Model-Free Neural Fault Detection and Isolation for Safe Control
IEEE Control. Syst. Lett.(2023)
摘要
A sudden actuator fault in a safety-critical system can cause safety violations and lead to severe consequences. Existing fault-tolerant control (FTC) approaches normally focus on maintaining system performance and do not consider system safety. Control Barrier Functions (CBFs) have emerged as useful tools from control theory for providing safety guarantees for control systems. However, existing applications of CBFs either do not consider actuator faults or only consider the special case where it is known which actuator is faulty or the case when redundant actuators are present to maintain controllability even under faults and failures. In this letter, we address the problem of safe recovery under a more realistic scenario where it is completely unknown which actuator is faulty and when the fault occurs. We develop a novel model-free learning framework for an output-based neural fault-detector that detects when a fault occurs and in which actuator. Based on the learned functions, we propose a switching framework for automatically detecting and recovering from faults. We evaluate our method on a case study involving a Crazyflie quadrotor with a motor failure.
更多查看译文
关键词
Fault detection,machine learning,neural networks
AI 理解论文
溯源树
样例
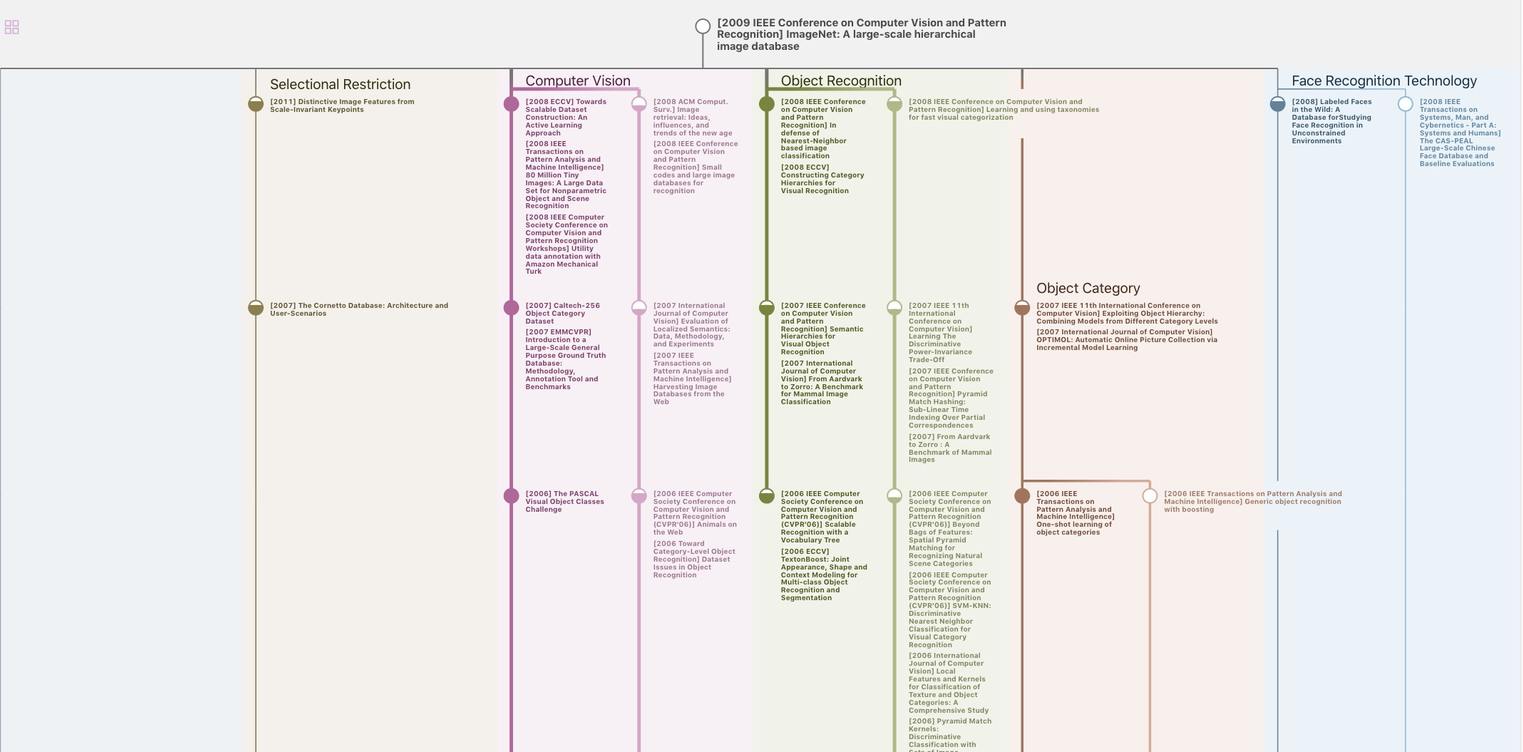
生成溯源树,研究论文发展脉络
Chat Paper
正在生成论文摘要