Indirect Inference and Small Sample Bias — Some Recent Results
OPEN ECONOMIES REVIEW(2023)
摘要
Macroeconomic researchers use a variety of estimators to parameterise their models empirically. One such is FIML; another is indirect inference (II). One form of indirect inference is ‘informal’ whereby data features are ‘targeted’ by the model — i.e. parameters are chosen so that model-simulated features replicate the data features closely. Monte Carlo experiments show that in the small samples prevalent in macro data, both FIML informal II produce high bias, while formal II, in which the joint probability of the data- generated auxiliary model is maximised under the model simulated distribution, produces low bias. They also show that FII gets this low bias from its high power in rejecting misspecified models, which comes in turn from the fact that this distribution is restricted by the model-specified parameters, so sharply distinguishing it from rival misspecified models.
更多查看译文
关键词
Moments,Indirect inference
AI 理解论文
溯源树
样例
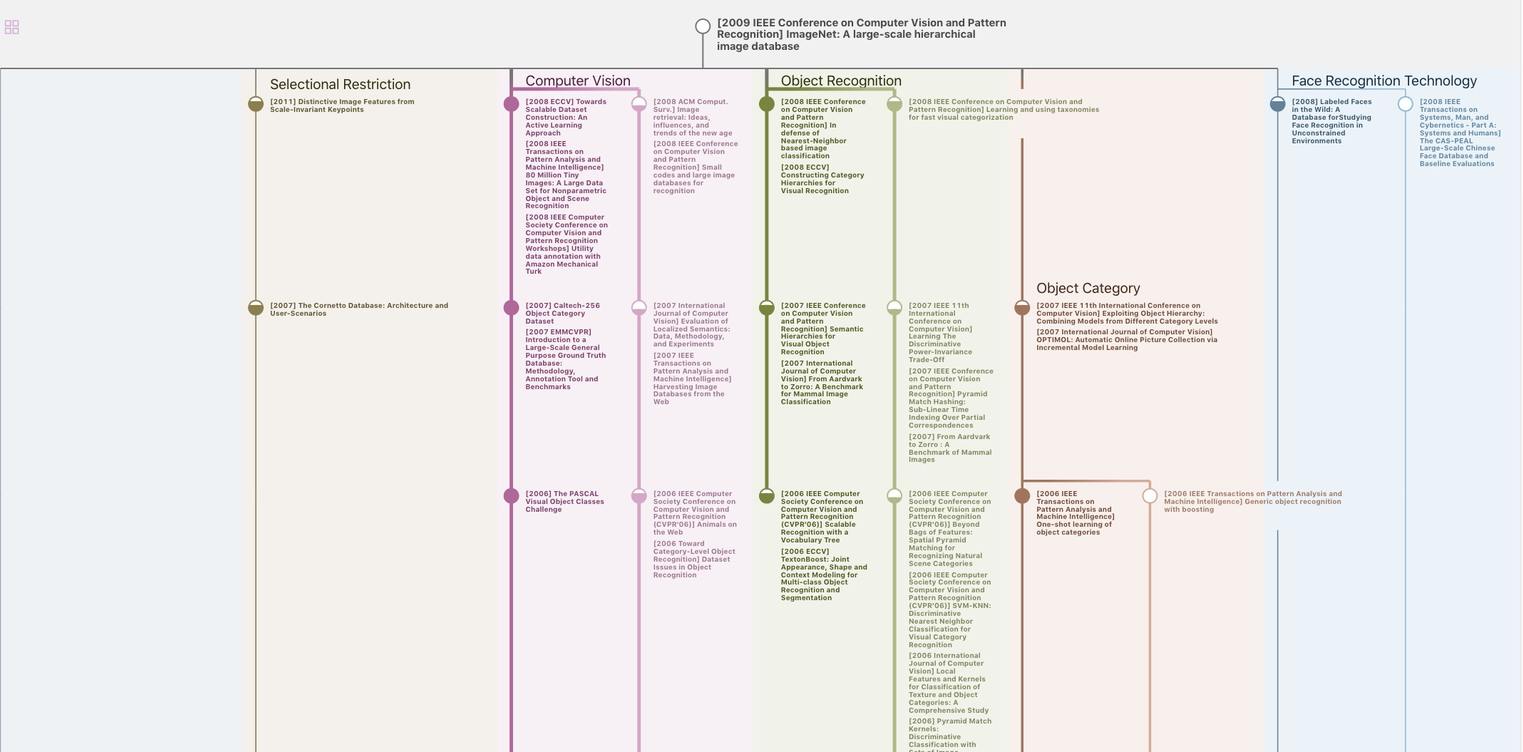
生成溯源树,研究论文发展脉络
Chat Paper
正在生成论文摘要