Learning Vehicle Dynamics From Cropped Image Patches for Robot Navigation in Unpaved Outdoor Terrains
IEEE ROBOTICS AND AUTOMATION LETTERS(2024)
摘要
In the realm of autonomous mobile robots, safe navigation through unpaved outdoor environments remains a challenging task. Due to the high-dimensional nature of sensor data, extracting relevant information becomes a complex problem, which hinders adequate perception and path planning. Previous works have shown promising performances in extracting global features from full-sized images. However, they often face challenges in capturing essential local information. In this letter, we propose Crop-LSTM, which iteratively takes cropped image patches around the current robot's position and predicts the future position, orientation, and bumpiness. Our method performs local feature extraction by paying attention to corresponding image patches along the predicted robot trajectory in the 2D image plane. This enables more accurate predictions of the robot's future trajectory. With our wheeled mobile robot platform Raicart, we demonstrated the effectiveness of Crop-LSTM for point-goal navigation in an unpaved outdoor environment. Our method enabled safe and robust navigation using RGBD images in challenging unpaved outdoor terrains.
更多查看译文
关键词
Navigation,Robots,Vehicle dynamics,Mobile robots,Collision avoidance,Robot sensing systems,Predictive models,Autonomous vehicle navigation,deep learning methods
AI 理解论文
溯源树
样例
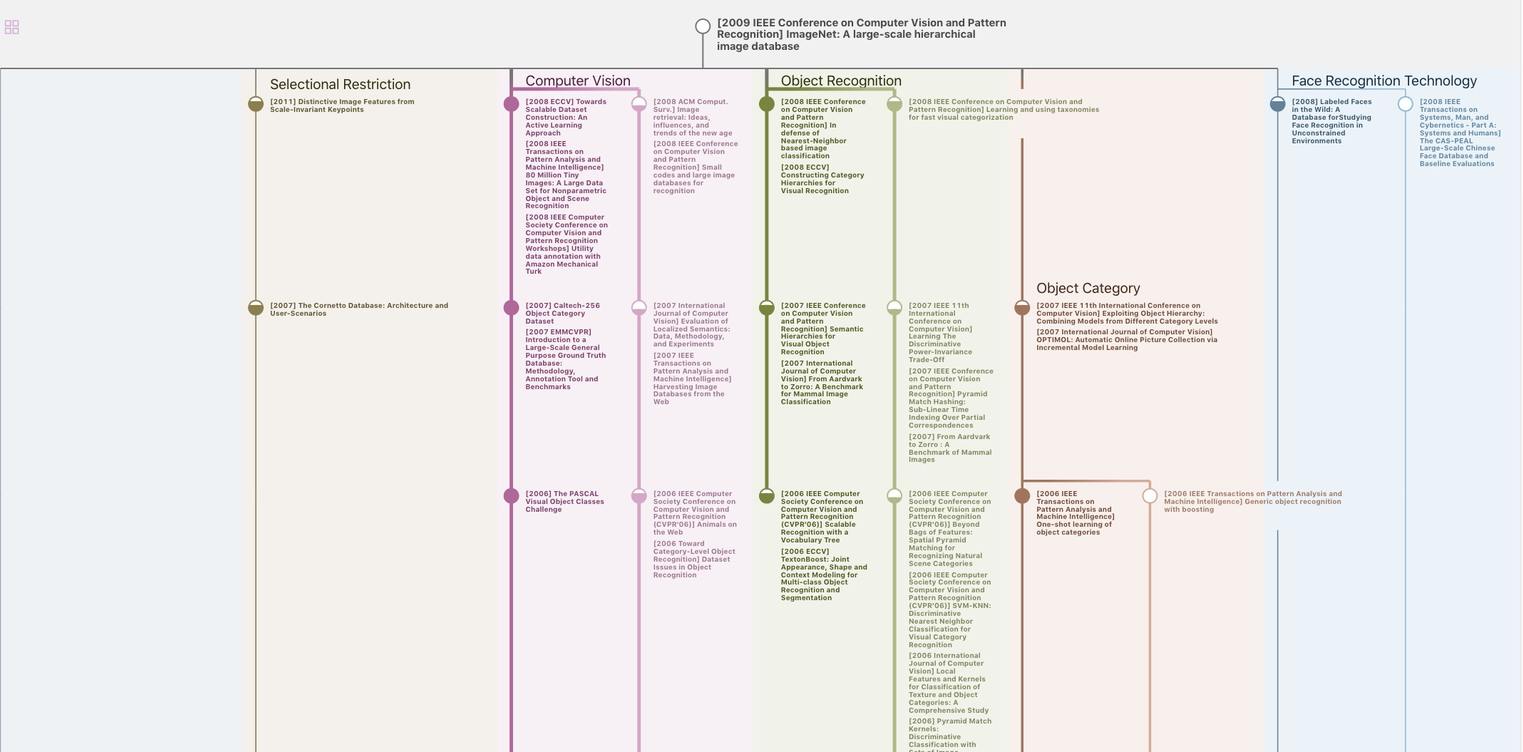
生成溯源树,研究论文发展脉络
Chat Paper
正在生成论文摘要