Robust Networked Federated Learning for Localization
2023 ASIA PACIFIC SIGNAL AND INFORMATION PROCESSING ASSOCIATION ANNUAL SUMMIT AND CONFERENCE, APSIPA ASC(2023)
摘要
This paper addresses the problem of localization, which is inherently non-convex and non-smooth in a federated setting where the data is distributed across a multitude of devices. Due to the decentralized nature of federated environments, distributed learning becomes essential for scalability and adaptability. Moreover, these environments are often plagued by outlier data, which presents substantial challenges to conventional methods, particularly in maintaining estimation accuracy and ensuring algorithm convergence. To mitigate these challenges, we propose a method that adopts an L1-norm robust formulation within a distributed sub-gradient framework, explicitly designed to handle these obstacles. Our approach addresses the problem in its original form, without resorting to iterative simplifications or approximations, resulting in enhanced computational efficiency and improved estimation accuracy. We demonstrate that our method converges to a stationary point, highlighting its effectiveness and reliability. Through numerical simulations, we confirm the superior performance of our approach, notably in outlier-rich environments, which surpasses existing state-of-the-art localization methods.
更多查看译文
关键词
Federated learning,Robust learning,distributed learning,localization,non-convex and non-smooth optimization
AI 理解论文
溯源树
样例
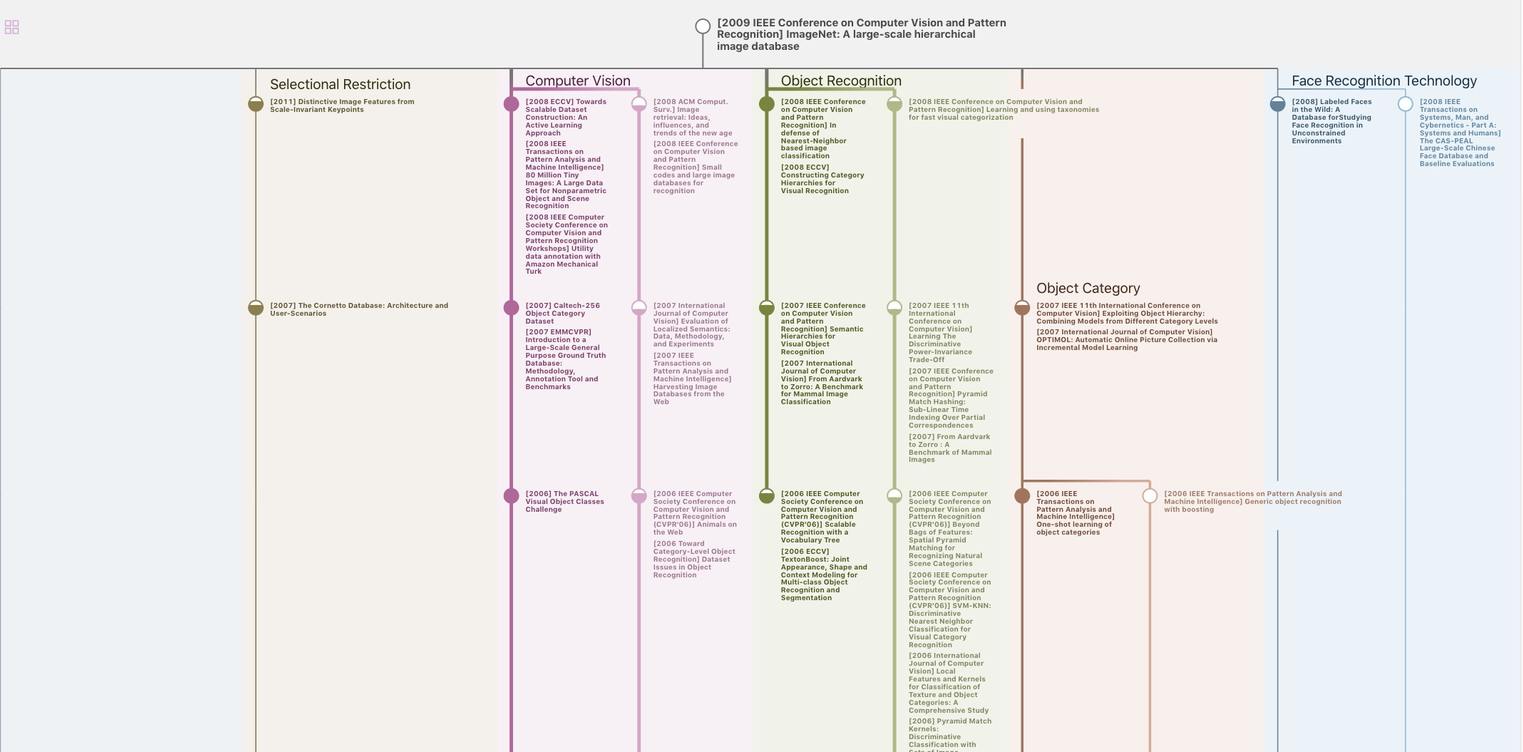
生成溯源树,研究论文发展脉络
Chat Paper
正在生成论文摘要