Self-supervised contrastive speaker verification with nearest neighbor positive instances.
Pattern Recognit. Lett.(2023)
摘要
Self-supervised contrastive learning (SSCL) has achieved a great success in speaker verification (SV). All recent works treat within-utterance speaker embeddings (SE) to be positive instances, encouraging them to be as close as possible. However, positive instances from the same utterance have similar channel and related semantic information, which are difficult to distinguish from the speaker features. Moreover, these positive instances can only provide limited variances in a certain speaker. To tackle the above problems, we propose to use nearest neighbor (NN) positive instances for training, which are selected from a dynamic queue. The NN positive instances can provide different channel and semantic information, increasing the variances in a certain speaker. Our proposed method are validated through comprehensive experiments on VoxCeleb and CNCeleb1 datasets, demonstrating its effectiveness in improving both SSCL and fine-tuning results. Additionally, our SSCL model outperforms supervised training model in cross-dataset testing due to the use of massive unlabeled data.
更多查看译文
关键词
Self-supervised contrastive learning,Speaker verification,Nearest neighbor positive instances
AI 理解论文
溯源树
样例
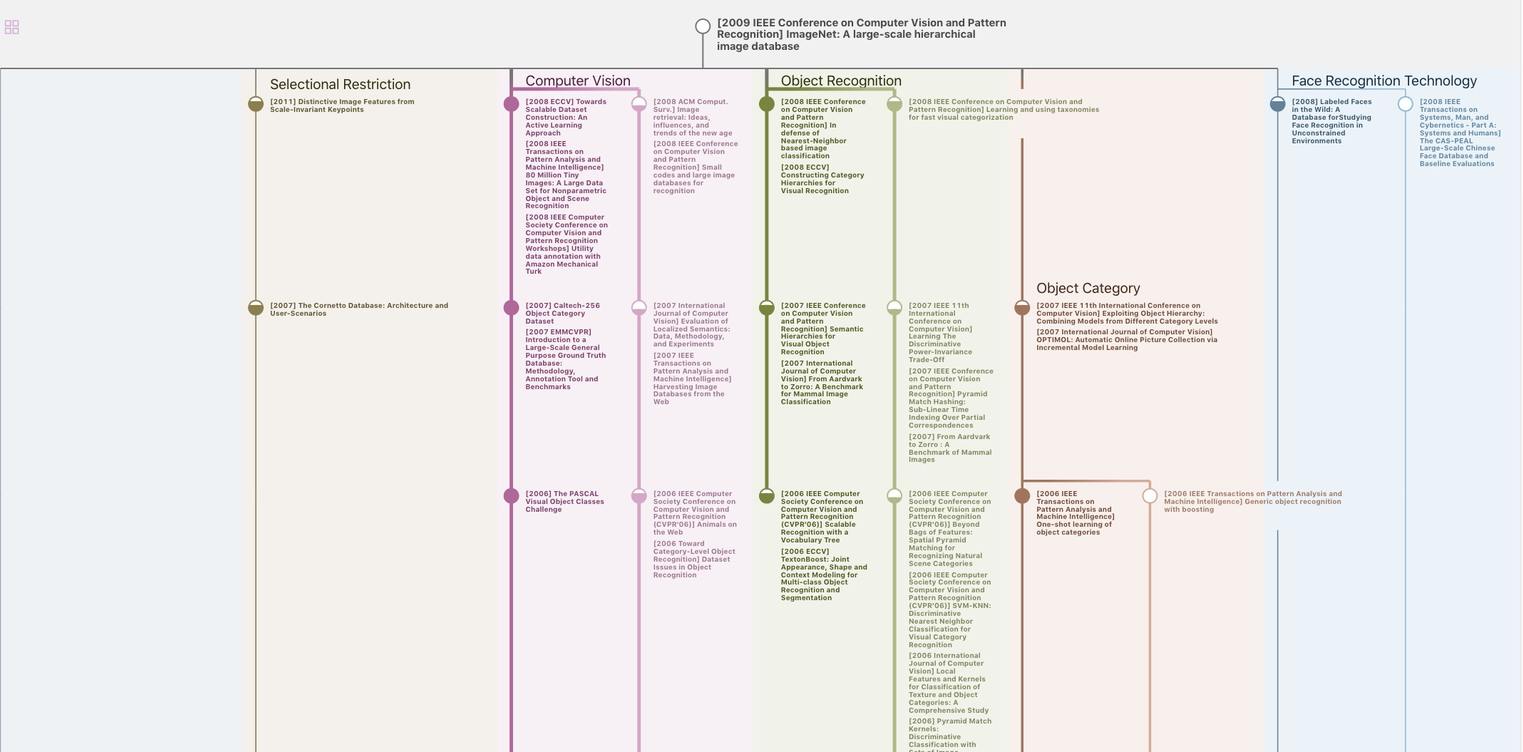
生成溯源树,研究论文发展脉络
Chat Paper
正在生成论文摘要