Why do residents fail simulation-based assessments of operative competence? A qualitative analysis
Global Surgical Education - Journal of the Association for Surgical Education(2023)
摘要
This qualitative study explored factors influencing the scoring decisions of raters in a simulation-based assessment of operative competence, by analysing feedback provided to trainees receiving ‘borderline’ or ‘not competent’ global scores across stations in an Objective Structured Clinical Examination (OSCE). Directed qualitative content analysis was conducted on feedback provided to trainees during a novel simulation-based assessment. Initial codes were derived from the domains of the Objective Structured Assessment of Technical Skills (OSATS) tool. Further quantitative analysis was conducted to compare the frequency of codes across feedback provided to ‘junior’ and ‘senior’ general surgery trainees. Thirteen trainees undertook the eight-station OSCE and were observed by ten trained assessors. Seven of these were ‘senior’ trainees in their last 4 years of surgical training, while 6 were ‘junior’ trainees in their first 4 years. A total of 130 individual observations were recorded. Written feedback was available for 44 of the 51 observations scored as ‘borderline’ or ‘not competent’. On content analysis, ‘knowledge of the specific procedure’ was the most commonly cited reason for failure, while ‘judgement’ and ‘the model as a confounder’ were two newly generated categories found to contribute to scoring decisions. The OSATS tool can capture a majority of reasons cited for ‘borderline’ or ‘not competent’ performance. Deficiencies in ‘Judgement’ may require simultaneous non-technical skill assessments to adequately capture. It is imperative that assessors and candidates are adequately familiarised with models prior to assessment, to limit the potential impact of model unfamiliarity as a confounder.
更多查看译文
关键词
Assessment, Surgical training, Operative skill, Performance of procedures
AI 理解论文
溯源树
样例
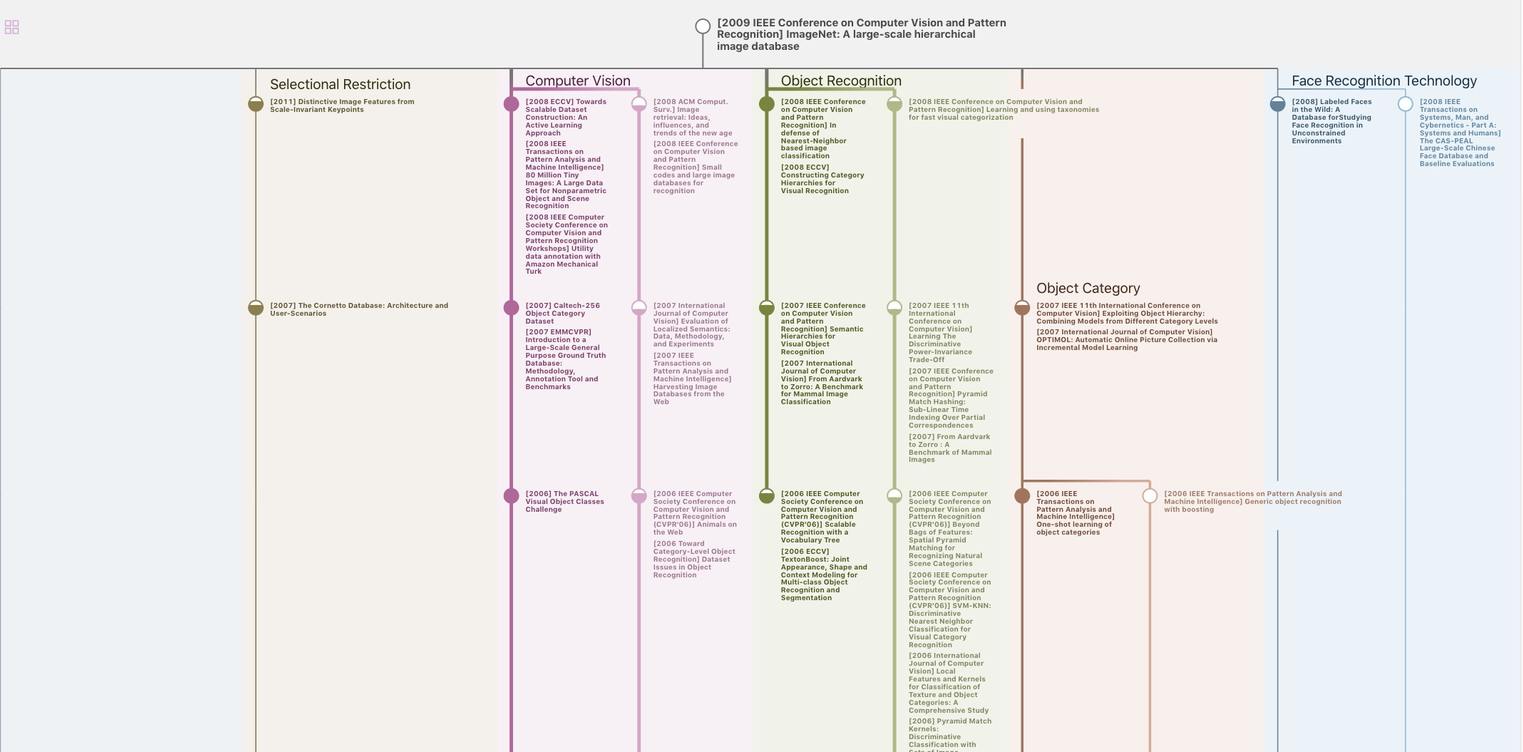
生成溯源树,研究论文发展脉络
Chat Paper
正在生成论文摘要