How Crowd Worker Factors Influence Subjective Annotations: A Study of Tagging Misogynistic Hate Speech in Tweets
CoRR(2023)
摘要
Crowdsourced annotation is vital to both collecting labelled data to train and test automated content moderation systems and to support human-in-the-loop review of system decisions. However, annotation tasks such as judging hate speech are subjective and thus highly sensitive to biases stemming from annotator beliefs, characteristics and demographics. We conduct two crowdsourcing studies on Mechanical Turk to examine annotator bias in labelling sexist and misogynistic hate speech. Results from 109 annotators show that annotator political inclination, moral integrity, personality traits, and sexist attitudes significantly impact annotation accuracy and the tendency to tag content as hate speech. In addition, semi-structured interviews with nine crowd workers provide further insights regarding the influence of subjectivity on annotations. In exploring how workers interpret a task - shaped by complex negotiations between platform structures, task instructions, subjective motivations, and external contextual factors - we see annotations not only impacted by worker factors but also simultaneously shaped by the structures under which they labour.
更多查看译文
关键词
tagging misogynistic hate speech,hate speech,tweets
AI 理解论文
溯源树
样例
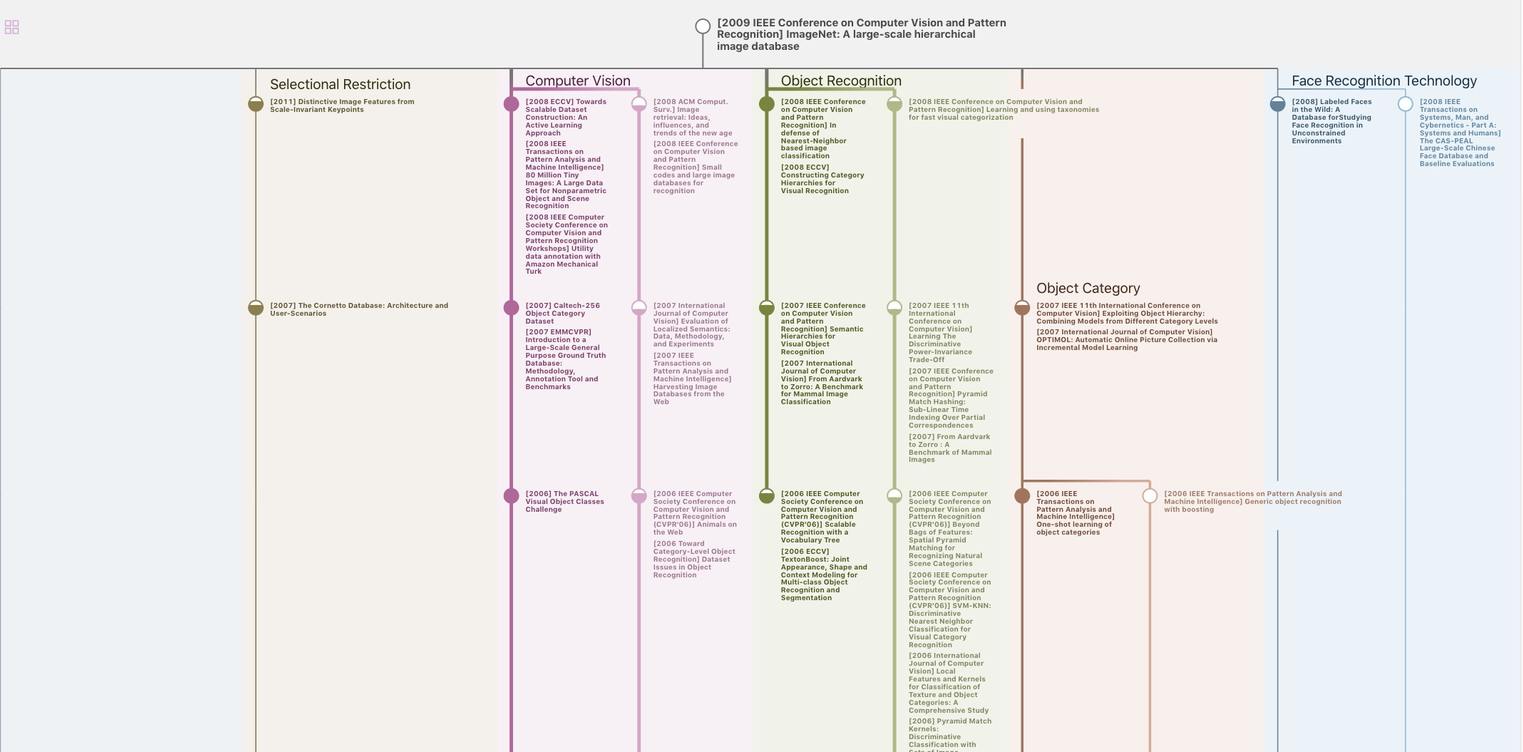
生成溯源树,研究论文发展脉络
Chat Paper
正在生成论文摘要