Differentially Private Synthetic Heavy-tailed Data
arXiv (Cornell University)(2023)
摘要
The U.S. Census Longitudinal Business Database (LBD) product contains employment and payroll information of all U.S. establishments and firms dating back to 1976 and is an invaluable resource for economic research. However, the sensitive information in LBD requires confidentiality measures that the U.S. Census in part addressed by releasing a synthetic version (SynLBD) of the data to protect firms' privacy while ensuring its usability for research activities, but without provable privacy guarantees. In this paper, we propose using the framework of differential privacy (DP) that offers strong provable privacy protection against arbitrary adversaries to generate synthetic heavy-tailed data with a formal privacy guarantee while preserving high levels of utility. We propose using the K-Norm Gradient Mechanism (KNG) with quantile regression for DP synthetic data generation. The proposed methodology offers the flexibility of the well-known exponential mechanism while adding less noise. We propose implementing KNG in a stepwise and sandwich order, such that new quantile estimation relies on previously sampled quantiles, to more efficiently use the privacy-loss budget. Generating synthetic heavy-tailed data with a formal privacy guarantee while preserving high levels of utility is a challenging problem for data curators and researchers. However, we show that the proposed methods can achieve better data utility relative to the original KNG at the same privacy-loss budget through a simulation study and an application to the Synthetic Longitudinal Business Database.
更多查看译文
关键词
data,heavy-tailed
AI 理解论文
溯源树
样例
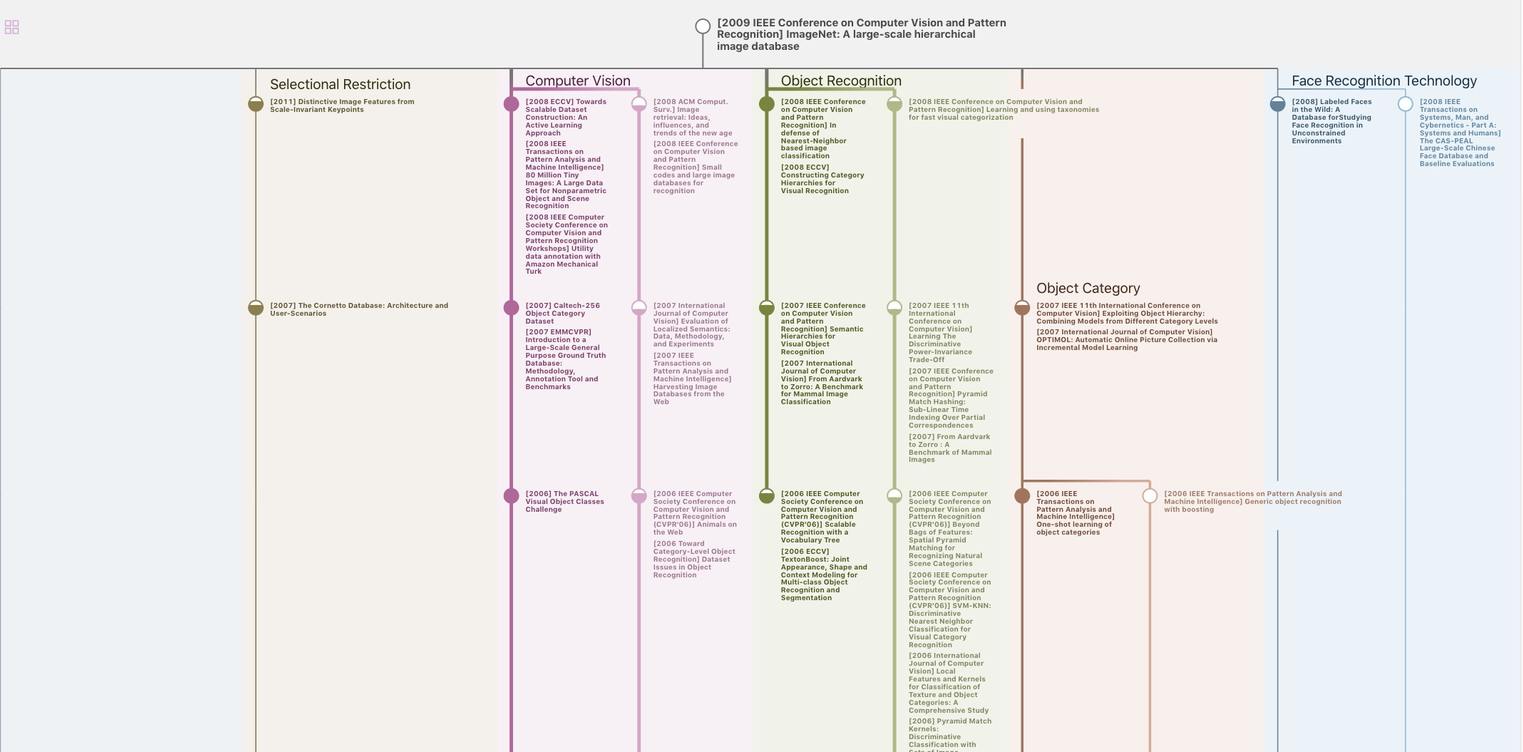
生成溯源树,研究论文发展脉络
Chat Paper
正在生成论文摘要