3DCNN predicting brain age using diffusion tensor imaging
Medical & biological engineering & computing(2023)
摘要
Neuroimaging-based brain age prediction using deep learning is gaining popularity. However, few studies have attempted to leverage diffusion tensor imaging (DTI) to predict brain age. In this study, we proposed a 3D convolutional neural network model (3DCNN) and trained it on fractional anisotropy (FA) data from six publicly available datasets ( n = 2406, age = 17–60) to estimate brain age. Implementing a two-loop nested cross-validation scheme with a tenfold cross-validation procedure, we achieved a robust prediction performance of a mean absolute error (MAE) of 2.785 and a correlation coefficient of 0.932. We also employed Grad-Cam++ to visualize the salient features of the proposed model. We identified a few highly salient fiber tracts, including the genu of corpus callosum and the left cerebellar peduncle, among others that play a pivotal role in our model. In sum, our model reliably predicted brain age and provided novel insight into age-related changes in brains’ axonal structure. Graphical Abstract
更多查看译文
关键词
MRI, Diffusion tensor imaging, Deep learing, Brain age, Interpretability
AI 理解论文
溯源树
样例
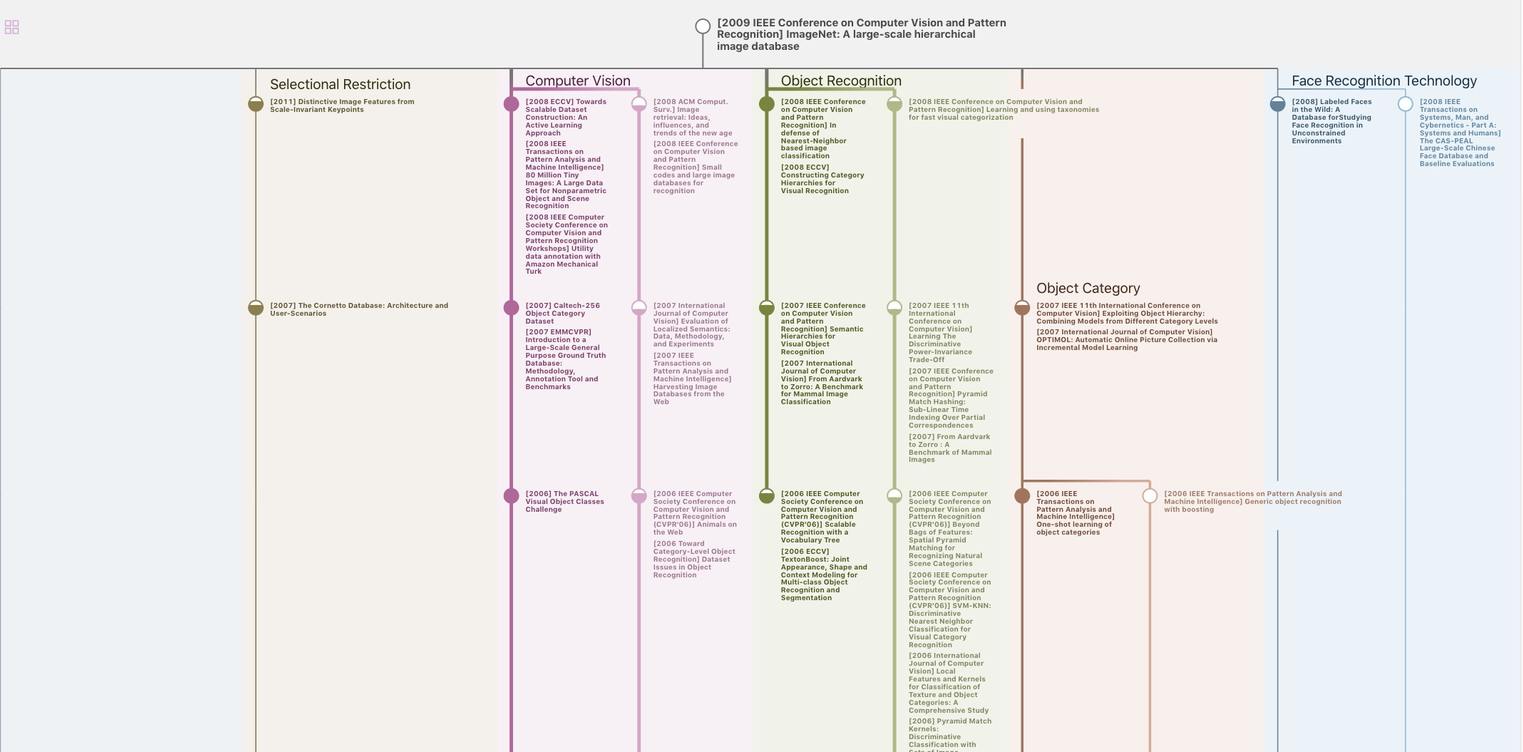
生成溯源树,研究论文发展脉络
Chat Paper
正在生成论文摘要