Sparsesat-nerf: dense depth supervised neural radiance fields for sparse satellite images
GEOSPATIAL WEEK 2023, VOL. 10-1(2023)
摘要
Digital surface model generation using traditional multi-view stereo matching (MVS) performs poorly over non-Lambertian surfaces, with asynchronous acquisitions, or at discontinuities. Neural radiance fields (NeRF) offer a new paradigm for reconstructing surface geometries using continuous volumetric representation. NeRF is self-supervised, does not require ground truth geometry for training, and provides an elegant way to include in its representation physical parameters about the scene, thus potentially remedying the challenging scenarios where MVS fails. However, NeRF and its variants require many views to produce convincing scene's geometries which in earth observation satellite imaging is rare. In this paper we present SparseSat-NeRF (SpS-NeRF) - an extension of Sat-NeRF adapted to sparse satellite views. SpS-NeRF employs dense depth supervision guided by cross-correlation similarity metric provided by traditional semi-global MVS matching. We demonstrate the effectiveness of our approach on stereo and tri-stereo Pleiades 1B/WorldView-3 images, and compare against NeRF and Sat-NeRF. The code is available at https://github.com/LulinZhang/SpS-NeRF
更多查看译文
关键词
neural radiance fields,depth supervision,multi-view stereo matching,satellite images,sparse views
AI 理解论文
溯源树
样例
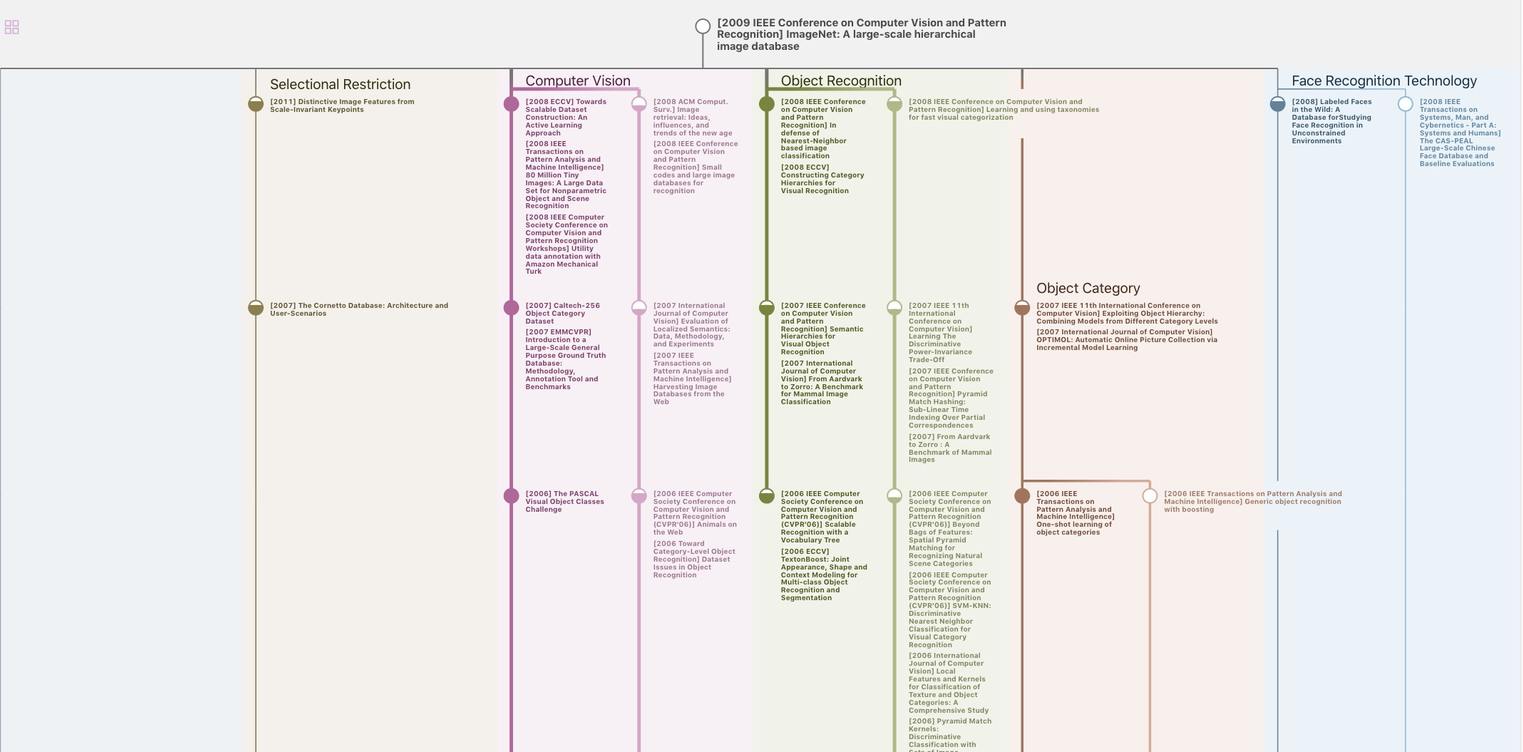
生成溯源树,研究论文发展脉络
Chat Paper
正在生成论文摘要