Cognition-Mode Aware Variational Representation Learning Framework for Knowledge Tracing
23RD IEEE INTERNATIONAL CONFERENCE ON DATA MINING, ICDM 2023(2023)
摘要
The Knowledge Tracing (KT) task plays a crucial role in personalized learning, and its purpose is to predict student responses based on their historical practice behavior sequence. However, the KT task suffers from data sparsity, which makes it challenging to learn robust representations for students with few practice records and increases the risk of model overfilling. Therefore, in this paper, we propose a Cognition -Mode Aware Variational Representation Learning Framework (CMVF) that can be directly applied to existing KT methods. Our framework uses a probabilistic model to generate a distribution for each student, accounting for uncertainty in those with limited practice records, and estimate the student's distribution via variational inference (VI). In addition, we also introduce a cognition mode aware multinomial distribution as prior knowledge that constrains the posterior student. distributions learning, so as to ensure that students with similar cognition modes have similar distributions, avoiding overwhelming personalization for students with few practice records. At last, extensive experimental results confirm that CMVF can effectively aid existing KT methods in learning more robust student representations. Our code is available at littps://github.comizmy-9/CMVF.
更多查看译文
关键词
Representation Learning,Knowledge Tracing,Variational Inference,Educational Data Milling
AI 理解论文
溯源树
样例
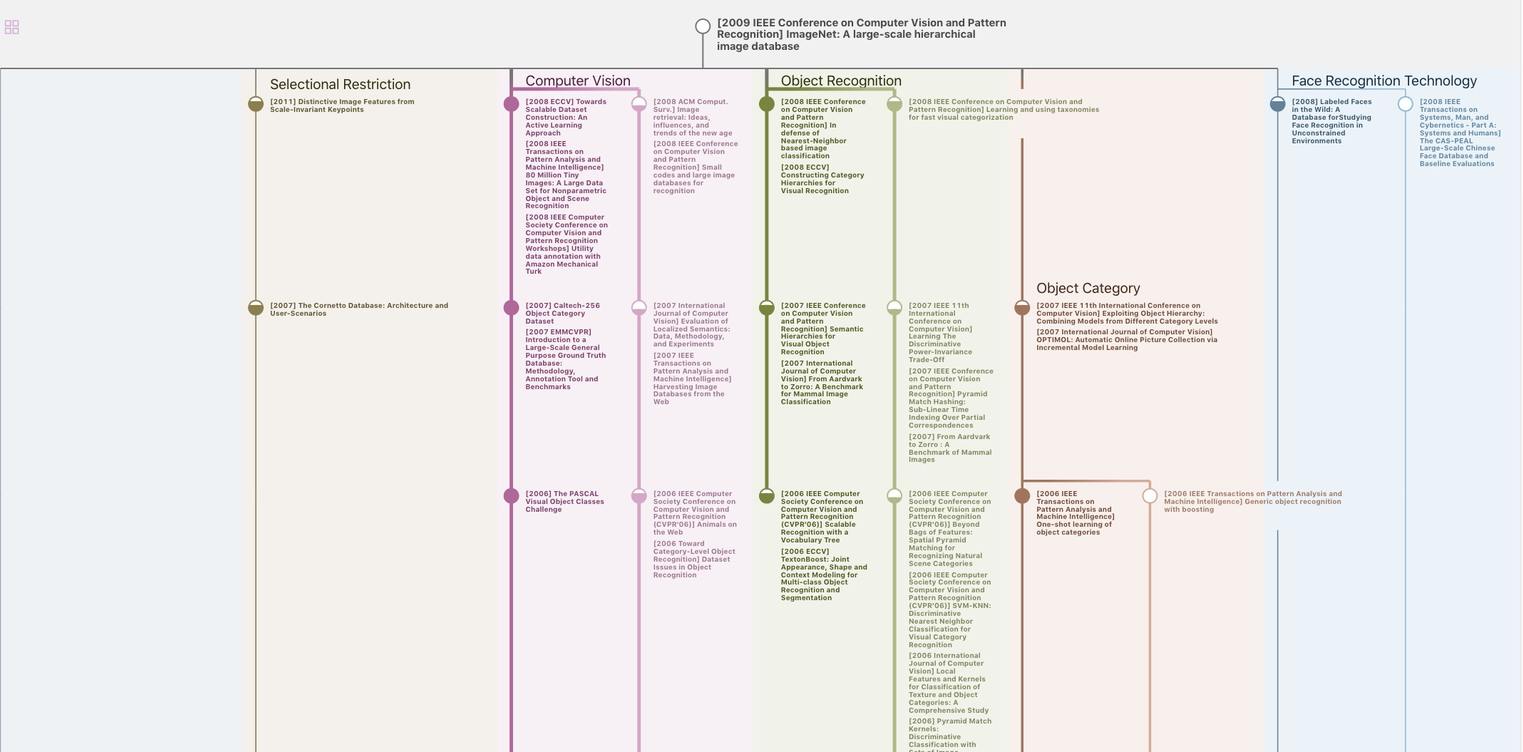
生成溯源树,研究论文发展脉络
Chat Paper
正在生成论文摘要