MFCINet: multi-level feature and context information fusion network for RGB-D salient object detection
JOURNAL OF SUPERCOMPUTING(2024)
摘要
Recently, RGB-D salient object detection (SOD) has aroused widespread research interest. Existing methods tend to treat equally features at different levels and lead to inadequate interaction with cross-level features. Furthermore, many methods rely on the stacking of convolution layers or the use of dilated convolutions to increase the receptive field to extract high-level semantic features. However, these approaches may not effectively obtain context information, resulting in the loss of semantic information. In this paper, we propose a novel multi-level feature and context information fusion network (MFCINet) for RGB-D SOD, which mainly includes a detail enhancement fusion module (DEFM), semantic enhancement fusion module (SEFM), and multi-scale receptive field enhancement module (MREM). Concretely, we first design a detail enhancement fusion module (DEFM) and a semantic enhancement fusion module (SEFM) by introducing a combination of dual attention mechanisms to better fuse the rich details in low-level features and the rich semantic information in high-level features, respectively. Subsequently, a multi-scale receptive field enhancement module (MREM) is deployed to obtain the rich context semantic information in the network with the help of the parallel operation of convolution cores and skip connections, which are input into the subsequent dense connection pyramid decoder for SOD. Experimental results on five common datasets show that our model outperforms the 17 state-of-the-art (SOTA) methods.
更多查看译文
关键词
Context semantic information,Cross-level features,Multi-level fusion,Salient object detection
AI 理解论文
溯源树
样例
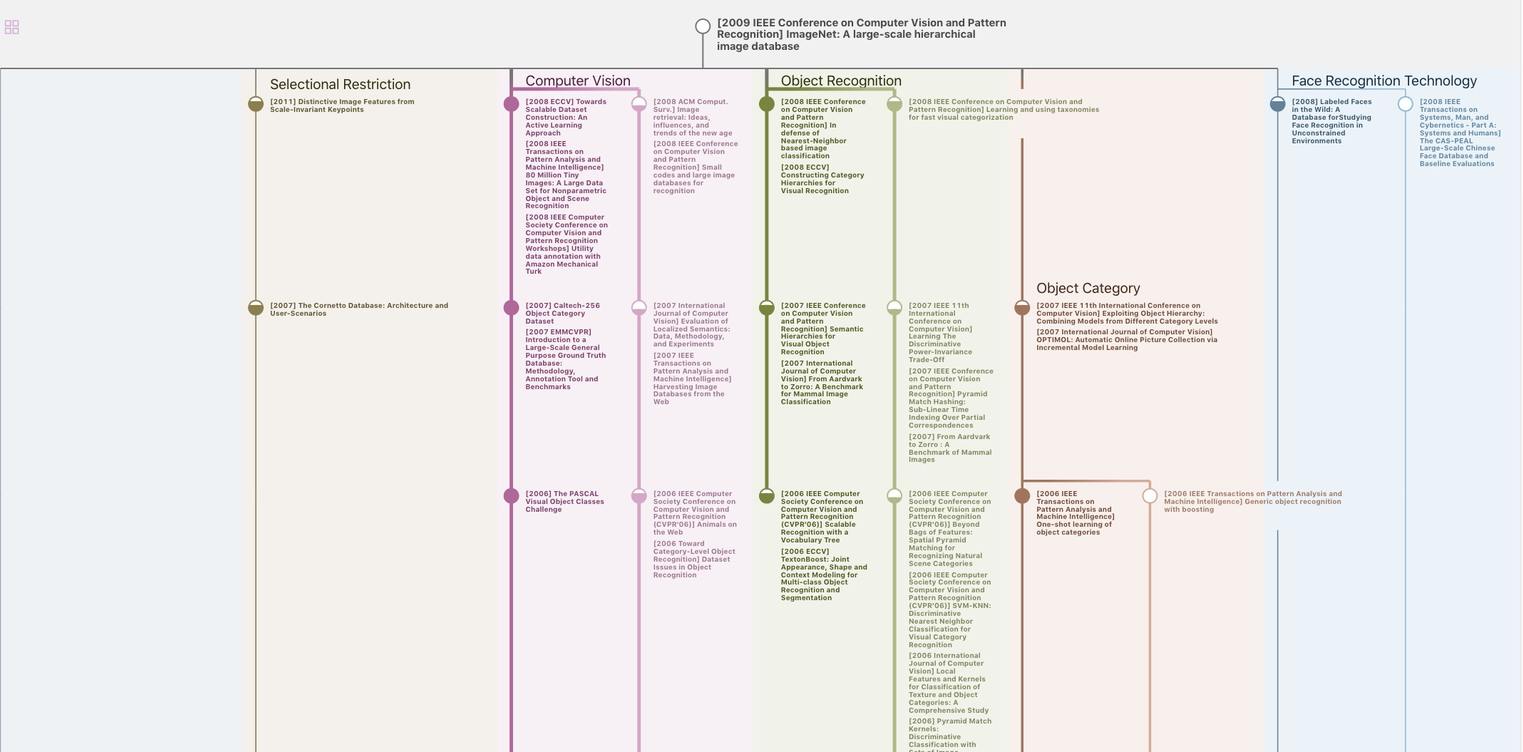
生成溯源树,研究论文发展脉络
Chat Paper
正在生成论文摘要