Predictions and uncertainty estimates of reactor pressure vessel steel embrittlement using Machine learning
MATERIALS & DESIGN(2023)
摘要
An essential aspect of extending safe operation of the world's active nuclear reactors is understanding and predicting the embrittlement that occurs in the steels that make up the Reactor pressure vessel (RPV). In this work we integrate state of the art machine learning methods using ensembles of neural networks with unprecedented data collection and integration to develop a new model for RPV steel embrittlement. The new model has multiple improvements over previous machine learning and hand-tuned efforts, including greater accuracy (e.g., at high-fluence relevant for extending the life of present reactors), wider domain of applicability (e.g., including a wide-range of compositions), uncertainty quantification, and online accessibility for easy use by the community. These improvements provide a model with significant new capabilities, including the ability to easily and accurately explore compositions, flux, and fluence effects on RPV steel embrittlement for the first time. Furthermore, our detailed comparisons show our approach improves on the leading American Society for Testing and Materials (ASTM) E900-15 standard model for RPV embrittlement on every metric we assessed, demonstrating the efficacy of machine learning approaches for this type of highly demanding materials property prediction.
更多查看译文
关键词
Reactor pressure vessel,Embrittlement,Transition temperature shift,Machine learning,Neural network
AI 理解论文
溯源树
样例
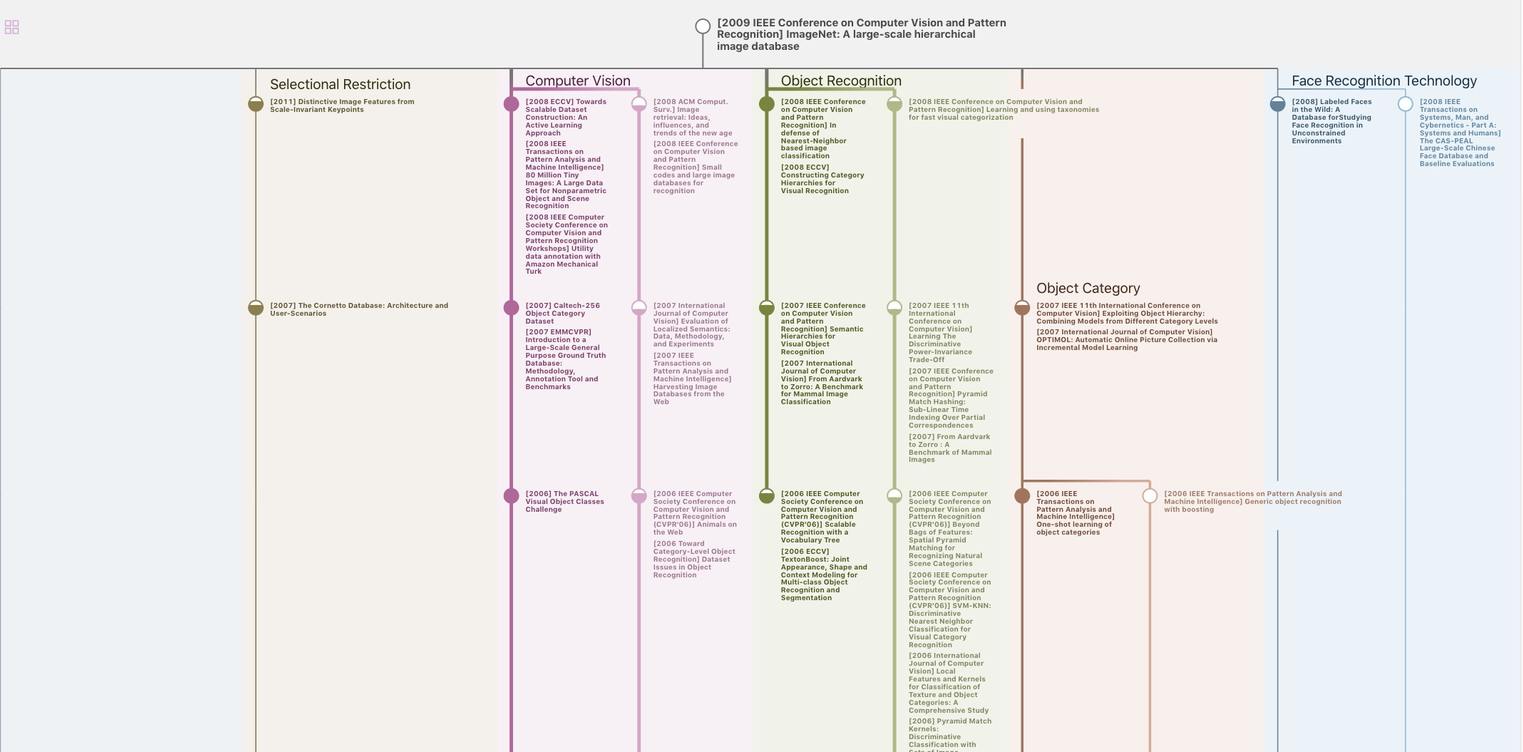
生成溯源树,研究论文发展脉络
Chat Paper
正在生成论文摘要