VARF: An Incentive Mechanism of Cross-Silo Federated Learning in MEC.
IEEE Internet Things J.(2023)
摘要
Cross-silo federated learning (FL) is a privacy-preserving distributed machine learning where organizations acting as clients cooperatively train a global model without uploading their raw local data. Recently, the cross-silo FL in multiaccess edge computing (MEC) is used in increasing industrial applications. Most existing research on cross-silo FL pays attention to the performance aspect, ignoring the incentive mechanism for high-quality client selection and long participation in model training for efficient and stable FL, which has prevented the widespread adoption of cross-silo FL in MEC. In this article, we propose an incentive mechanism with quality-Aware and reputation-Aware based on the infinitely repeated game for cross-silo FL named VARF. VARF selects high-quality and high-reputation edge nodes (ENs) as candidates for model training in the cross-silo FL by a heuristic algorithm and then motivates the selected ENs to actively contribute their resources. VARF also models the long-term behavior of ENs in cross-silo FL as an infinitely repeated game and derives a stable and long-term cooperative strategy for clients while maximizing the amount of local data for model learning in cross-silo FL. Extensive simulations with real-world data sets demonstrate that the performance of VARF is more beneficial than other benchmarks. Meanwhile, experimental results show that cloud platforms (CPs) and ENs eventually form a long and stable cooperative relationship under the trigger strategy.
更多查看译文
关键词
federated learning,incentive mechanism,cross-silo
AI 理解论文
溯源树
样例
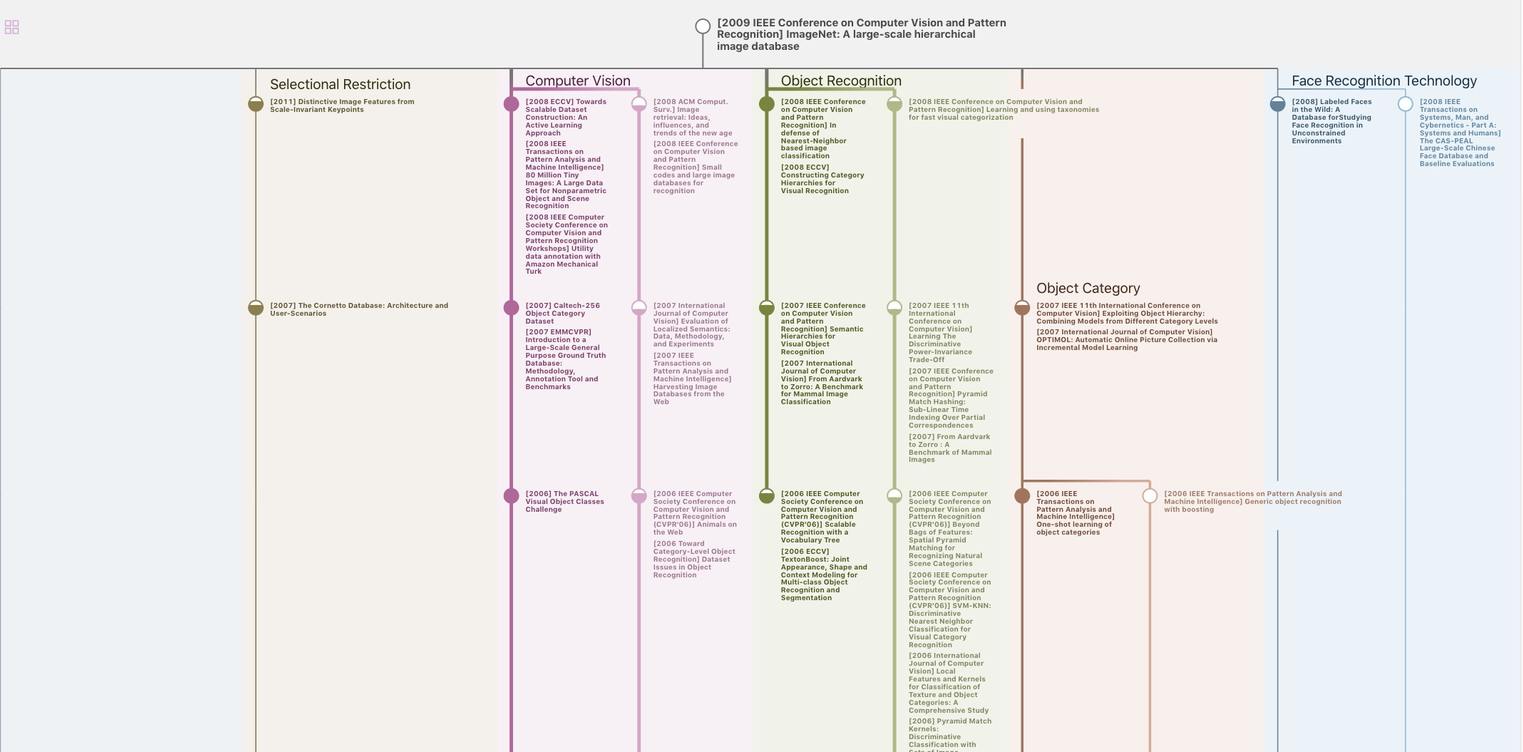
生成溯源树,研究论文发展脉络
Chat Paper
正在生成论文摘要