Variable screening for Lasso based on multidimensional indexing
DATA MINING AND KNOWLEDGE DISCOVERY(2023)
摘要
In this paper we present a correlation based safe screening technique for building the complete Lasso path. Unlike many other Lasso screening approaches we do not consider prespecified values of the regularization parameter, but, instead, prune variables which cannot be the next best feature to be added to the model. Based on those results we present a modified homotopy algorithm for computing the regularization path. We demonstrate that, even though our algorithm provides the complete Lasso path, its performance is competitive with state of the art algorithms which, however, only provide solutions at a prespecified sample of regularization parameters. We also address problems of extremely high dimensionality, where the variables may not fit into main memory and are assumed to be stored on disk. A multidimensional index is used to quickly retrieve potentially relevant variables. We apply the approach to the important case when multiple models are built against a fixed set of variables, frequently encountered in statistical databases. We perform experiments using the complete Eurostat database as predictors and demonstrate that our approach allows for practical and efficient construction of Lasso models, which remain accurate and interpretable even when millions of highly correlated predictors are present.
更多查看译文
关键词
Variable screening,Lasso,Linear regression,Open data
AI 理解论文
溯源树
样例
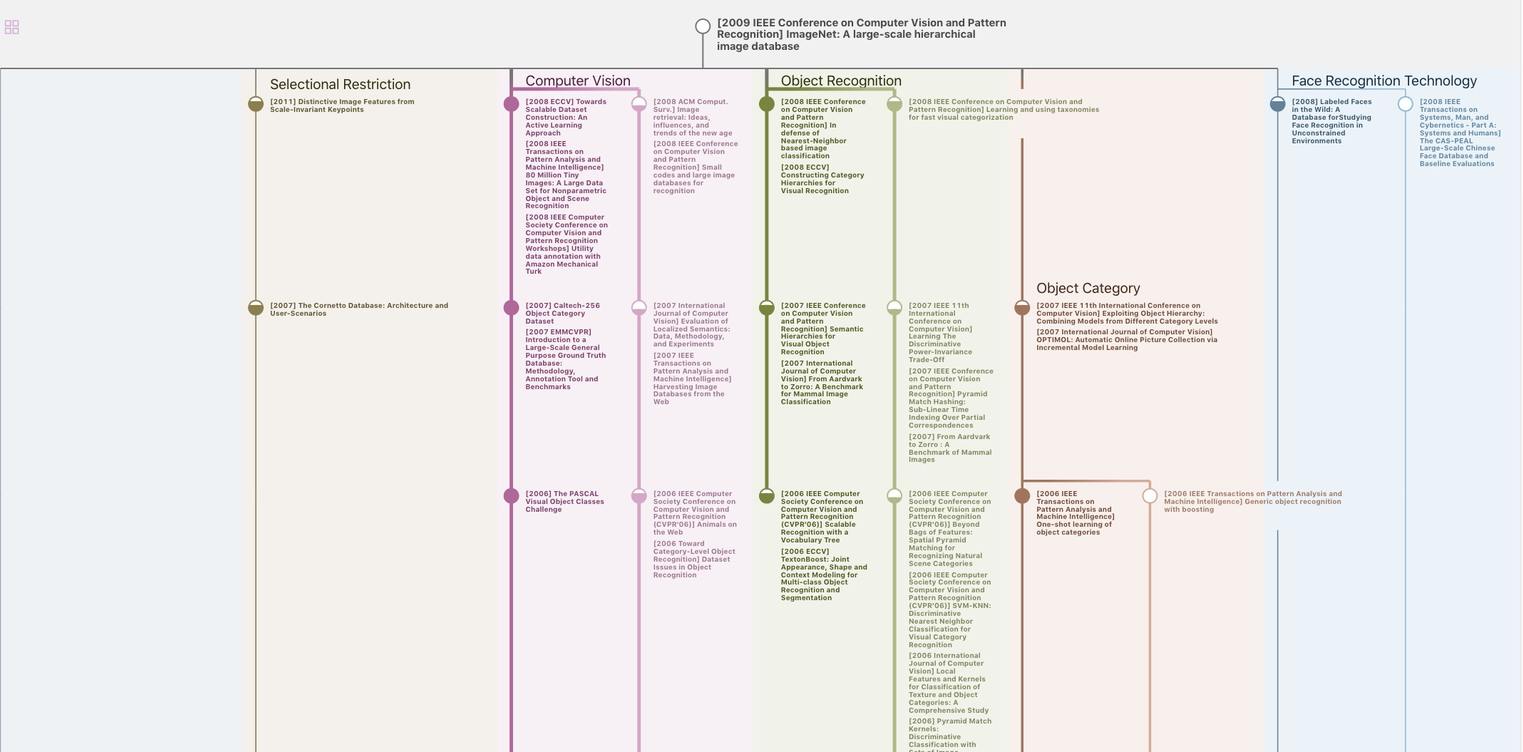
生成溯源树,研究论文发展脉络
Chat Paper
正在生成论文摘要