PPeFL: Privacy-Preserving Edge Federated Learning With Local Differential Privacy.
IEEE Internet Things J.(2023)
摘要
Since traditional federated learning (FL) algorithms cannot provide sufficient privacy guarantees, an increasing number of approaches apply local differential privacy (LDP) techniques to FL to provide strict privacy guarantees. However, the privacy budget heavily increases proportionally with the dimension of the parameters, and the large variance generated by the perturbation mechanisms leads to poor performance of the final model. In this article, we propose a novel privacy-preserving edge FL framework based on LDP (PPeFL). Specifically, we present three LDP mechanisms to address the privacy problems in the FL process. The proposed filtering and screening with exponential mechanism (FS-EM) filters out the better parameters for global aggregation based on the contribution of weight parameters to the neural network. Thus, we can not only solve the problem of fast growth of privacy budget when applying perturbation mechanism locally but also greatly reduce the communication costs. In addition, the proposed data perturbation mechanism with stronger privacy (DPM-SP) allows a secondary scrambling of the original data of participants and can provide strong security. Further, a data perturbation mechanism with enhanced utility (DPM-EU) is proposed in order to reduce the variance introduced by the perturbation. Finally, extensive experiments are performed to illustrate that the PPeFL scheme is practical and efficient, providing stronger privacy protection while ensuring utility.
更多查看译文
关键词
Edge computing,federated learning (FL),local differential privacy (LDP),privacy protection
AI 理解论文
溯源树
样例
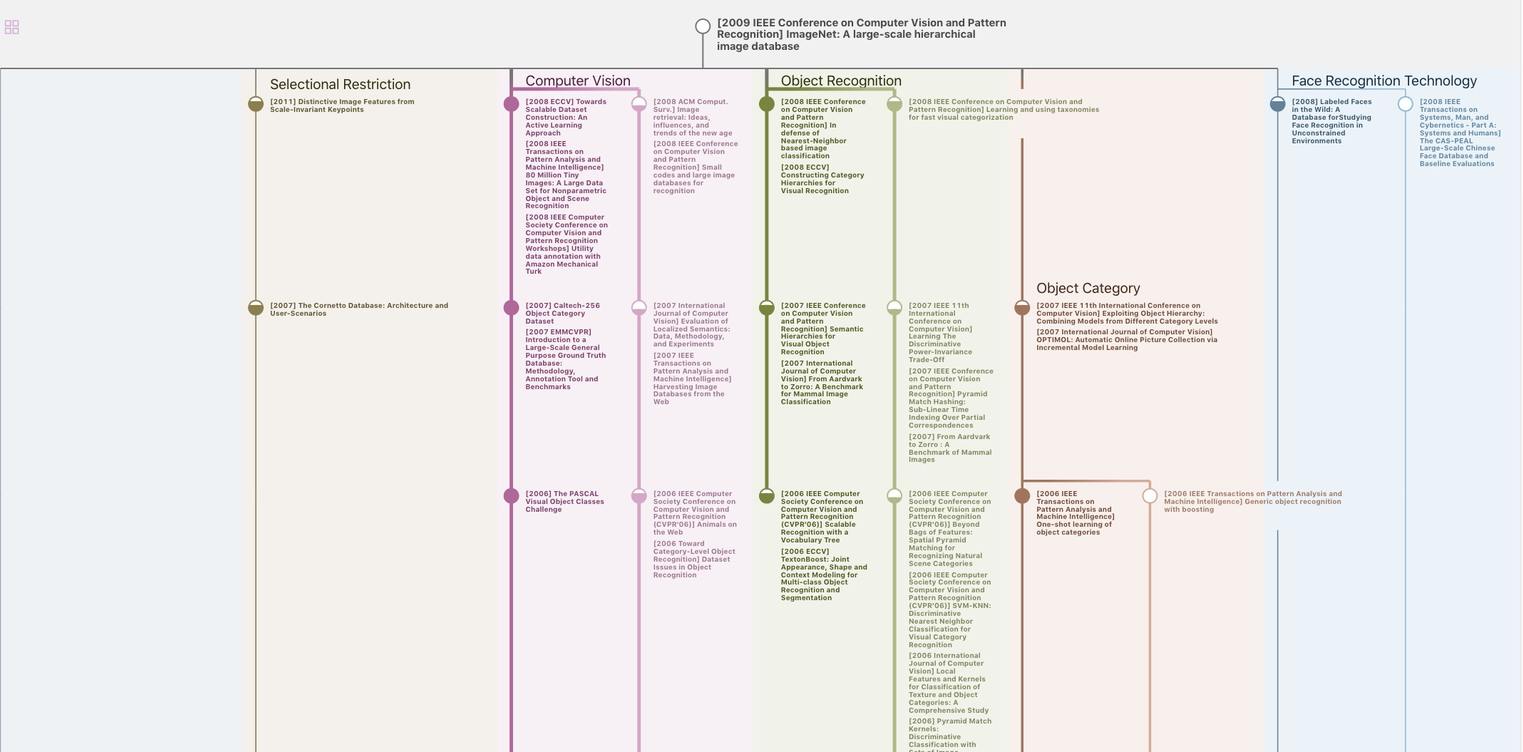
生成溯源树,研究论文发展脉络
Chat Paper
正在生成论文摘要