LSD: Adversarial Examples Detection Based on Label Sequences Discrepancy.
IEEE Trans. Inf. Forensics Secur.(2023)
摘要
Deep neural network (DNN) models have been widely used in many tasks due to their superior performance. However, DNN models are usually vulnerable to adversarial example attacks, which limits their applications in many safety-critic scenarios. How to effectively detect adversarial examples to enhance the robustness of DNN models has attracted much attention in recent years. Most adversarial example detection methods require modifying or retraining the model, which is impractical and reduces the classification accuracy of normal examples. In this paper, we propose an adversarial example detection approach that does not require modification of the DNN models and meanwhile retains the classification accuracy of normal examples. The key observation is that when we transform the input example with some operations (e.g., masking a pixel with a reference value), feed the transformed example to the target model, and use the output of the intermediate layers to predict the label of the example, the generated label sequences of adversarial examples will be extremely discrepant but the label sequences of normal examples keep nearly unchanged. Motivated by this observation, we design an approach to detect adversarial examples based on the label sequence discrepancy (LSD) of the given examples. The experimental results against five mainstream adversarial attacks on three benchmark datasets demonstrate that LSD outperforms the state-of-the-art solutions in the detection rate of adversarial examples. Moreover, LSD performs well at various confidence levels and exhibits good generalizability between different attacks.
更多查看译文
关键词
adversarial examples detection,label sequences discrepancy,lsd
AI 理解论文
溯源树
样例
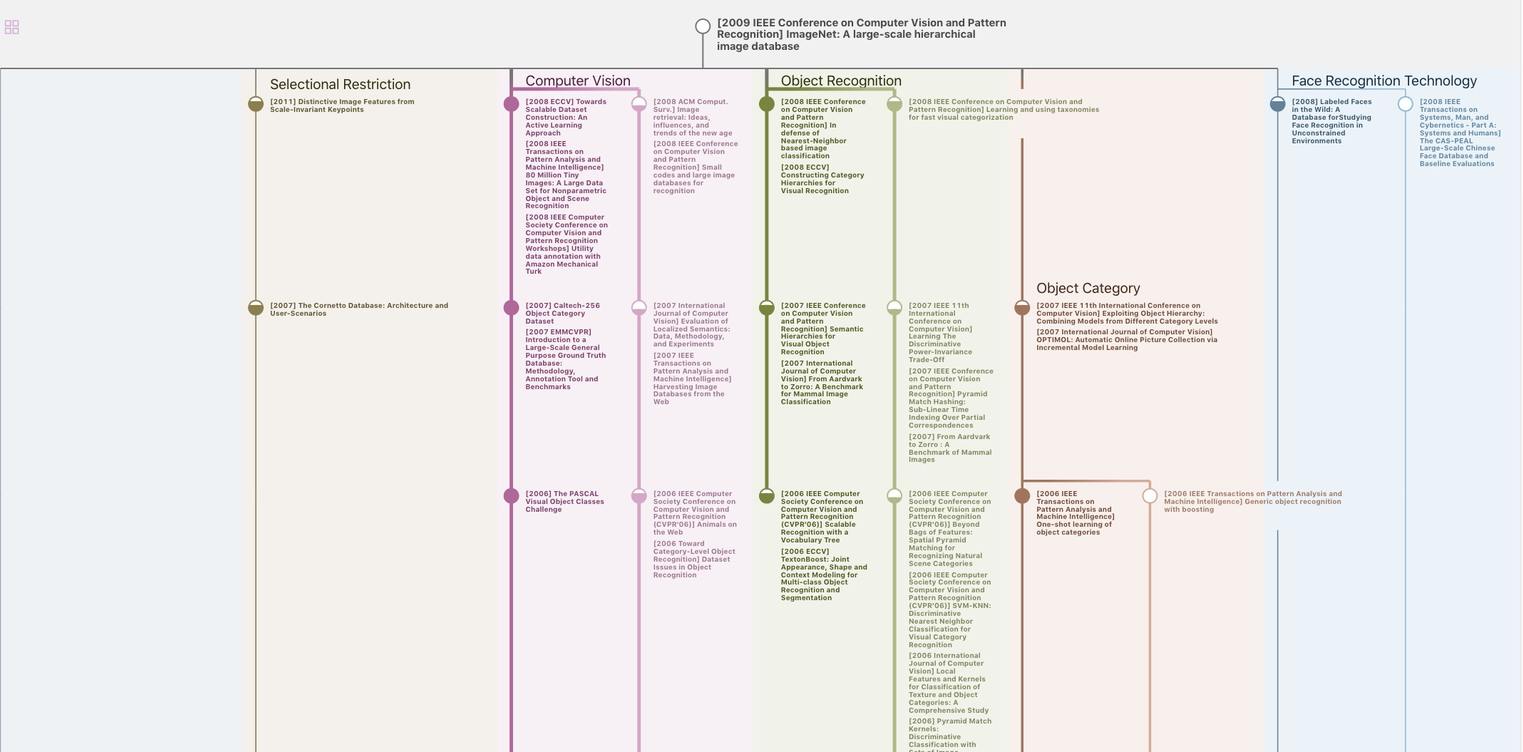
生成溯源树,研究论文发展脉络
Chat Paper
正在生成论文摘要