RetroBridge: Modeling Retrosynthesis with Markov Bridges
ICLR 2024(2024)
摘要
Retrosynthesis planning is a fundamental challenge in chemistry which aims at designing reaction pathways from commercially available starting materials to a target molecule. Each step in multi-step retrosynthesis planning requires accurate prediction of possible precursor molecules given the target molecule and confidence estimates to guide heuristic search algorithms. We model single-step retrosynthesis planning as a distribution learning problem in a discrete state space. First, we introduce the Markov Bridge Model, a generative framework aimed to approximate the dependency between two intractable discrete distributions accessible via a finite sample of coupled data points. Our framework is based on the concept of a Markov bridge, a Markov process pinned at its endpoints. Unlike diffusion-based methods, our Markov Bridge Model does not need a tractable noise distribution as a sampling proxy and directly operates on the input product molecules as samples from the intractable prior distribution. We then address the retrosynthesis planning problem with our novel framework and introduce RetroBridge, a template-free retrosynthesis modeling approach that achieves state-of-the-art results on standard evaluation benchmarks.
更多查看译文
关键词
Retrosynthesis,Reactions,Chemistry,Drug Discovery,Markov Bridge
AI 理解论文
溯源树
样例
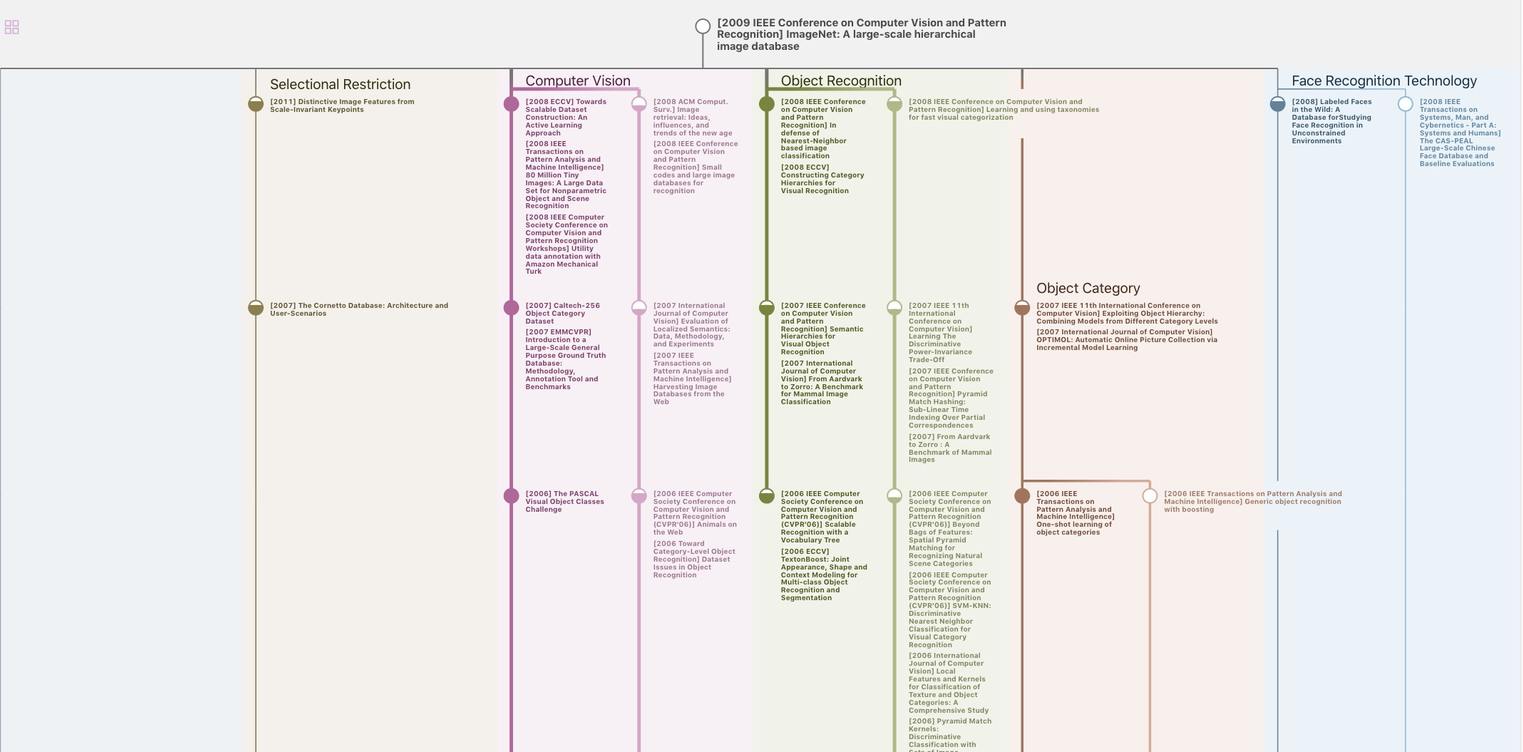
生成溯源树,研究论文发展脉络
Chat Paper
正在生成论文摘要