A coevolutionary algorithm assisted by two archives for constrained multi-objective optimization problems
SWARM AND EVOLUTIONARY COMPUTATION(2023)
摘要
Coevolutionary algorithms have demonstrated high performance on many constrained multi-objective prob-lems. However, on some problems with fraudulent constraints or small feasible regions, they may fail to converge to the Pareto front (PF) or even fail to find a feasible solution. To search for feasible solutions robustly for various problems and obtain solutions approaching the PF as possible, this paper proposes a coevolutionary algorithm assisted by two archives. To be specific, a population for minimizing constraint violation and a population for optimizing objectives without constraints coevolve to find a feasible solution first. Then, the feasible solutions are improved by the population with constrained dominance principle and an archive consisting of inversely-updated infeasible solutions. In addition to searching in the feasible regions found so far, the unconstrained population continues searching the objectives without constraints, expecting that new feasible regions can be spotted in the promising regions. To mitigate the issues that small feasible regions may be missed by the unconstrained population, a diversity archive is updated in a larger objective space than the unconstrained population to enhance exploration. In the experiments, the proposed method is compared with 11 state-of-the-art algorithms on 67 problems to demonstrate its effectiveness. The results show that the proposed method is very robust in finding feasible solutions and obtains better or competitive performance on most problems.
更多查看译文
关键词
coevolutionary algorithm,optimization,multi-objective
AI 理解论文
溯源树
样例
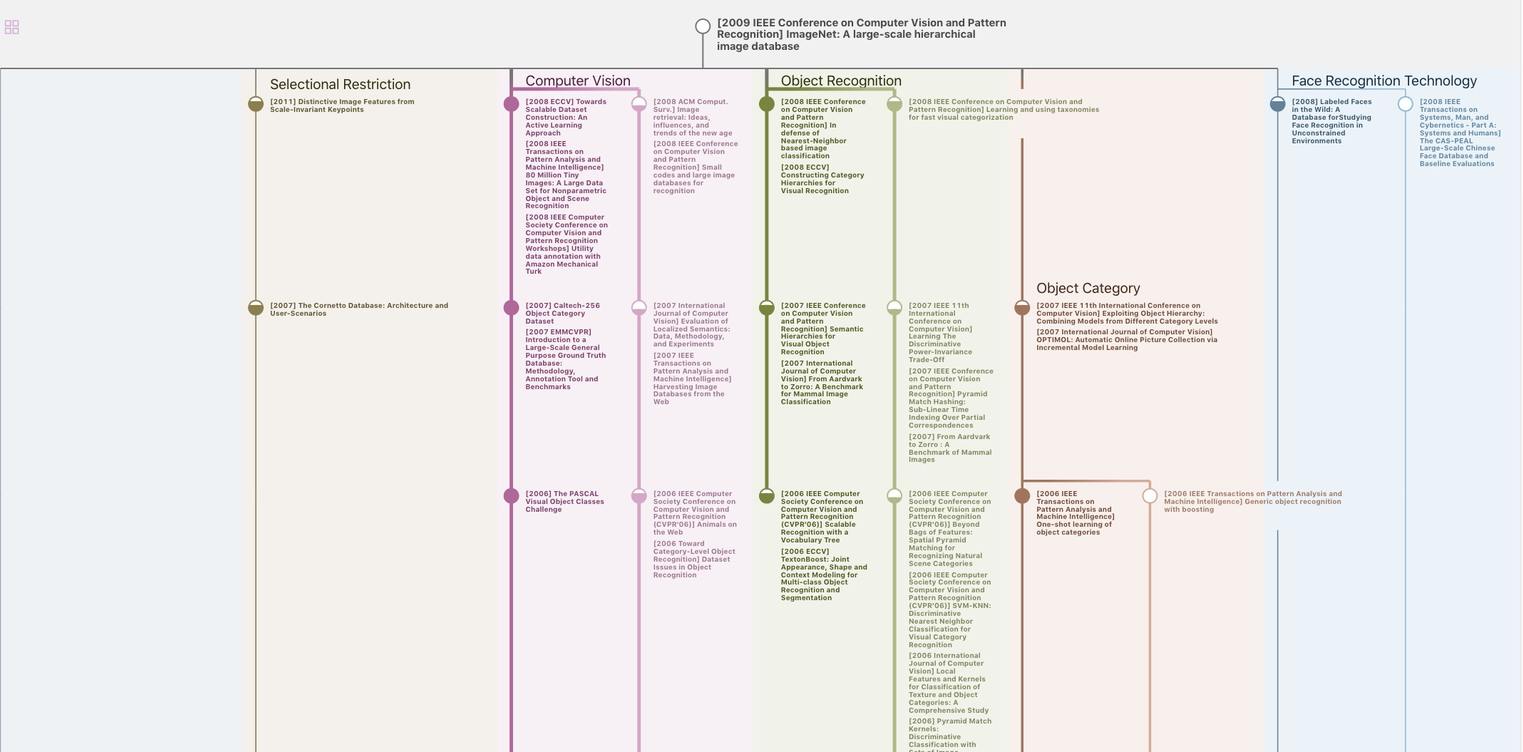
生成溯源树,研究论文发展脉络
Chat Paper
正在生成论文摘要