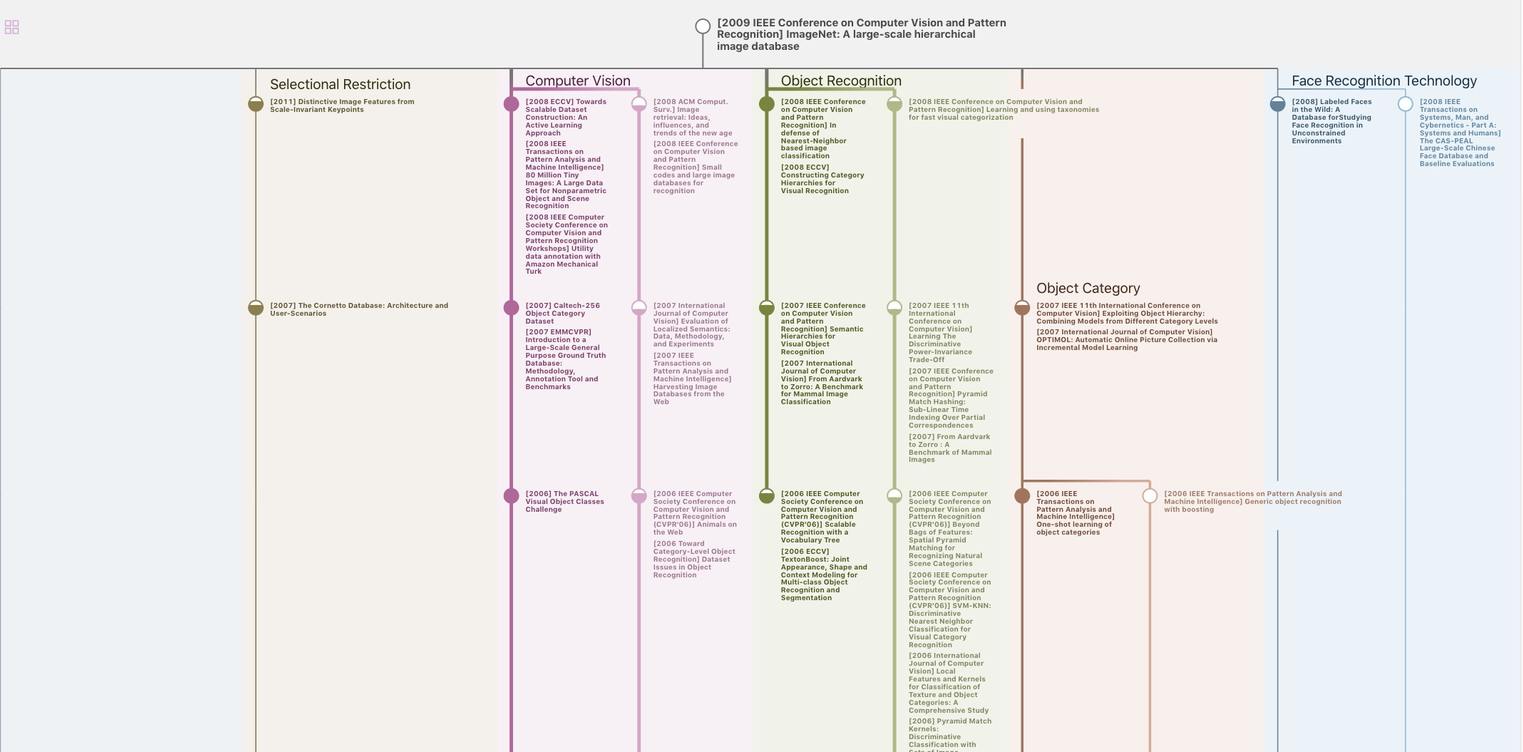
生成溯源树,研究论文发展脉络
Chat Paper
正在生成论文摘要