Catalyst Energy Prediction with CatBERTa: Unveiling Feature Exploration Strategies Through Large Language Models
arXivorg(2023)
摘要
Efficient catalyst screening necessitates predictive models for adsorption energy, a key property of reactivity. However, prevailing methods, notably graph neural networks (GNNs), demand precise atomic coordinates for constructing graph representations, while integrating observable attributes remains challenging. This research introduces CatBERTa, an energy prediction Transformer model using textual inputs. Built on a pretrained Transformer encoder, CatBERTa processes human-interpretable text, incorporating target features. Attention score analysis reveals CatBERTa's focus on tokens related to adsorbates, bulk composition, and their interacting atoms. Moreover, interacting atoms emerge as effective descriptors for adsorption configurations, while factors such as bond length and atomic properties of these atoms offer limited predictive contributions. By predicting adsorption energy from the textual representation of initial structures, CatBERTa achieves a mean absolute error (MAE) of 0.75 eV-comparable to vanilla Graph Neural Networks (GNNs). Furthermore, the subtraction of the CatBERTa-predicted energies effectively cancels out their systematic errors by as much as 19.3% for chemically similar systems, surpassing the error reduction observed in GNNs. This outcome highlights its potential to enhance the accuracy of energy difference predictions. This research establishes a fundamental framework for text-based catalyst property prediction, without relying on graph representations, while also unveiling intricate feature-property relationships.
更多查看译文
关键词
computational catalysis,catalyst screening,renewable energy,machine learning,Transformer,large language model
AI 理解论文
溯源树
样例
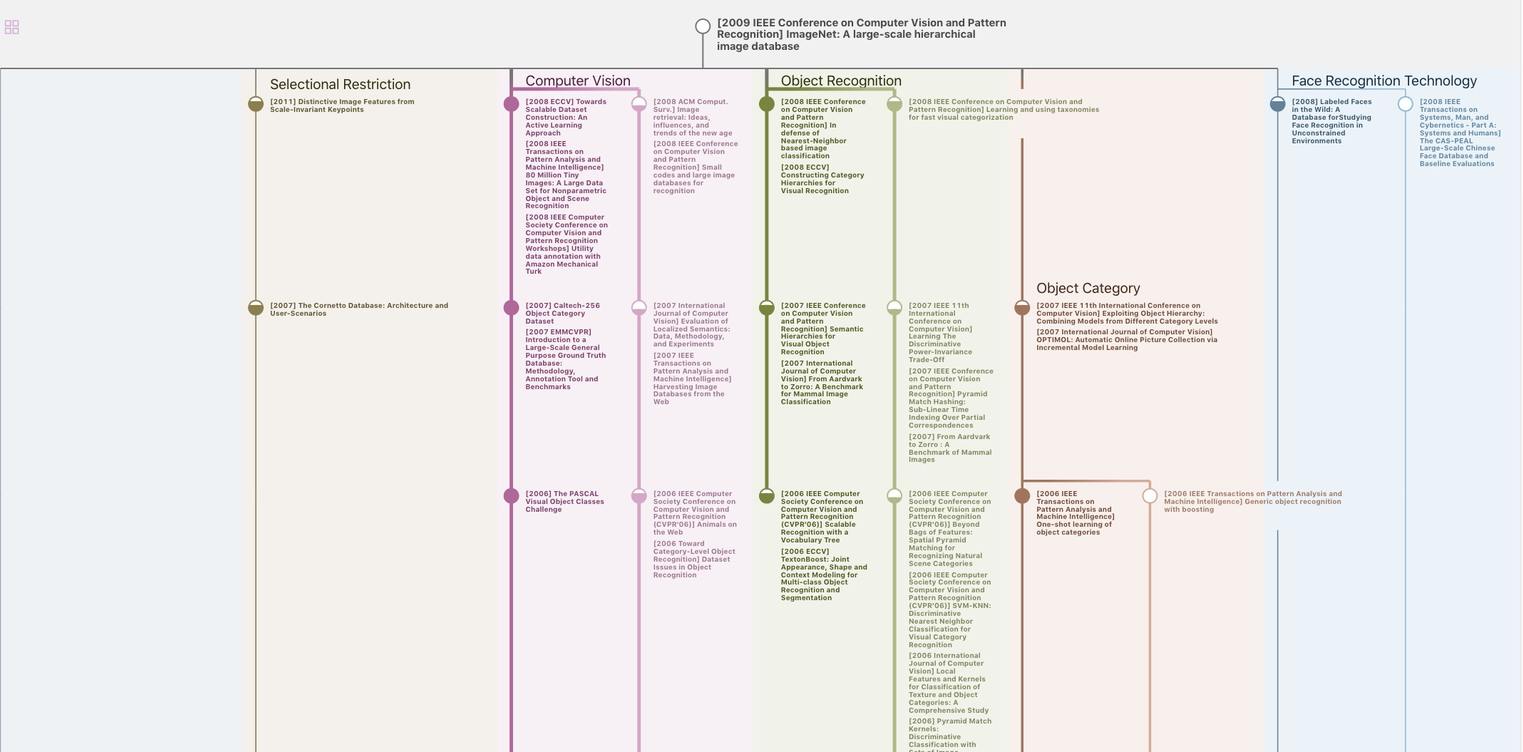
生成溯源树,研究论文发展脉络
Chat Paper
正在生成论文摘要