On the numerical approximation of the distance to singularity for matrix-valued functions
CoRR(2023)
摘要
Given a matrix-valued function $\mathcal{F}(\lambda)=\sum_{i=1}^d f_i(\lambda) A_i$, with complex matrices $A_i$ and $f_i(\lambda)$ analytic functions for $i=1,\ldots,d$, we discuss a method for the numerical approximation of the distance to singularity for $\mathcal{F}(\lambda)$. The closest matrix-valued function $\widetilde {\mathcal{F}}(\lambda)$ with respect to the Frobenius norm is approximated using an iterative method. The condition of singularity on the matrix-valued function is translated into a numerical constraint for a suitable minimization problem. Unlike the case of matrix polynomials, in the general setting of matrix-valued functions the main issue is that the function $\det ( \widetilde{\mathcal{F}}(\lambda) )$ may have an infinite number of roots. The main feature of the numerical method consists in the possibility of extending it to different structures, such as sparsity patterns induced by the matrix coefficients.
更多查看译文
关键词
numerical approximation,singularity,matrix-valued
AI 理解论文
溯源树
样例
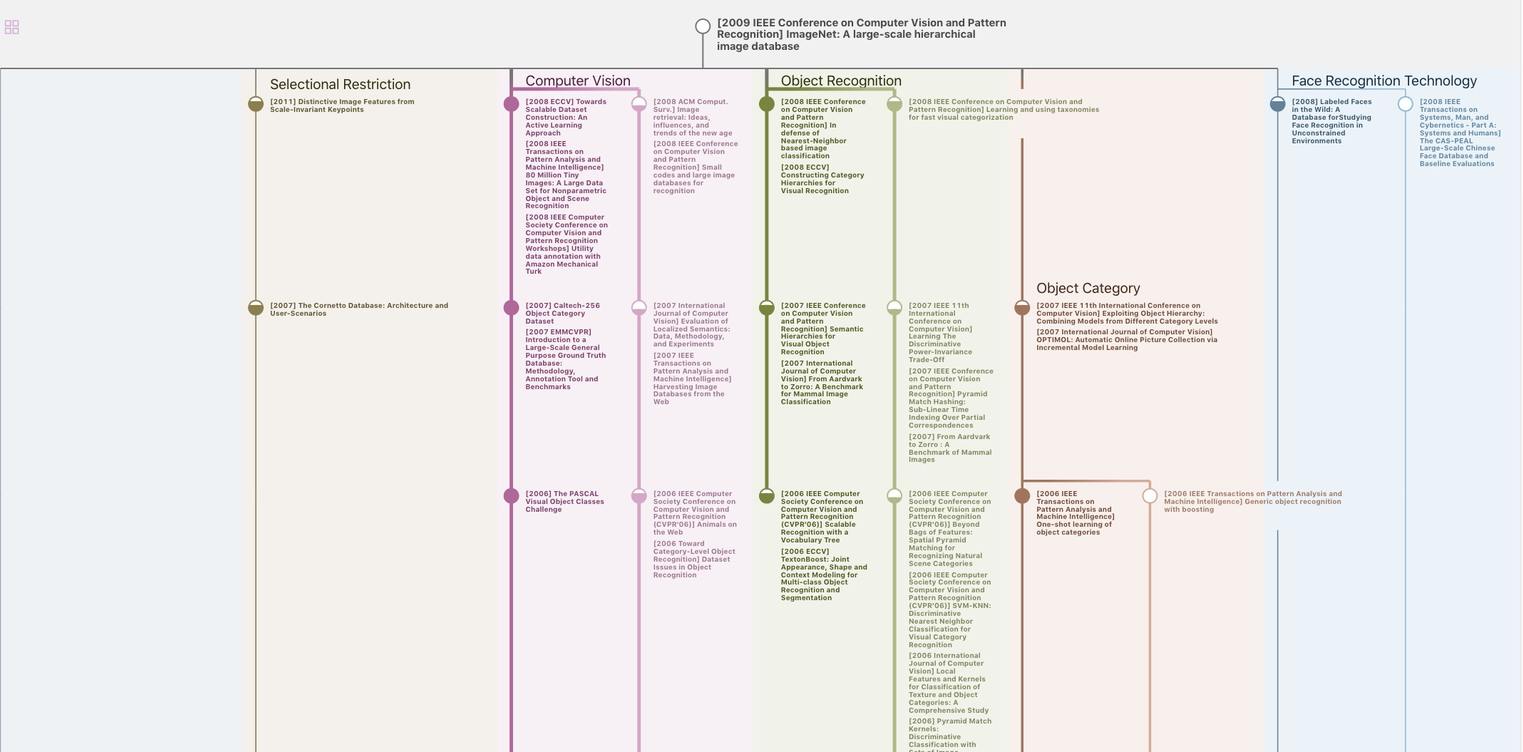
生成溯源树,研究论文发展脉络
Chat Paper
正在生成论文摘要