Learning to Estimate Without Bias
IEEE TRANSACTIONS ON SIGNAL PROCESSING(2023)
摘要
The Gauss-Markov theorem states that the weighted least squares estimator is a linear minimum variance unbiased estimation (MVUE) in linear models. In this paper, we take a first step towards extending this result to non-linear settings via deep learning with bias constraints. The classical approach to designing non-linear MVUEs is through maximum likelihood estimation (MLE) which often involves computationally challenging optimizations. On the other hand, deep learning methods allow for non-linear estimators with fixed computational complexity. Learning based estimators perform optimally on average with respect to their training set but may suffer from significant bias in other parameters. To avoid this, we propose to add a simple bias constraint to the loss function, resulting in an estimator we refer to as Bias Constrained Estimator (BCE). We prove that this yields asymptotic MVUEs that behave similarly to the classical MLEs and asymptotically attain the Cramer Rao bound. We demonstrate the advantages of our approach in the context of signal to noise ratio estimation as well as covariance estimation. A second motivation to BCE is in applications where multiple estimates of the same unknown are averaged for improved performance. Examples include distributed sensor networks and data augmentation in test-time. In such applications, we show that BCE leads to asymptotically consistent estimators.
更多查看译文
关键词
bias,learning,estimate
AI 理解论文
溯源树
样例
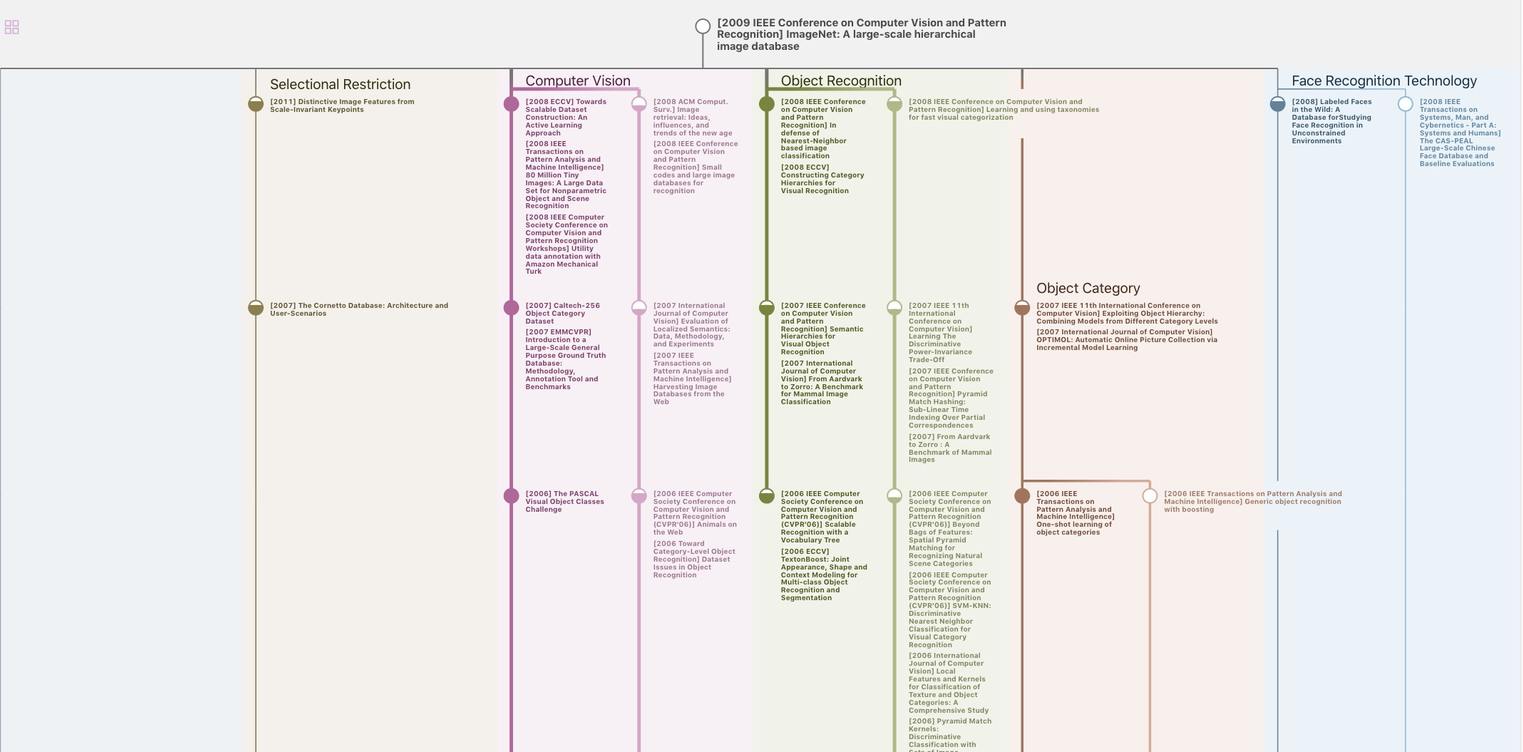
生成溯源树,研究论文发展脉络
Chat Paper
正在生成论文摘要