A Neural Network Driven Approach for Characterizing the Interplay Between Short Range Ordering and Enthalpy of Mixing of Binary Subsystems in the NbTiVZr High Entropy Alloy
Journal of Phase Equilibria and Diffusion(2023)
摘要
Recently high entropy alloys (HEA) have shown remarkable potential due to their extraordinary properties and applications. HEAs are explored to create a new class of materials with an attractive set of properties that are difficult to achieve by conventional materials. Short-range ordering (SRO) is important in determining various materials properties at nanometer scale, such as phase stability. The relationship between SRO and phase stability can be understood through the enthalpy of mixing. Cluster expansion (CE) is often used to understand the relationship between the enthalpy of mixing and SRO parameters. Although exact, CE must be truncated in practice beyond some maximal-sized cluster, leading to truncation errors. In this work, as an alternative, a neural network is trained to understand the relationship between SRO and enthalpy of mixing among the various binary subsystems of NbTiVZr HEA. For training, a large pool of structures and their corresponding correlation functions (or SRO parameters) are generated using the alloy theoretic automated toolkit (ATAT) software for each subsystem. First-principles calculations are used to determine the enthalpy of mixing of these structures. This database is used to train a neural network and the predicted values of enthalpy of mixing from the trained neural network are found to be reasonably accurate and better than the corresponding CE model. The neural network approach is found to clarify the complex relationship between the enthalpy of mixing and SRO, especially when there is a limitation over the number of fitting parameters due to smaller size of databases.
更多查看译文
关键词
cluster expansion,convex hull,data correlation,database,first-principles calculations
AI 理解论文
溯源树
样例
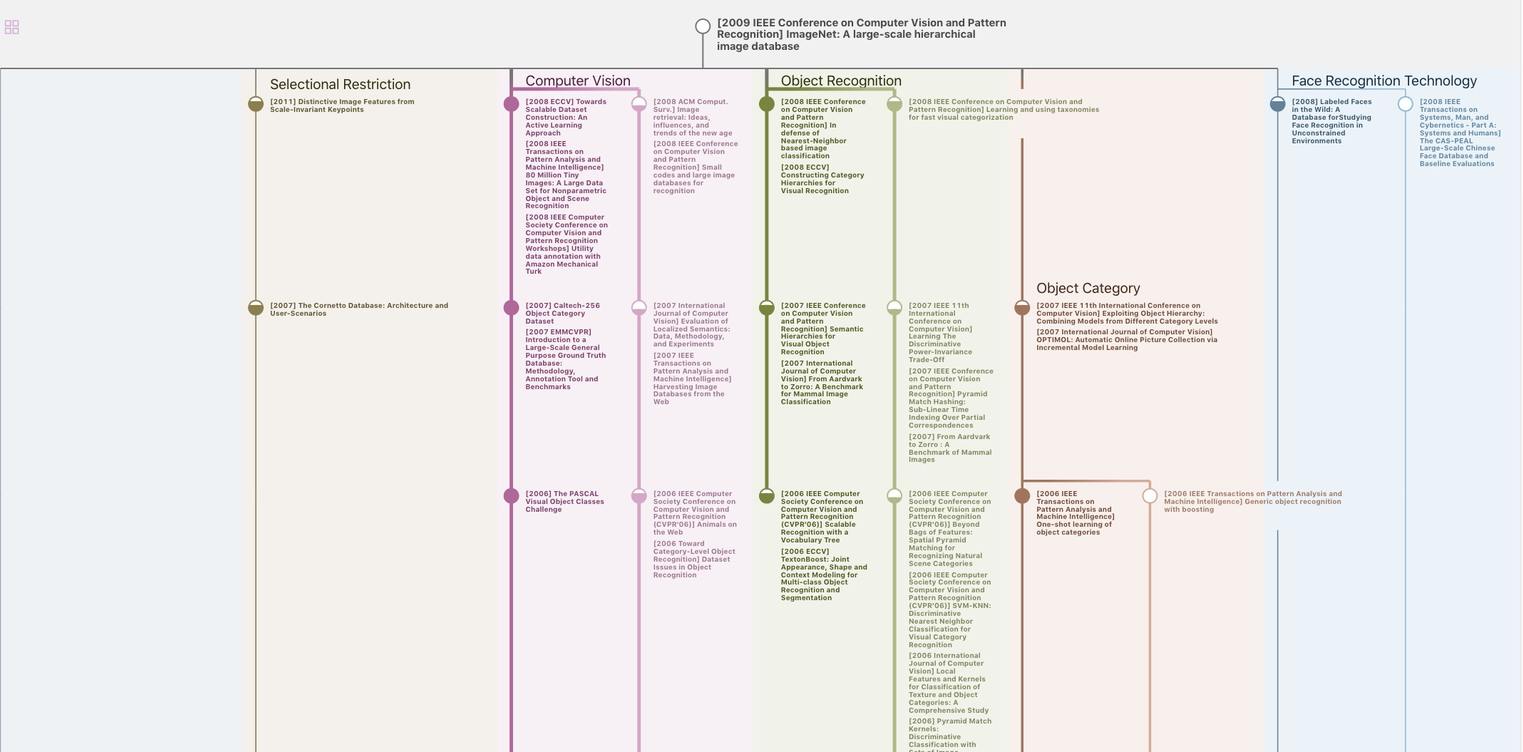
生成溯源树,研究论文发展脉络
Chat Paper
正在生成论文摘要