A hybrid machine learning framework for forecasting house price
Expert Systems with Applications(2023)
摘要
House price prediction is one of the most important factors affecting national real estate policies. However, developing an accurate housing price prediction model is a significant challenge for the real estate market. This study presents a framework of house price prediction models that address this issue by improving forecasting performance, explicitly demonstrating the novelty in the Hybrid Bayesian Optimization (HBO) models combined with Stacking (HBOS), Bagging (HBOB), and Transformer (HBOT) techniques. These hybrid models employ Bayesian Optimization for hyperparameter tuning, leading to superior prediction accuracy and stability. Additionally, the proposed framework can assess a statistical and accurate assessment of the predictive performance of house price forecasting models in different scenarios. Furthermore, we constructed a multi-source dataset containing 1,898,175 transactions of the Hong Kong real estate market covering a period from January 2, 1996, to May 13, 2021. This dataset, another major contribution to the field, enables comprehensive model testing and could be a valuable resource for future research. Then, the proposed hybrid models are compared with 18 benchmark models. Thirteen evaluation metrics are used to evaluate the predictive performance, while the non-parametric testing, including Friedman, Iman–Davenport, and Nemenyi post-hoc tests methods, are adopted to assess the significance of differences in the predictive performance of each model. The experimental results show that the HBOS models are superior to the other benchmark models for application in the house price prediction problem. The HBOS-CatBoost model showed superior performance in terms of RMSE compared to both the HBOB-XGBoost and HBOT-ConvLSTM models, with relative RMSE reductions of 5.11% and 25.56%, respectively. The main contributions of this work are the creation of a rich multi-source dataset, the proposal of novel hybrid models for improved house price prediction, and a comprehensive performance evaluation framework. These findings offer a significant step forward in the housing price prediction field.
更多查看译文
关键词
Machine learning,House prices,Stacking,Bagging,Transformer
AI 理解论文
溯源树
样例
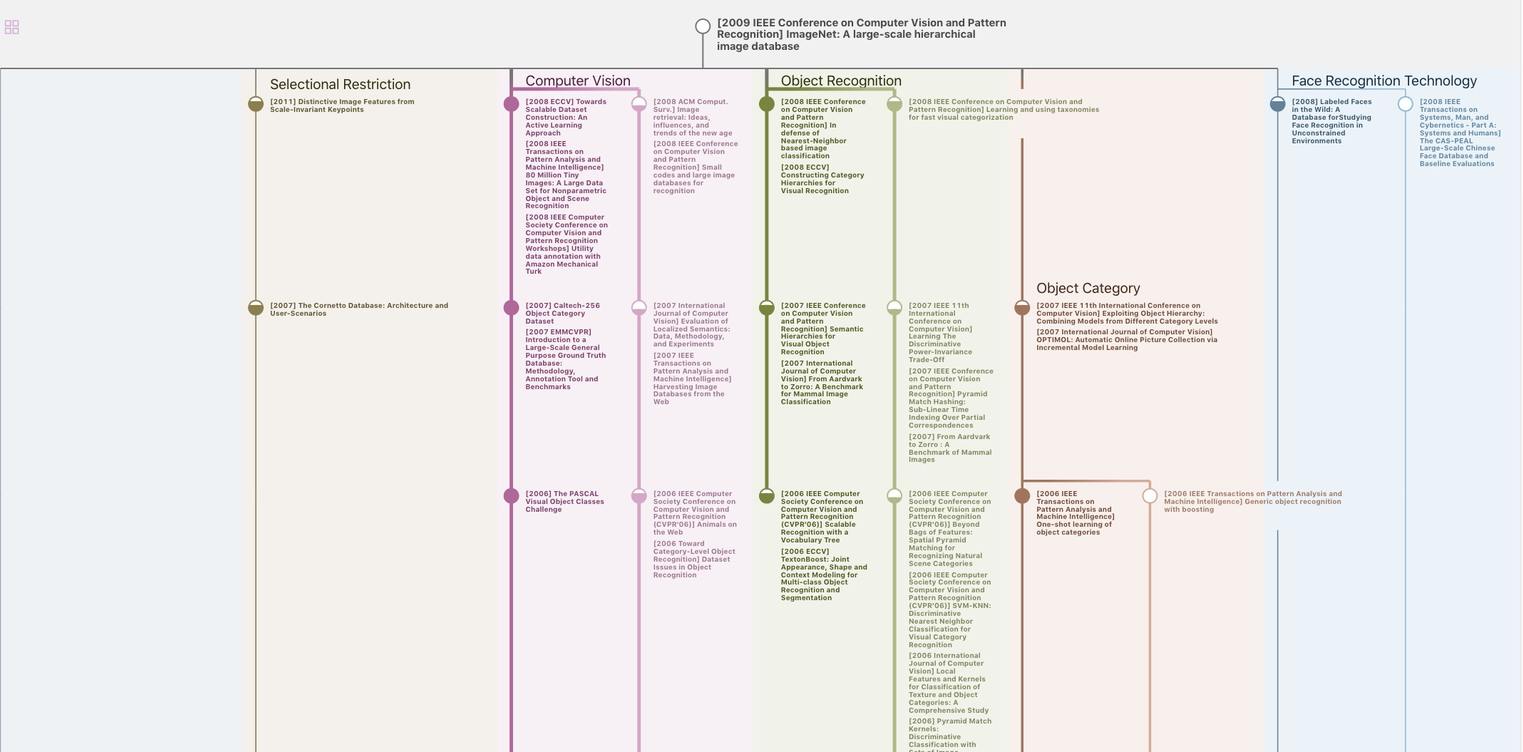
生成溯源树,研究论文发展脉络
Chat Paper
正在生成论文摘要