Impact of Bayesian Priors on the Inferred Masses of Quasi-Circular Intermediate-Mass Black Hole Binaries
arXiv (Cornell University)(2023)
摘要
Observation of gravitational waves from inspiralling binary black holes has offered a unique opportunity to study the physical parameters of the component black holes. To infer these parameters, Bayesian methods are employed in conjunction with general relativistic waveform models that describe the source's inspiral, merger, and ringdown. The results depend not only on the accuracy of the waveform models but also on the underlying fiducial prior distribution used for the analysis. In particular, when the pre-merger phase of the signal is barely observable within the detectors' bandwidth, as is currently the case with intermediate-mass black hole binary signals in ground-based gravitational wave detectors, different prior assumptions can lead to different interpretations. In this study, we utilise the gravitational-wave inference library, $\texttt{Parallel Bilby}$, to evaluate the impact of mass prior choices on the parameter estimation of intermediate-mass black hole binary signals. While previous studies focused primarily on analysing event data, we offer a broader, more controlled study by using simulations. Our findings suggest that the posteriors in total mass, mass ratio and luminosity distance are contingent on the assumed mass prior distribution used during the inference process. This is especially true when the signal lacks sufficient pre-merger information and/or has inadequate power in the higher-order radiation multipoles. In conclusion, our study underscores the importance of thoroughly investigating similarly heavy events in current detector sensitivity using a diverse choice of priors. Absent such an approach, adopting a flat prior on the binary's redshifted total mass and mass ratio emerges as a reasonable choice, preventing biases in the detector-frame mass posteriors.
更多查看译文
关键词
inferred masses,bayesian priors,quasi-circular,intermediate-mass
AI 理解论文
溯源树
样例
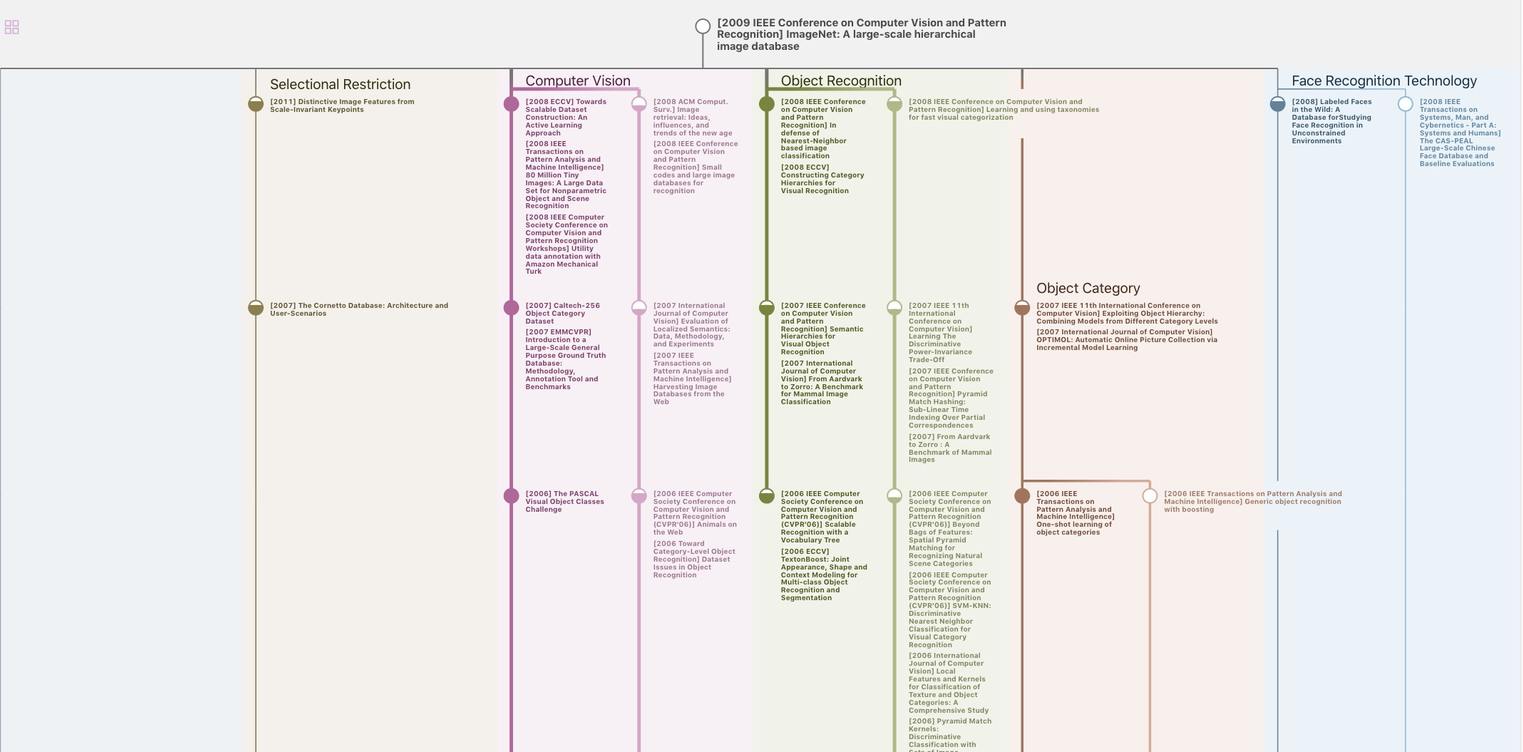
生成溯源树,研究论文发展脉络
Chat Paper
正在生成论文摘要