Magnetic Resonance Imaging Brain Tumor Segmentation Using Multiscale Ghost Generative Adversarial Network
Acta Photonica Sinica(2023)
摘要
Brain tumors are abnormal cells that grow in the brain or skull, and malignant brain tumors always cause great danger to the life and health of patients. Magnetic Resonance Imaging (MRI) can produce high-quality brain images without damage and skull artifacts, and it is currently one of the main technologies for the diagnosis and treatment of brain tumors. Meanwhile, the automatic segmentation of MRI brain tumor lesion regions is of great significance for the clinical diagnosis, surgical planning, and postoperative evaluation of brain tumor patients. However, due to the complexity and diversity of brain tumor images and the difficulty in obtaining the large-scale high-quality brain tumor segmentation dataset, it is still a difficult task to achieve high-precision automatic segmentation of MRI brain tumors. In recent years, with the breakthrough development of deep learning in computer vision tasks, it has also been successfully applied in the field of medical image analysis, and has achieved significant performance improvement in a number of medical image analysis tasks. Among them, U-Net, with its simple architecture and high performance, has become a mainstream model to solve a series of medical image segmentation tasks, including brain tumor segmentation. To this end, according to the advantages of the U-Net network architecture, focusing on issues of large variation of tumor spatial information and small number of finely labeled samples, a novel brain tumor image segmentation method, called Multi-scale Ghost Generation Adversarial Network (MG2AN), is proposed by combining U-Net with unsupervised generative adversarial network. MG2AN leverages the 3D U-Net model as a generator to obtain brain tumor segmentation results, and introduces 3D PatchGAN corresponding to multi-scale feature information of the generator as a discriminator to judge brain tumor segmentation results and ground truth, and the whole model is trained by adversarial learning. To improve the MRI brain tumor segmentation effect, a ghost module is introduced in the encoding stage of the generator, so that the ghost image and convolution feature map can be captured simultaneously during the convolution process, thus improving the brain tumor generation results of the generator. Considering the computational cost and the degree of information loss, the ghost module is only introduced in the final stage of the encoder. Meanwhile, multi-scale feature fusion is proposed in the decoding process to obtain three different brain tumor segmentation results for detail information, local information and global information, and then three kind of feature information for different emphasis are fused to further boost the segmentation performance in adversarial learning. To train the model, the loss of generated adversarial segmentation network is first back-propagated during the training process, and the new loss value is calculated based on the brain tumor segmentation results with the ground truth. Then, the two parts of the loss value are combined into the final loss value to jointly supervise the network. In addition, considering the obvious differences in grayscale and contrast of brain tumor images from different modalities, the Z-score method is utilized to normalize the image data in preprocessing. Meanwhile, to reduce the influence of large amount of useless background information in brain tumor images, the multi-modal 3D MRI brain tumor images are randomly cropped to a size of 128x128x128 as the input of the network. The data augmentation strategies such as random flip and intensity transformation are also adopted to guarantee the segmentation accuracy and generalizability of the model. The proposed MG2AN model is extensively evaluated on the public BraTS2019 and BraTS2020 brain tumor image datasets via ablation experiments, comparison experiments, and visualization results. The dice values of whole-tumor, core-tumor, and enhanced-tumor segmentation obtained by MG2AN on the BraTS2019 validation set are 0.902, 0.836, and 0.77, respectively. Meanwhile, the corresponding dice values on the BraTS2020 training and validation data sets are 0.902/0.903, 0.836/0.826 and 0.77/0.782, respectively. Compared with the baseline network, the dice values of MG2AN on the BraTS2020 training and validation data sets increase by 4.1%/1.3%, 2.2%/0.9% and 5.7%/2.7% on the whole tumor, core tumor and enhanced tumor, respectively, demonstrating the effectiveness of introducing generative adversarial networks and the improved generators. When comparing MG2AN with the state-of-the-art methods in the field of brain tumor segmentation, it can show comparable or better performance by considering both dice and Hausdorff 95 evaluation results. Finally, it also can be carried out that the segmentation effectiveness of MG2AN model is better than the baseline model in the visual evaluation of brain tumor segmentation. Therefore, the comprehensive experimental evaluation and analysis results demonstrate the effectiveness of the proposed MG2AN for the brain tumor segmentation task.
