Image denoising in photon-counting CT using PFGM++ with hijacked regularized sampling
arXiv (Cornell University)(2023)
摘要
Deep learning (DL) has proven to be an important tool for high quality image denoising in low-dose and photon-counting CT. However, DL models are usually trained using supervised methods, requiring paired data that may be difficult to obtain in practice. Physics-inspired generative models, such as score-based diffusion models, offer unsupervised means of solving a wide range of inverse problems via posterior sampling. The latest in this family are Poisson flow generative models (PFGM)++ which, inspired by electrostatics, treat the $N$-dimensional data as positive electric charges in a $N+D$-dimensional augmented space. The electric field lines generated by these charges are used to find an invertible mapping, via an ordinary differential equation, between an easy-to-sample prior and the data distribution of interest. In this work, we propose a method for CT image denoising based on PFGM++ that does not require paired training data. To achieve this, we adapt PFGM++ for solving inverse problems via posterior sampling, by hijacking and regularizing the sampling process. Our method incorporates score-based diffusion models (EDM) as a special case as $D\rightarrow \infty$, but additionally allows trading off robustness for rigidity by varying $D$. The network is efficiently trained on randomly extracted patches from clinical normal-dose CT images. The proposed method demonstrates promising performance on clinical low-dose CT images and clinical images from a prototype photon-counting system.
更多查看译文
关键词
sampling,photon-counting
AI 理解论文
溯源树
样例
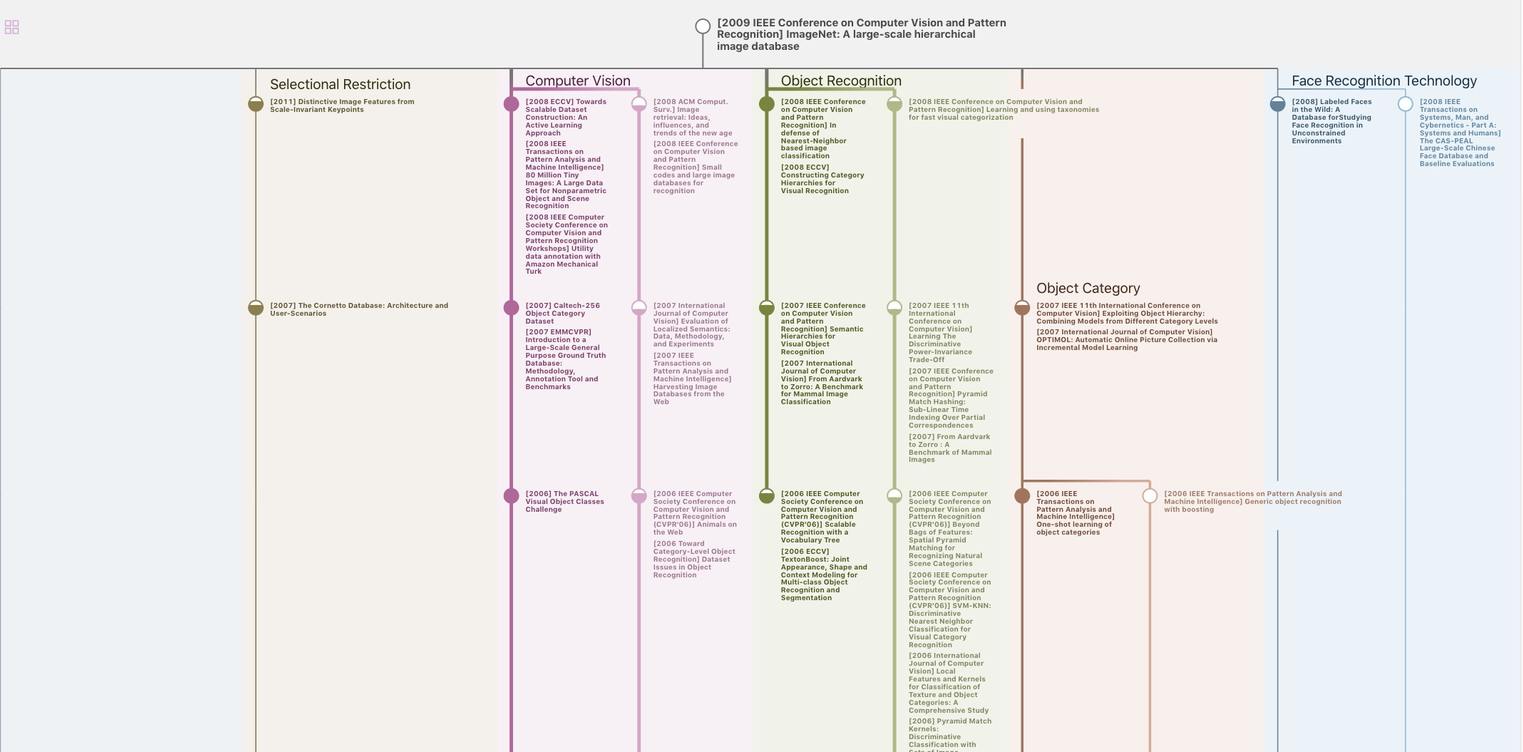
生成溯源树,研究论文发展脉络
Chat Paper
正在生成论文摘要