Convergence Analysis of the Best Response Algorithm for Time-Varying Games
2023 62ND IEEE CONFERENCE ON DECISION AND CONTROL, CDC(2023)
摘要
This paper studies a class of strongly monotone games involving non-cooperative agents that optimize their own time-varying cost functions. We assume that the agents can observe other agents' historical actions and choose actions that best respond to other agents' previous actions; we call this a best response scheme. We start by analyzing the convergence rate of this best response scheme for standard time-invariant games. Specifically, we provide a sufficient condition on the strong monotonicity parameter of the time-invariant games under which the proposed best response algorithm achieves exponential convergence to the static Nash equilibrium. We further illustrate that this best response algorithm may oscillate when the proposed sufficient condition fails to hold, which indicates that this condition is tight. Next, we analyze this best response algorithm for time-varying games where the cost functions of each agent change over time. Under similar conditions as for time-invariant games, we show that the proposed best response algorithm stays asymptotically close to the evolving equilibrium. We do so by analyzing both the equilibrium tracking error and the dynamic regret. Numerical experiments on economic market problems are presented to validate our analysis.
更多查看译文
关键词
best response algorithm,games,time-varying
AI 理解论文
溯源树
样例
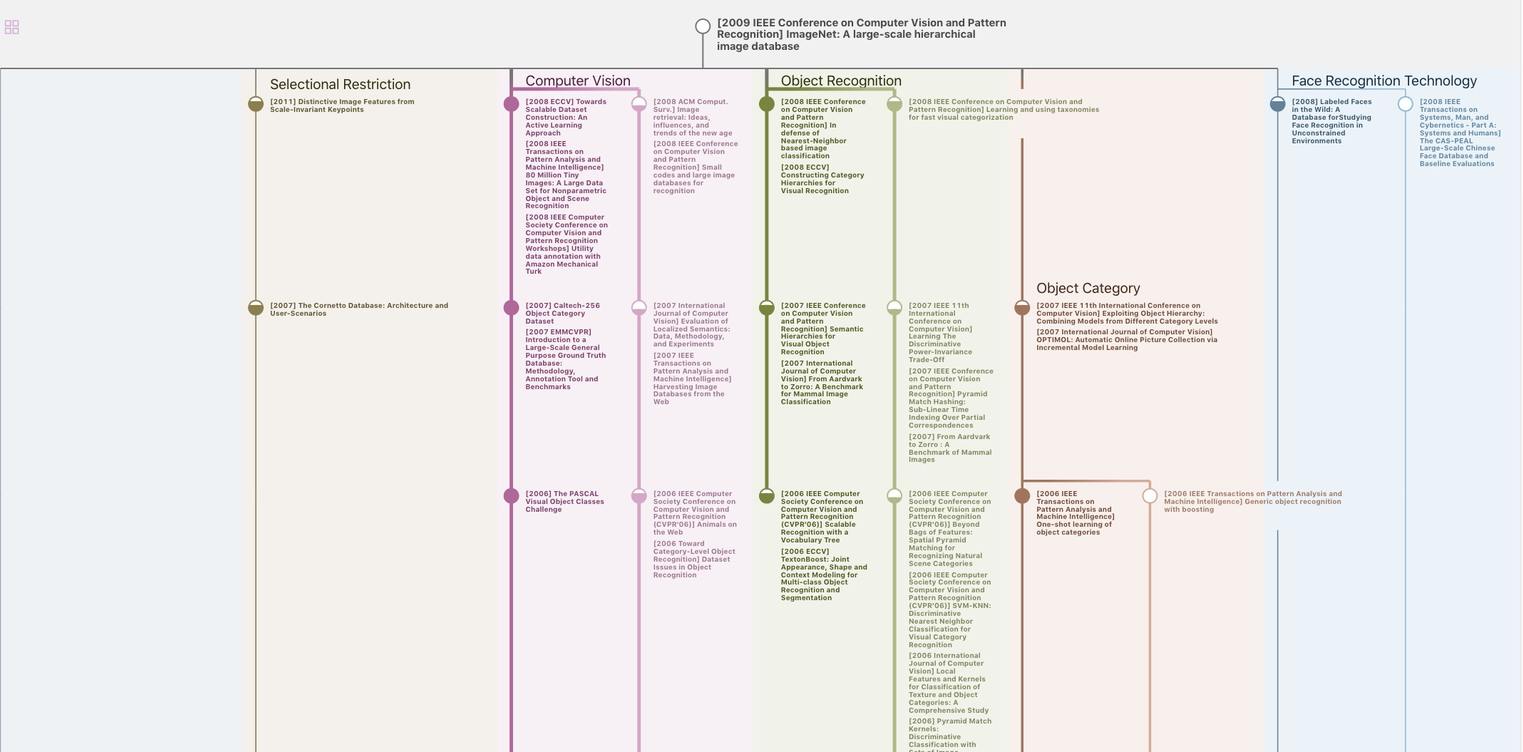
生成溯源树,研究论文发展脉络
Chat Paper
正在生成论文摘要