Improving graph collaborative filtering with multimodal-side-information-enriched contrastive learning
Journal of Intelligent Information Systems(2024)
摘要
The multimodal side information such as images and text have been commonly used as supplements to improve graph collaborative filtering recommendations. However, there is often a semantic gap between multimodal information and collaborative filtering information. Previous works often directly fuse or align these information, which results in semantic distortion or degradation. Additionally, multimodal information also introduces additional noises, and previous methods lack explicit supervision to identify these noises. To tackle the issues, we propose a novel contrastive learning approach to improve graph collaborative filtering, named M ultimodal- S ide- I nformation-enriched C ontrastive L earning ( MSICL ), which does not fuse multimodal information directly, but still explicitly captures users’ potential preferences for similar images or text by contrasting ID embeddings, and filters noises in multimodal side information. Specifically, we first search for samples with similar images or text as positive contrastive pairs. Secondly, some searched sample pairs may be irrelevant, so we distinguish the noise by filtering out sample pairs that have no interaction relationship. Thirdly, we contrast the ID embeddings of the true positive sample pairs to excavate the potential similarity relationship in multimodal side information. Extensive experiments on three datasets demonstrate the superiority of our method in multimodal recommendation. Moreover, our approach significantly reduces computation and memory cost compared to previous work.
更多查看译文
关键词
Recommender systems,Collaborative filtering,Graph neural network,Multimodal recommendation,Contrastive learning
AI 理解论文
溯源树
样例
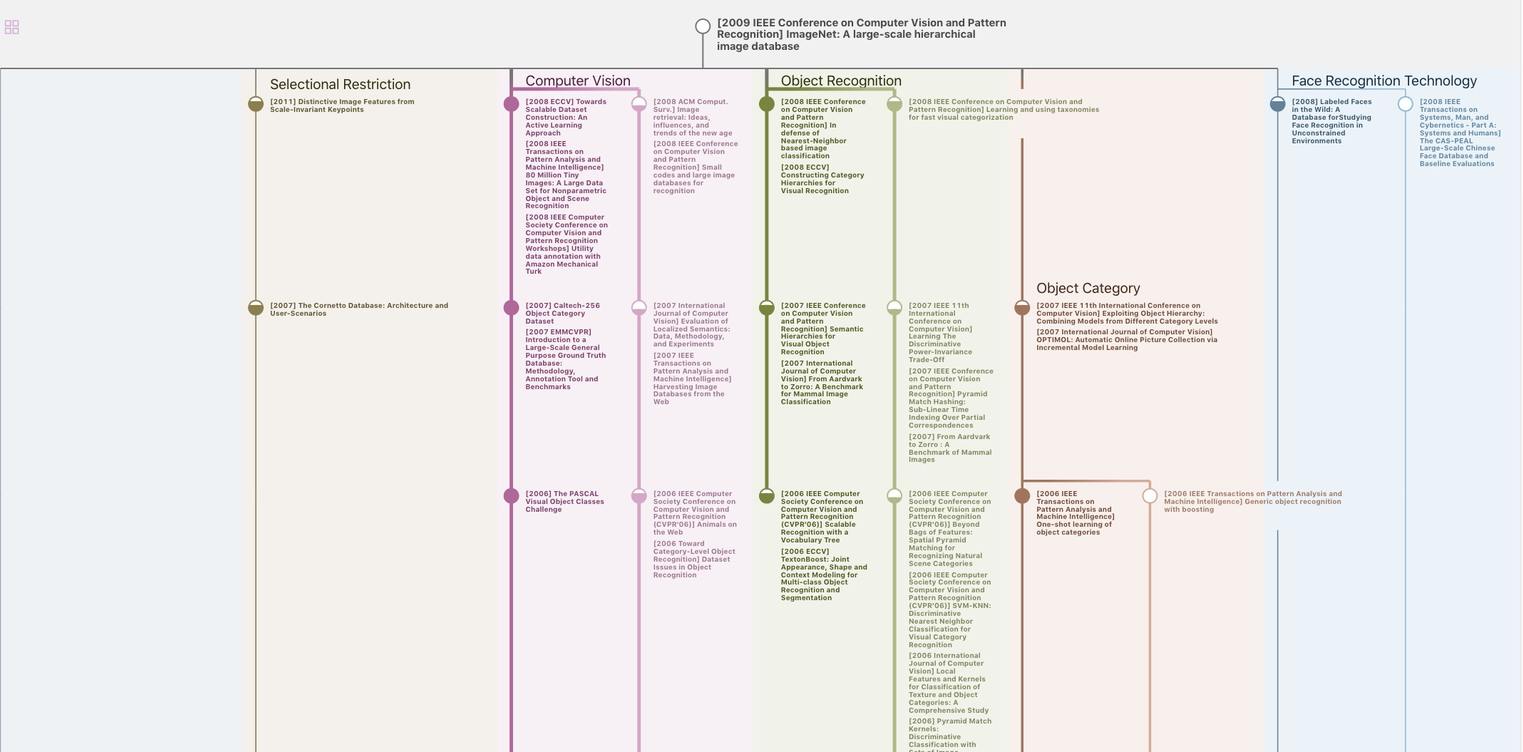
生成溯源树,研究论文发展脉络
Chat Paper
正在生成论文摘要