Machine-learning potentials for nanoscale simulations of deformation and fracture: example of TiB$_2$ ceramic
arXiv (Cornell University)(2023)
摘要
Machine-learning interatomic potentials (MLIPs) offer a powerful avenue for simulations beyond length and timescales of ab initio methods. Their development for investigation of mechanical properties and fracture, however, is far from trivial since extended defects -- governing plasticity and crack nucleation in most materials -- are too large to be included in the training set. Using TiB$_2$ as a model ceramic material, we propose a strategy for fitting MLIPs suitable to simulate mechanical response of monocrystals until fracture. Our MLIP accurately reproduces ab initio stresses and failure mechanisms during room-temperature uniaxial tensile deformation of TiB$_2$ at the atomic scale ($\approx{10}^3$ atoms). More realistic tensile tests (low strain rate, Poisson's contraction) at the nanoscale ($\approx{10}^4$--10$^6$ atoms) require MLIP up-fitting, i.e. learning from additional ab initio configurations. Consequently, we elucidate trends in theoretical strength, toughness, and crack initiation patterns under different loading directions. To identify useful environments for further up-fitting, i.e., making the MLIP applicable to a wider spectrum of simulations, we asses transferability to other deformation conditions and phases not explicitly trained on.
更多查看译文
关键词
nanoscale simulations,tib2,ceramic,machine-learning machine-learning,deformation
AI 理解论文
溯源树
样例
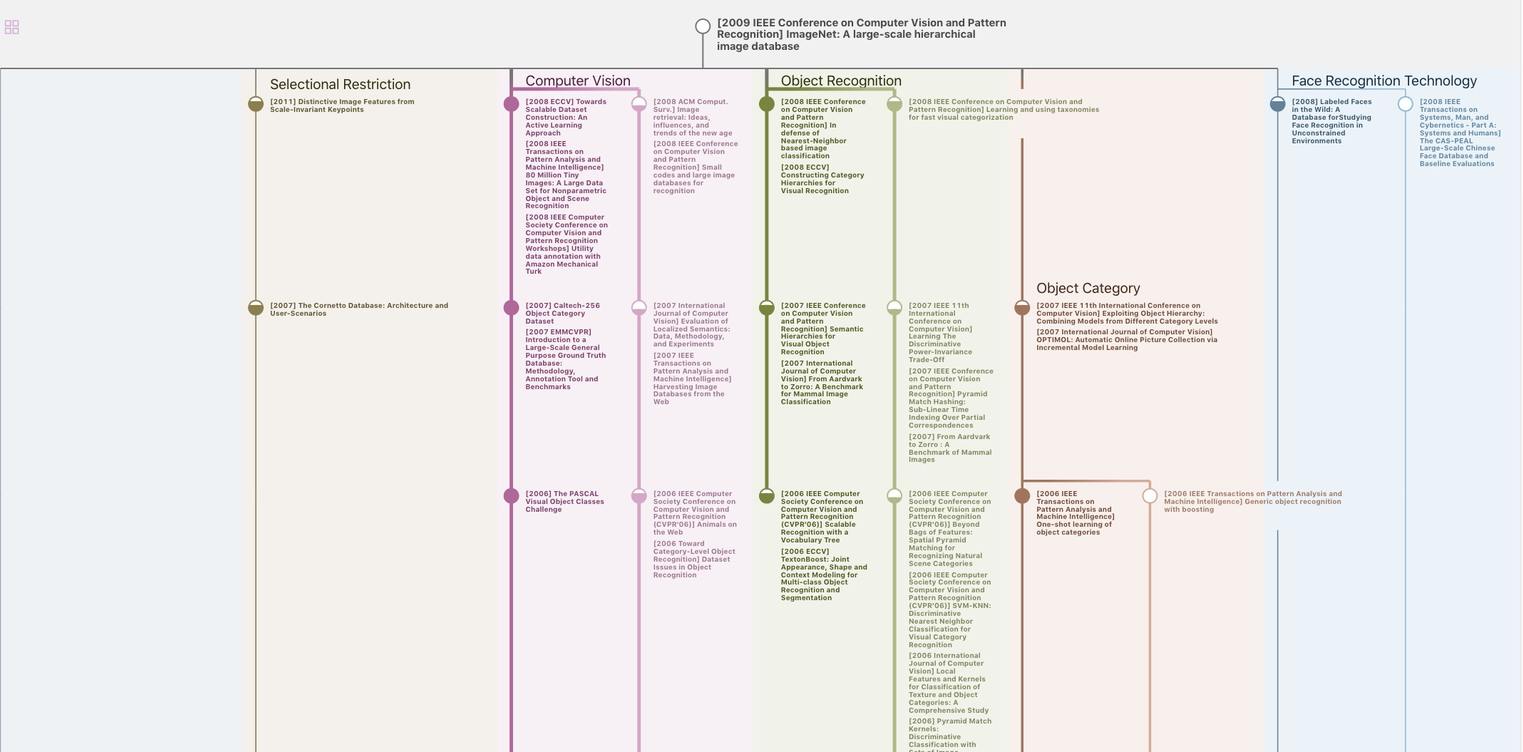
生成溯源树,研究论文发展脉络
Chat Paper
正在生成论文摘要