更多查看译文
关键词
Brain tumor segmentation,3D U-Net,Generative adversarial network,Ghost feature,Multi-scale feature fusion
AI 理解论文
溯源树
样例
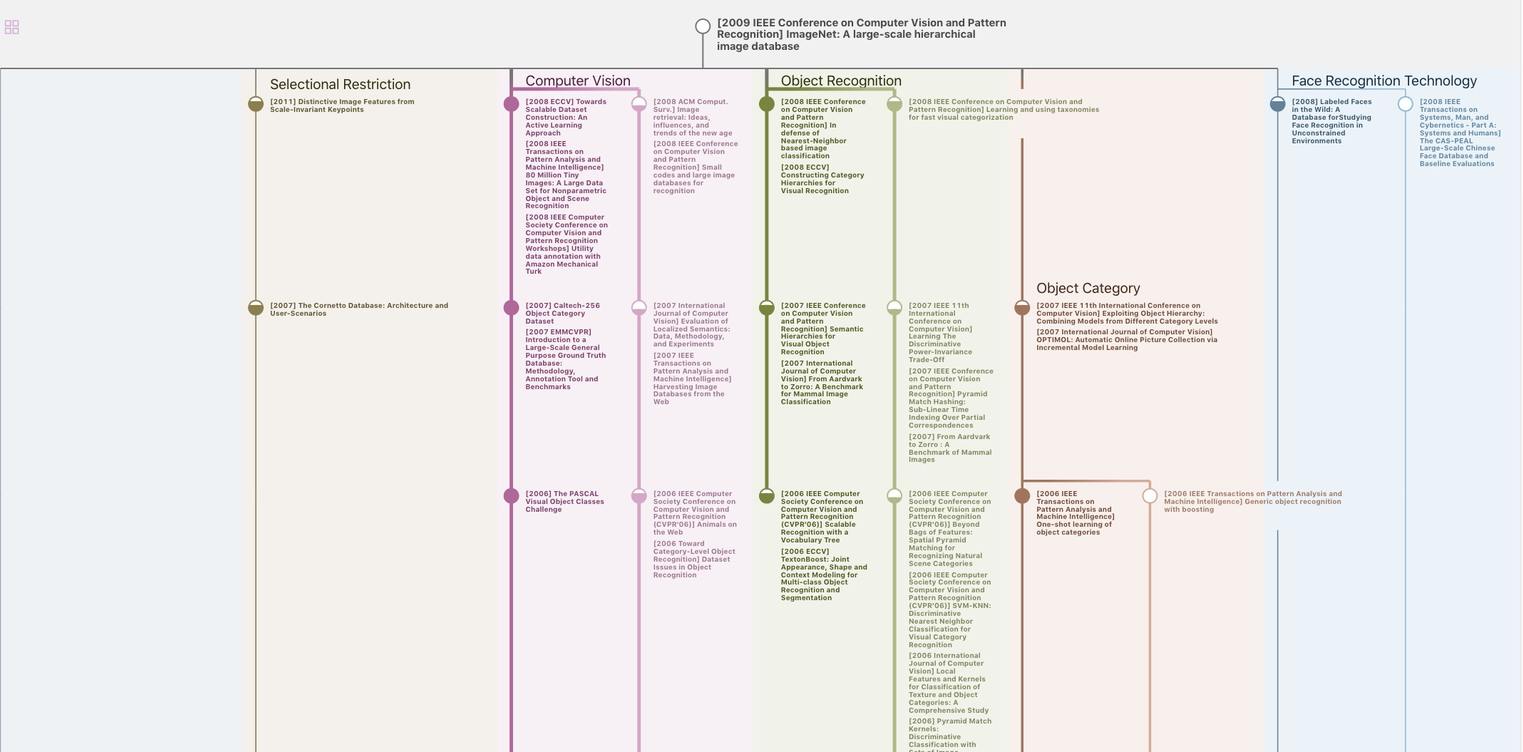
生成溯源树,研究论文发展脉络
Chat Paper
正在生成论文摘要