An improved U-Net-based network for multiclass segmentation and category ratio statistics of ore images
Soft Computing(2024)
摘要
The ratio of various types of ores on a conveyor belt indicates how well the ores are blended, which can play a crucial role in determining the quality of steel. However, the conventional method of artificial naked eye detection based on experience is risky, time-consuming, and inaccurate. To address this issue, this study proposes an automatic ore category percentage statistics method based on multiclass semantic segmentation. The first step involves creating a clean dataset comprising five different categories of ores collected at a mine site. Subsequently, a novel U-Net-based network is introduced to segment the ore images. Specifically, a pre-trained VGG16 is adopted to replace the original backbone to enhance the feature extraction ability. A pyramid pooling module is also simplified to effectively aggregate the multiscale information. In addition, a W-shaped architecture, which bridges feature maps between the first and second encoders through addition, is utilized to generate the final probability map in a predict-refine manner. The experimental results demonstrate that the proposed model achieves a pixel-wise accuracy of 92.45% and a mean intersection over union of 72.42%, outperforming ten other state-of-the-art segmentation algorithms. Furthermore, the proposed method is applied to predict images of unprocessed ores from a mine site to determine their category proportions, thus providing valuable guidance for optimizing the ore blending process.
更多查看译文
关键词
Ore category ratio statistics,Multiclass image segmentation,Transfer learning,Multiscale,W-shaped architecture,Bridging method
AI 理解论文
溯源树
样例
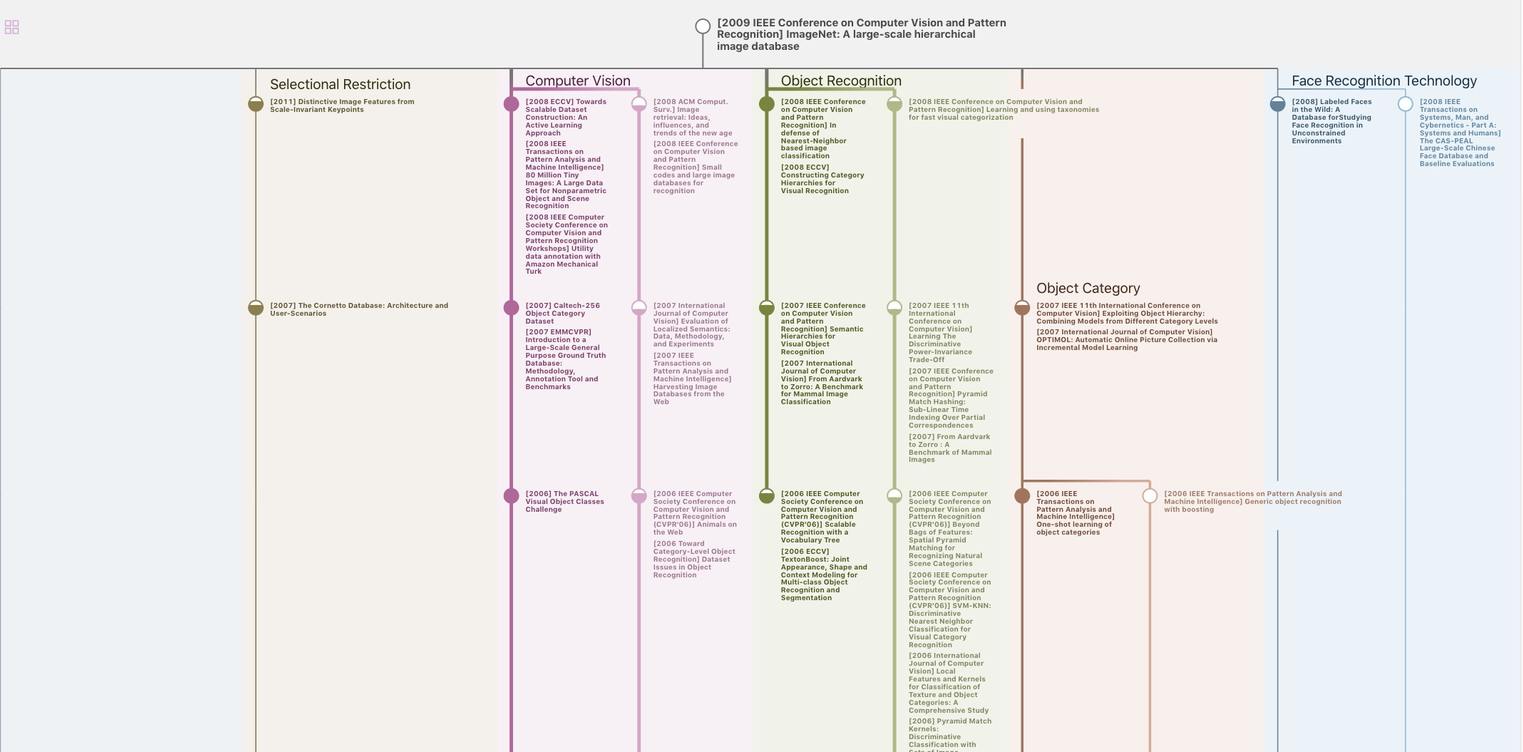
生成溯源树,研究论文发展脉络
Chat Paper
正在生成论文摘要