Supervised dimensionality reduction for multiple imputation by chained equations
arXiv (Cornell University)(2023)
摘要
Multivariate imputation by chained equations (MICE) is one of the most popular approaches to address missing values in a data set. This approach requires specifying a univariate imputation model for every variable under imputation. The specification of which predictors should be included in these univariate imputation models can be a daunting task. Principal component analysis (PCA) can simplify this process by replacing all of the potential imputation model predictors with a few components summarizing their variance. In this article, we extend the use of PCA with MICE to include a supervised aspect whereby information from the variables under imputation is incorporated into the principal component estimation. We conducted an extensive simulation study to assess the statistical properties of MICE with different versions of supervised dimensionality reduction and we compared them with the use of classical unsupervised PCA as a simpler dimensionality reduction technique.
更多查看译文
关键词
multiple imputation,supervised dimensionality reduction,equations
AI 理解论文
溯源树
样例
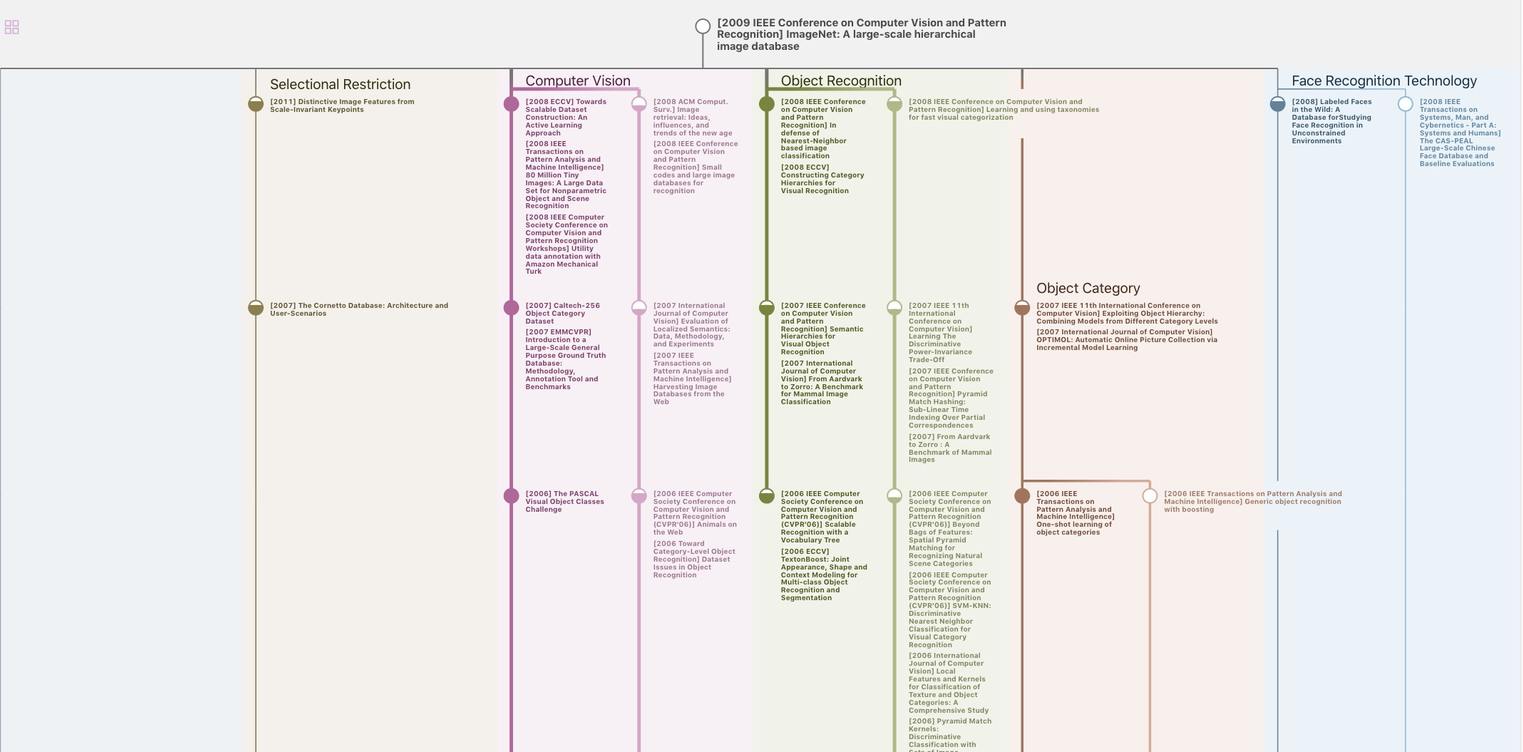
生成溯源树,研究论文发展脉络
Chat Paper
正在生成论文摘要