wAdvMTD: A Mitigation to White-box Adversarial Examples Using Heterogeneous Models and Moving Target Defense
2023 3RD ASIA-PACIFIC CONFERENCE ON COMMUNICATIONS TECHNOLOGY AND COMPUTER SCIENCE, ACCTCS(2023)
摘要
In recent years, the adversarial example has become a non-trivial threat to the deep learning model. It harms the availability and integrity of deep learning as the inconspicuous perturbation is hard to be distinguished by a human observer. Among the mitigation strategies, those schemes using moving target defense as the methodology to defend adversarial examples proclaims a satisfactory performance in the black-box context. As for protecting deep learning models from white-box adversaries, however, only scheduling a model from the candidate set randomly to handle these stronger adversaries seems insufficient. Furthermore, the mitigation is vulnerable if the scheduling process is exposed to the adversary. In this paper, we proposed a white-box adversarial examples mitigation mechanism called wAdvMTD. It can automatically diversify the model structure of deep neural networks to make the attack vector to a certain model fails on the rest of its heterogeneous models. We make a proof-of-concept by deploying this mechanism to ResNet. Compared with the methodology that only schedules one of the pre-built models, using a set of structure-diversified models can reach a higher accuracy even with the constraint that this mechanism is completely exposed to the adversary.
更多查看译文
关键词
Moving Target Defense,Adversarial Examples,Model Diversification
AI 理解论文
溯源树
样例
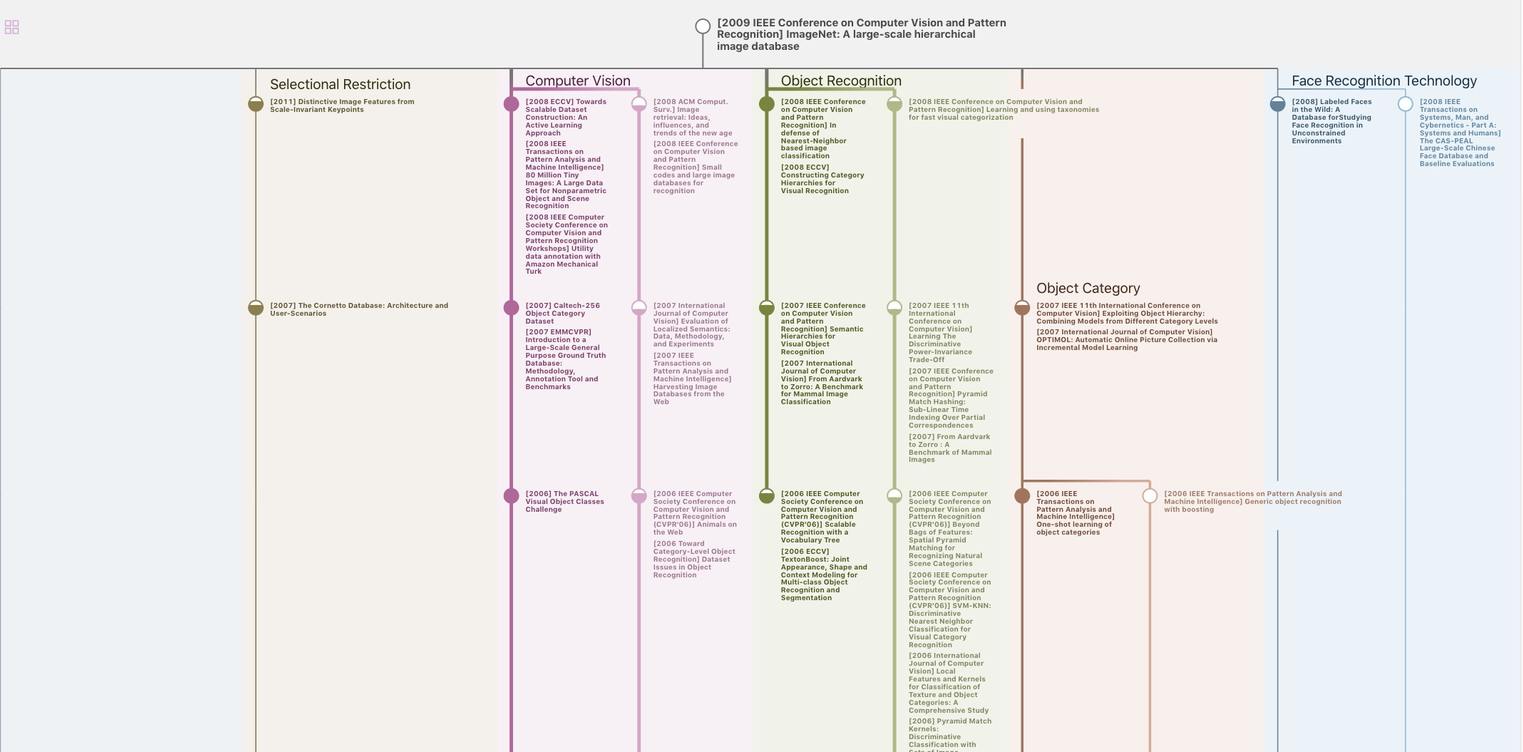
生成溯源树,研究论文发展脉络
Chat Paper
正在生成论文摘要