BSFormer: Transformer-Based Reconstruction Network for Hyperspectral Band Selection
IEEE Geosci. Remote. Sens. Lett.(2023)
摘要
Band selection (BS) is an effective approach to alleviate the spectral redundancy of a hyperspectral image (HSI). The emerging deep-learning-based BS methods have become a hot topic due to their ability to model nonlinear relationships between spectral bands. However, existing deep-learning-based BS methods fail to accurately extract the representativeness of each band as a result of the limitation of interpretation networks. Moreover, existing deep-learning methods cannot fully utilize the interband correlation and the spatial information of HSIs for BS. To solve these issues, in this letter, we propose a novel Transformer reconstruction network for unsupervised BS, termed BSFormer. Specifically, the Transformer reconstruction network, which contributes to leveraging the spectral-spatial information of the HSI, consists of a Transformer-based band attention (TBA) module and a convolutional autoencoder (CAE)-based reconstruction module. On this basis, we design a novel band evaluation criterion composed of representative metric and redundancy metric, which are interpreted with the help of the multihead self-attention layer in the TBA module. The designed criterion can fully use the band representativeness and interband correlation for BS. Experimental results on three well-known hyperspectral datasets verify that the proposed BSFormer can yield better classification performance than the competitors.
更多查看译文
关键词
Autoencoder (AE),hyperspectral image (HSI),Transformer,unsupervised band selection (BS)
AI 理解论文
溯源树
样例
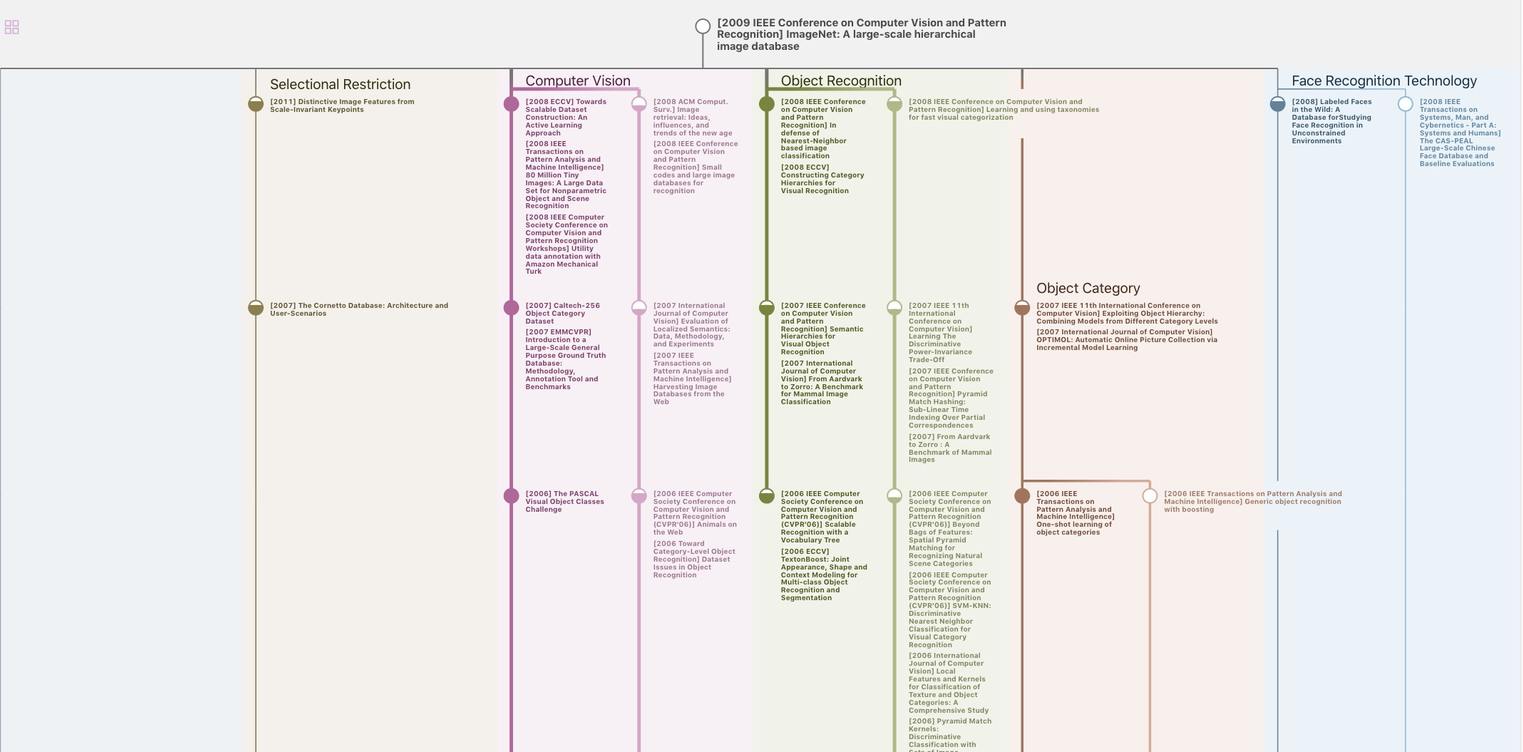
生成溯源树,研究论文发展脉络
Chat Paper
正在生成论文摘要