A Convex Reformulation and an Outer Approximation for a Large Class of Binary Quadratic Programs.
Oper. Res.(2023)
摘要
Binary Quadratic Program with Variable Partitioning Constraints The binary quadratic program with variable partitioning constraints is a very general class of optimization problems that is very difficult to solve because of the nonconvexity and integrality of the variables and is ubiquitous, among others, in network design, computer vision, and transportation and logistics. In their article, “A convex reformulation and an outer approximation for a large class of binary quadratic programs,” Rostami et al. show how to transform such a nonconvex challenging problem into a convex bilinear program with decomposable structure. The authors develop a branch-and-cut algorithm based on outer approximation cuts, in which the cuts are generated on the fly by efficiently solving separation subproblems. The results of their computational experiments on different problems confirm the efficacy of the solution methods in solving large-scale problem instances. In this paper, we propose a general modeling and solving framework for a large class of binary quadratic programs subject to variable partitioning constraints. Problems in this class have a wide range of applications as many binary quadratic programs with linear constraints can be represented in this form. By exploiting the structure of the partitioning constraints, we propose mixed-integer nonlinear programming (MINLP) and mixed-integer linear programming (MILP) reformulations and show the relationship between the two models in terms of the relaxation strength. Our solution methodology relies on a convex reformulation of the proposed MINLP and a branch-and-cut algorithm based on outer approximation cuts, in which the cuts are generated on the fly by efficiently solving separation subproblems. To evaluate the robustness and efficiency of our solution method, we perform extensive computational experiments on various quadratic combinatorial optimization problems. The results show that our approach outperforms the state-of-the-art solver applied to different MILP reformulations of the corresponding problems. Funding: This work was supported by the Natural Sciences and Engineering Research Council of Canada [Grants RGPIN- 2020-05395 and RGPIN 2015-06289] and Fonds de Recherche du Québec - Nature et technologies [FRQNT NC-198928].
更多查看译文
关键词
Optimization,binary quadratic program,convex reformulation,outer approximation,variable partitioning constraint
AI 理解论文
溯源树
样例
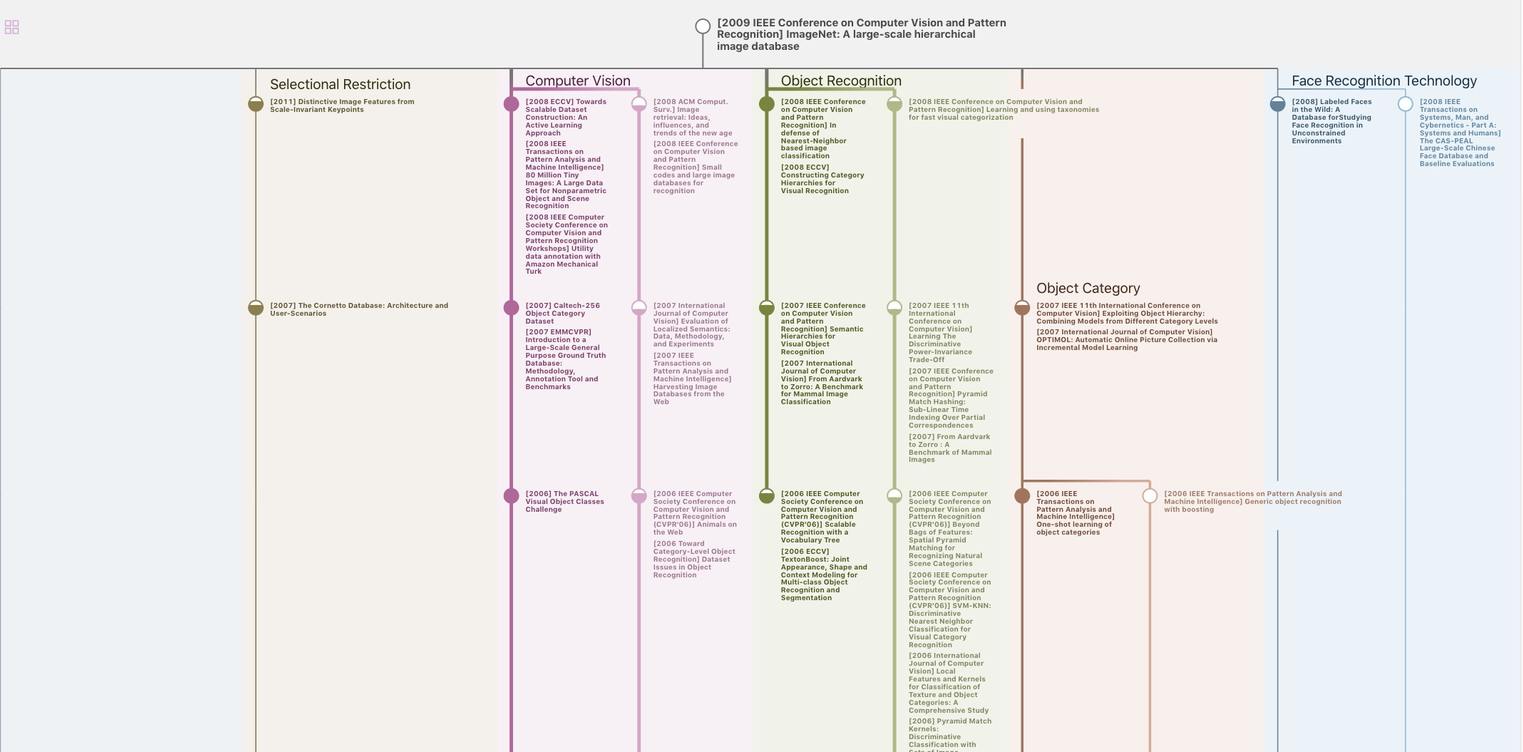
生成溯源树,研究论文发展脉络
Chat Paper
正在生成论文摘要