Breast ultrasound image classification and physiological assessment based on GoogLeNet
JOURNAL OF RADIATION RESEARCH AND APPLIED SCIENCES(2023)
摘要
Background: Medical ultrasound image classification based on convolutional neural network is the mainstream breast cancer classification model, but its limited perceptual ability limits its ability to obtain global information.Methodology: A total of 880 breast ultrasound images were collected from 700 patients including 103 normal images, 467 malignant tumor images and 210 benign tumor images. In this paper, the diagnosis of breast ul-trasound images was realized by constructing the CNN model of GoogLeNet. Firstly, the breast images were preprocessed based on the TV model. After that, the CNN model was trained and a more accurate model with a wider range of application was obtained based on improved Inception. Then we extract image features of different sizes; Then, feature classification was completed in different model classifiers to realize benign and malignant detection of breast cancer. Meanwhile, comparative analysis was performed to verify the excellence of the GoogLeNet model.Results: The training time for classification was effectively reduced, and the classification accuracy rate was improved, reaching 96.37% combined with transformer learning. The loss value is down to 0.3492. Then, the classification accuracy of different structural models is discussed in the two models. The results show that GoogLeNet has great advantages in the detection of breast cancer ultrasound images. The influence of migration on experimental results is further discussed. Finally, combined with transfer learning, the three models were tested separately. The results show that transfer learning can improve system performance.Conclusion: Based on the TV model and the GoogLeNet model, this paper designs a method of breast cancer detection and classification based on the combination of TV and GoogLeNet model and transfer learning. Ex-periments show that this method can repair part of the texture damaged by markers in ultrasonic images, effectively extract the features of thyroid nodule, and accurately judge whether the breast is diseased, which greatly improves the diagnostic efficiency of doctors.
更多查看译文
关键词
Breast cancer diagnosis,Transfer learning,Deeping learning,Image classification,GoogLeNet
AI 理解论文
溯源树
样例
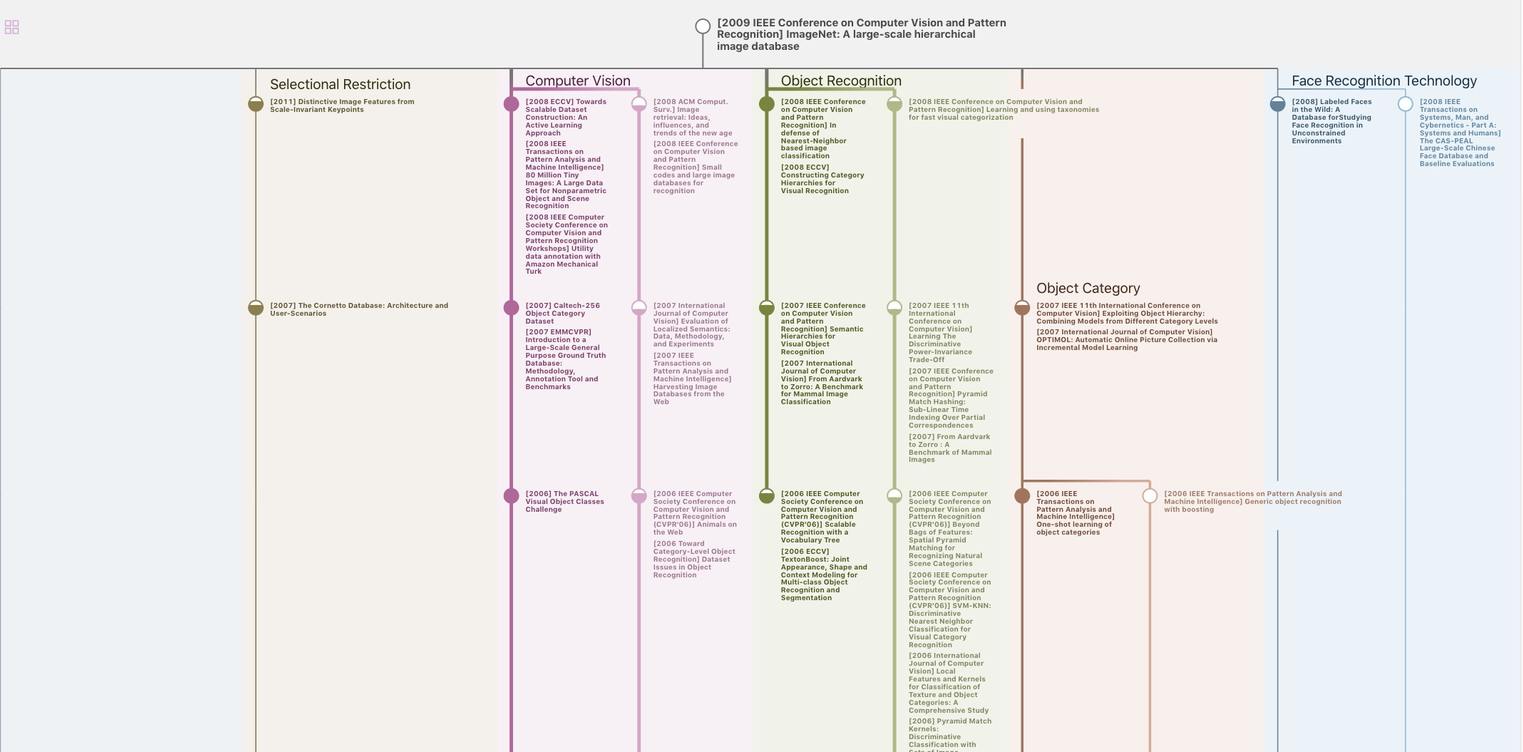
生成溯源树,研究论文发展脉络
Chat Paper
正在生成论文摘要