A Lightweight, Rapid and Efficient Deep Convolutional Network for Chest X-Ray Tuberculosis Detection
ISBI(2023)
摘要
Tuberculosis (TB) is still recognized as one of the leading causes of death worldwide. Recent advances in deep learning (DL) have shown to enhance radiologists' ability to interpret chest X-ray (CXR) images accurately and with fewer errors, leading to a better diagnosis of this disease. However, little work has been done to develop models capable of diagnosing TB that offer good performance while being efficient, fast and computationally inexpensive. In this work, we propose LightTBNet, a novel lightweight, fast and efficient deep convolutional network specially customized to detect TB from CXR images. Using a total of 800 frontal CXR images from two publicly available datasets, our solution yielded an accuracy, F1 and area under the ROC curve (AUC) of 0.906, 0.907 and 0.961, respectively, on an independent test subset. The proposed model demonstrates outstanding performance while delivering a rapid prediction, with minimal computational and memory requirements, making it highly suitable for deployment in handheld devices that can be used in low-resource areas with high TB prevalence. Code publicly available at https://github.com/dani-capellan/LightTBNet.
更多查看译文
关键词
Deep learning,Efficient ML,Deep convolutional neural network,Chest X-ray,Tuberculosis
AI 理解论文
溯源树
样例
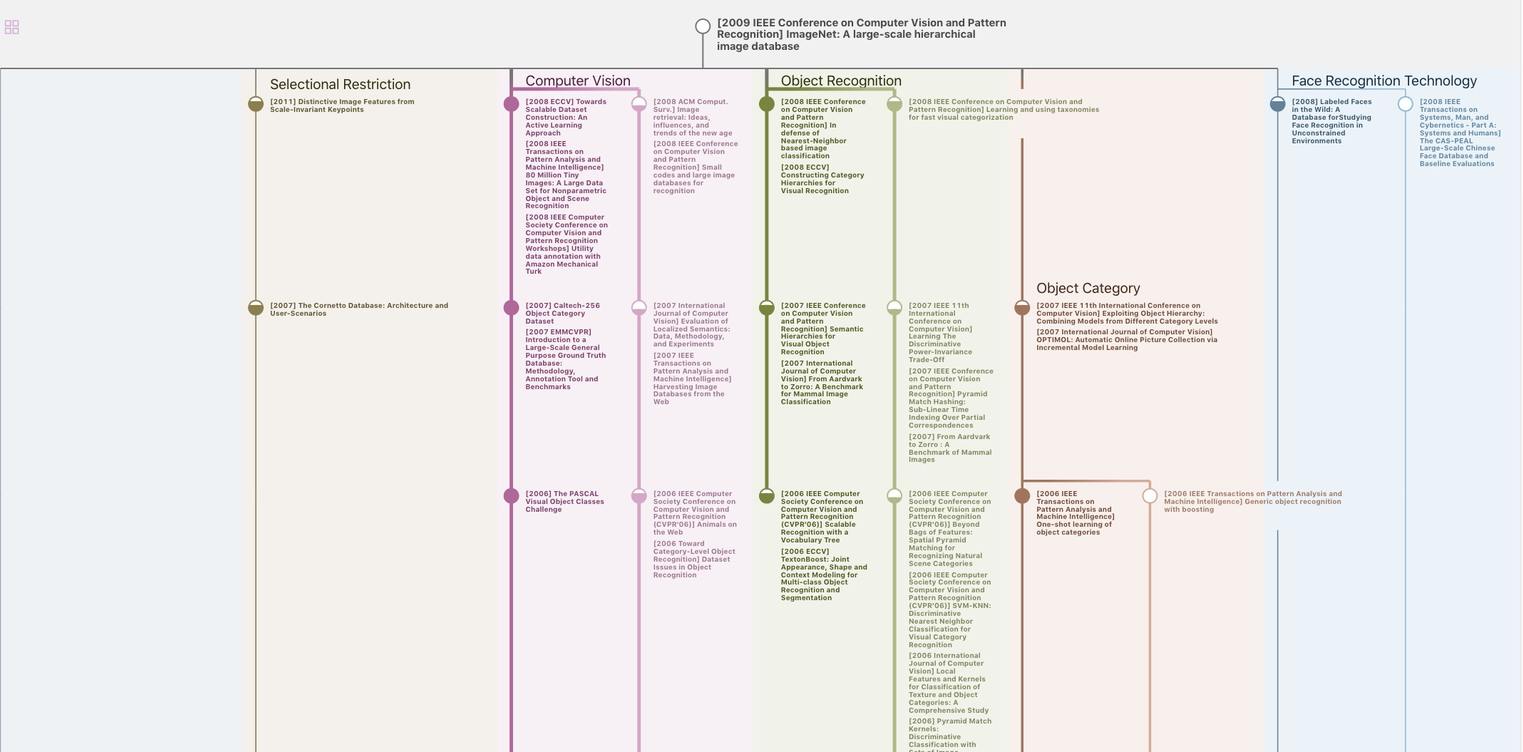
生成溯源树,研究论文发展脉络
Chat Paper
正在生成论文摘要