A GAN-Based Defense Framework Against Model Inversion Attacks.
IEEE Trans. Inf. Forensics Secur.(2023)
摘要
With the development of deep learning, deep neural network (DNN)-based application have become an indispensable aspect of daily life. However, recent studies have shown that these well-trained DNN models are vulnerable to model inversion attacks (MIAs), where attackers can recover their training data with high fidelity. Although several defensive strategies have been proposed to mitigate the impact of such attacks, existing defenses will inevitably compromise the model performance and are ineffective against more sophisticated attacks, such as Mirror (An et al., 2022). In this paper, we introduce a novel GAN-based defense approach against model inversion attacks. Unlike previous works that perturb the prediction vector of the model, we manipulate the training procedure of the victim model by incorporating carefully-designed GAN-based fake samples. We also adjust the loss of the inversed samples to inject misleading features into the protected label of the victim model. Additionally, we adopt the concept of continual learning to improve the utility of the model. Extensive experiments conducted on the CelebA, VGG-Face, and VGG-Face2 datasets demonstrate that our proposed method outperforms existing defenses against state-of-the-art model inversion attacks, including DMI (Chen et al., 2021), Mirror (An et al., 2022), Privacy (Fredrikson et al., 2014), and AMI (Yang et al., 2019). It is shown that our proposed method can also retain a high defense performance in black-box scenarios.
更多查看译文
关键词
Model inversion attacks, GAN-based fake sample generation, privacy-utility defense framework
AI 理解论文
溯源树
样例
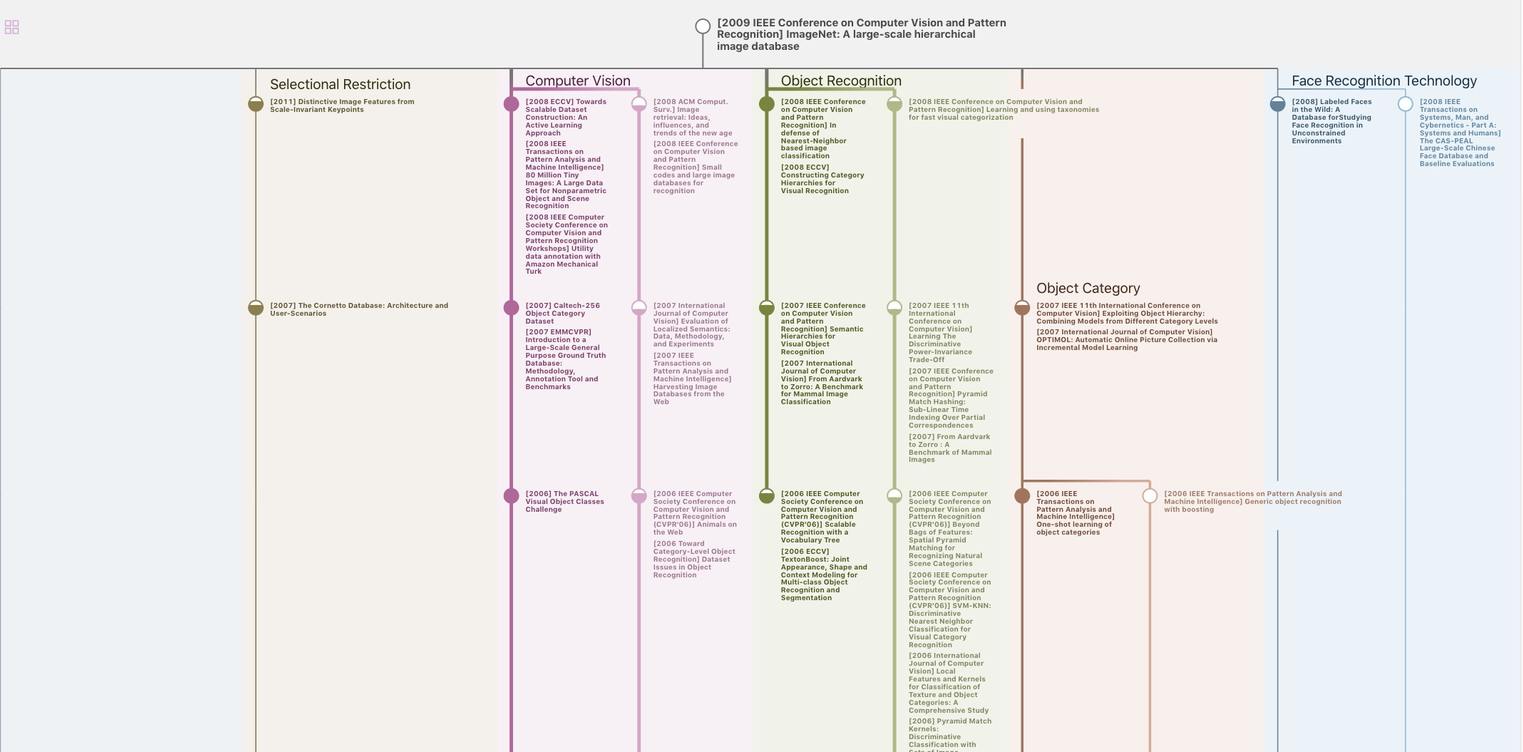
生成溯源树,研究论文发展脉络
Chat Paper
正在生成论文摘要