Identification of diversions in spent PWR fuel assemblies by PDET signatures using Artificial Neural Networks (ANNs)
ANNALS OF NUCLEAR ENERGY(2023)
摘要
Spent nuclear fuel represents the majority of materials placed under nuclear safeguards today and it requires to be inspected and verified regularly to promptly detect any illegal diversion. Research is ongoing both on the development of non-destructive assay instruments and methods for data analysis in order to enhance the verification accuracy and reduce the inspection time. In this paper, two models based on Artificial Neural Networks (ANNs) are studied to process measurements from the Partial Defect Tester (PDET) in spent fuel assemblies of Pressurized Water Reactors (PWRs), and thus to identify at different levels of detail whether nuclear fuel has been replaced with dummy pins or not. The first model provides an estimation of the percentage of replaced fuel pins within the inspected fuel assembly, while the second model determines the exact configuration of the replaced fuel pins. The two models are trained and tested using a dataset of Monte-Carlo simulated PDET responses for intact spent PWR fuel assemblies and a variety of hypothetical diversion scenarios. The first model classifies fuel assemblies according to the percentage of diverted fuel with a high accuracy (96.5%). The second model reconstructs the correct configuration for 57.5% of the fuel assemblies available in the dataset and still retrieves meaningful information of the diversion pattern in many of the misclassified cases.
更多查看译文
关键词
Artificial Neural Networks, Spent nuclear fuel, Nuclear safeguards, Machine learning, Radiation detection
AI 理解论文
溯源树
样例
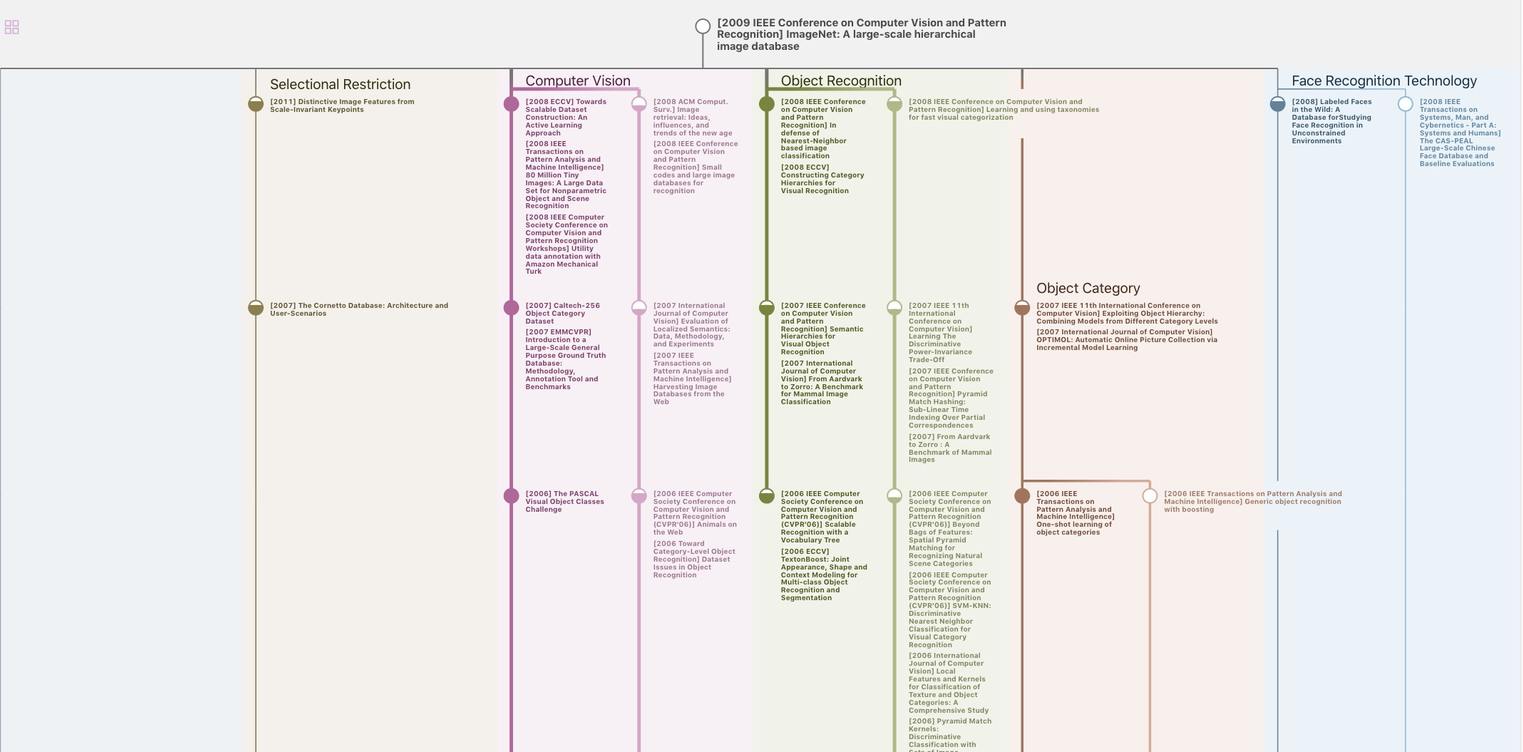
生成溯源树,研究论文发展脉络
Chat Paper
正在生成论文摘要